SC-PNC: Semantic Communication-Empowered Physical-layer Network Coding
IEEE Transactions on Cognitive Communications and Networking(2024)
摘要
This paper puts forth the first framework for semantic communication (SC)-empowered physical-layer network coding (PNC), referred to as SC-PNC. Although conventional bit-oriented PNC can enhance the throughput of wireless relay networks by turning mutual wireless interference into useful network-coded information, it faces two primary problems that limit its application in practice. First, bit-oriented PNC decoding is susceptible to the relative phase offsets among signals received from different nodes; in particular, some “bad” relative phase offsets could lead to significant performance degradation. Second, the scheduling design of bit-oriented PNC transmissions is limited by the bitwise operation. To address these issues, this paper designs SC-PNC, which leverages semantic communication to bypass the need for bit-perfect message recovery at the destination. First, we employ a two-way relay network (TWRN) to demonstrate how SC-PNC effectively mitigates the detrimental effects of “bad” relative phase offsets. Then, we explore a triangular relay network (TriRN) to show how we can take advantage of semantic communication to redesign the scheduling of PNC transmissions. Specifically, an SC-PNC TriRN architecture is designed, wherein each node receives information from the other two nodes in only two time slots. Taking image delivery as an example, experimental results reveal that SC-PNC consistently achieves high and stable image reconstruction quality under different channel conditions and relative phase offsets, outperforming the conventional bit-oriented counterparts. Moreover, the new two-slot SC-PNC TriRN architecture is effective in extracting semantically accurate information from images, showcasing its potential as a low-latency solution for semantic information exchange.
更多查看译文
关键词
Semantic communication,physical-layer network coding (PNC),deep learning,PNC scheduling
AI 理解论文
溯源树
样例
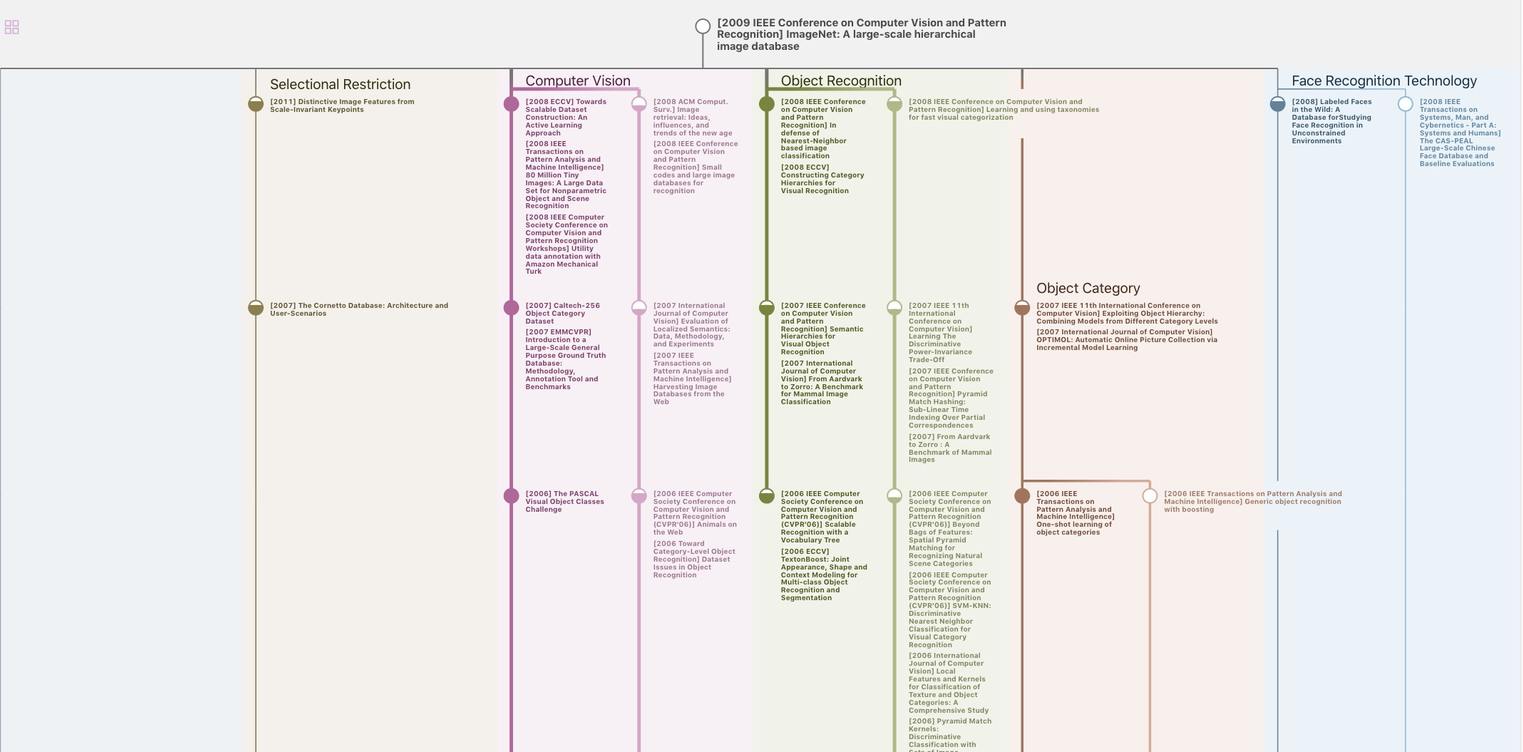
生成溯源树,研究论文发展脉络
Chat Paper
正在生成论文摘要