Treated Wastewater Assessment to Optimize Agricultural Water Reuse in Al-Qatif Region Saudi Arabia Using Hybrid Machine Learning Techniques
crossref(2024)
摘要
In the era of mounting water scarcity, particularly in arid regions, the agricultural sector is striving to optimize the water resource management practices. Treated wastewater (TWW) has emerged as a viable alternative to freshwater for irrigation, offering a sustainable solution to water conservation. However, ensuring the safety and quality of TWW is paramount to safeguard for both crop health and environmental integrity. Electrical conductivity (EC), a critical factor in assessing TWW suitability for irrigation, can significantly impact crop yields and soil health since it is directly linked to the concentration of dissolved salts and ions. Therefore, this study delves into the development of advanced predictive models for EC assessment by employing supervised machine learning (ML) techniques like the Support Vector Regression (SVR), Adaptive Network-based Fuzzy Inference System (ANFIS), and Multiple Linear Regression (MLR). The TWW samples collected from Al-Qatif area, Saudi Arabia were assessed in the lab through a sensor-based real-time monitoring system integrated with Internet of Things (IoT) network and a multiprobe device. The key water quality parameters assessed involved, temperature (T), pH, Oxidation Reduction Potential (ORP), Resistivity (RES), Total Dissolved Solids (TDS), Salinity and Turbidity which served as the foundation for these models, given their profound influence on and their anticipated impact on EC levels. Following feature selection, two distinct model combinations were identified for the SVR, ANFIS and MLR models. Furthermore, to evaluate prediction performance, four statistical criteria, including coefficient of determination (R²), correlation coefficient (R), mean square error (MSE), and root mean square error (RMSE), were employed. Overall, the comparative performance results proved that ANFIS-M2 indicated the strongest predictive capability with the R² value of 0.99572 and 0.75145 and the RMSE values of 0.00965 and 0.01134, respectively in both calibration and verification stages.
更多查看译文
AI 理解论文
溯源树
样例
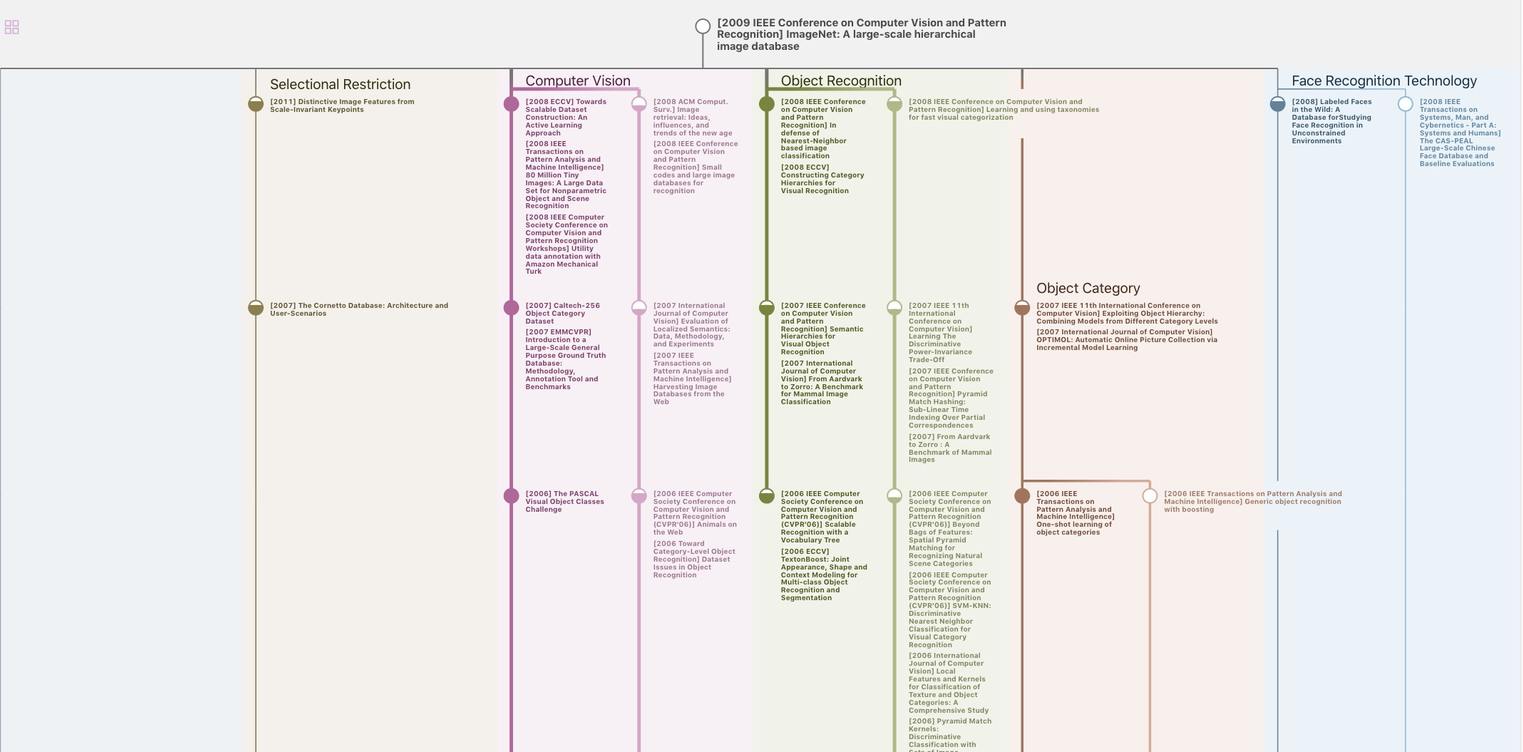
生成溯源树,研究论文发展脉络
Chat Paper
正在生成论文摘要