Machine learning power system emulation for rapid screening of multi-sector policies
crossref(2024)
摘要
Linking integrated water-energy simulation with multi-objective search algorithms provides a practical design tool for interdependent river basins and power systems. However, this approach is typically limited by the computational resources required to complete the many thousands of simulations to discover efficient solutions. We introduce an artificial neural network-based power system emulator to enable optimized design of large-scale detailed multi-sector water-energy systems. The proposed framework links an integrated power system emulator and river system simulator to an AI-driven multi-objective search design process. We compare optimized designs using both the power system emulator and simulator to check the emulators’ computational speed and accuracy. The framework is applied to the Sudanese power system and its link to the Eastern Nile river basin, to investigate how optimized operational strategies of the Grand Ethiopian Renaissance Dam (GERD) could affect Sudan’s resource systems. Results are similar for the power system emulator and simulator, showing the emulator helps to significantly reduce the computational cost of using sophisticated multi-sector policy design approaches.
更多查看译文
AI 理解论文
溯源树
样例
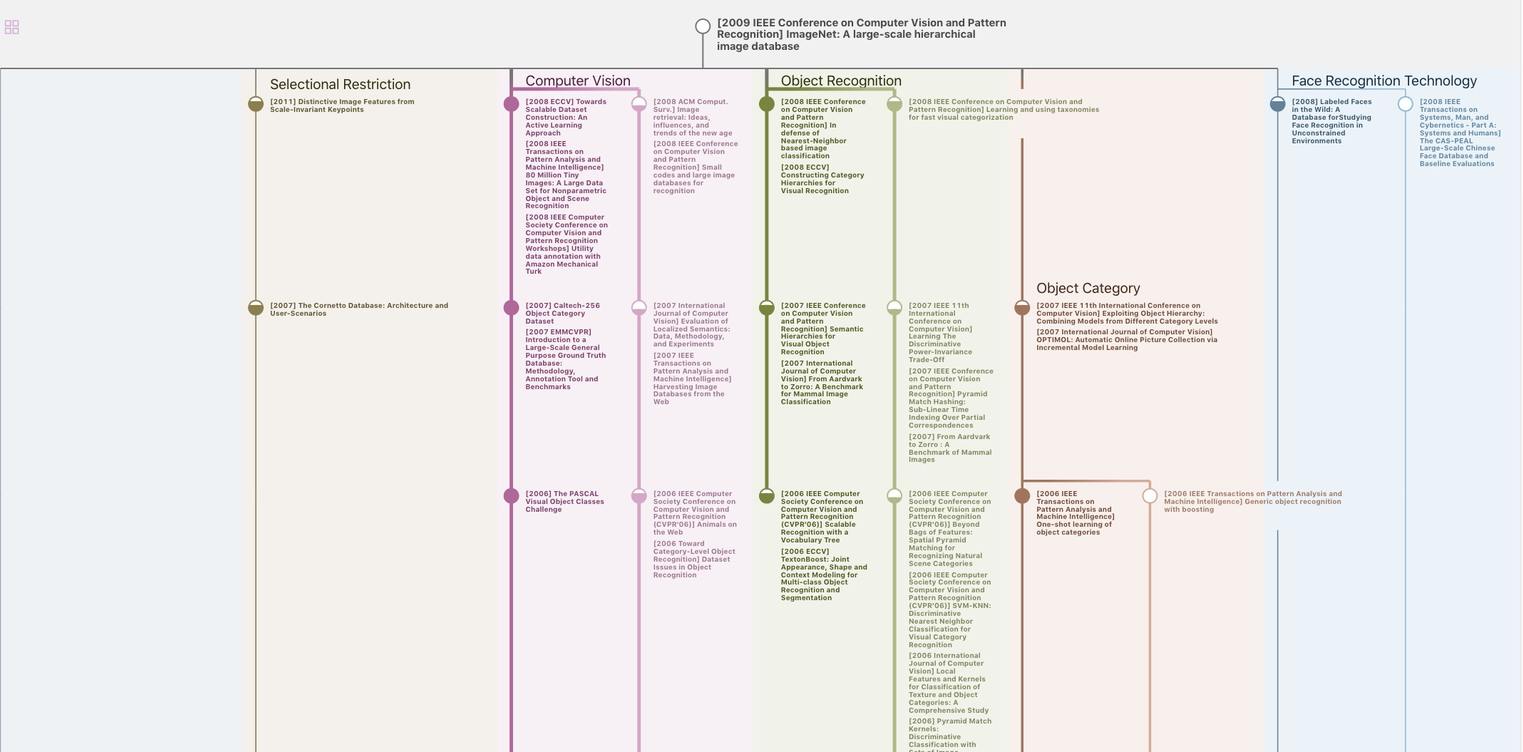
生成溯源树,研究论文发展脉络
Chat Paper
正在生成论文摘要