Machine learning models reveal distinct disease subgroups and improve diagnostic and prognostic accuracy for individuals with pathogenic SCN8A gain-of-function variants.
Biology open(2024)
摘要
Distinguishing clinical subgroups for patients suffering with diseases characterized by a wide phenotypic spectrum is essential for developing precision therapies. Patients with gain-of-function (GOF) variants in the SCN8A gene exhibit substantial clinical heterogeneity, viewed historically as a linear spectrum ranging from mild to severe. To test for hidden clinical subgroups, we applied two machine learning algorithms to analyze a dataset of patient features collected by the International SCN8A Patient Registry. We utilized two research methodologies: a supervised approach that incorporated feature severity cutoffs based on clinical conventions, and an unsupervised approach employing an entirely data-driven strategy. Both approaches found statistical support for three distinct subgroups and were validated by correlation analyses utilizing external variables. However, distinguishing features of the three subgroups within each approach were not concordant, suggesting a more complex phenotypic landscape. The unsupervised approach yielded strong support for a model involving three partially-ordered subgroups rather than a linear spectrum. Application of these machine-learning approaches may lead to improved prognosis and clinical management of individuals with SCN8A GOF variants and provide insights into the underlying mechanisms of the disease.
更多查看译文
关键词
pediatric epilepsy,rare disease,genetic epilepsy,clinical phenotype,unsupervised learning,patient registry
AI 理解论文
溯源树
样例
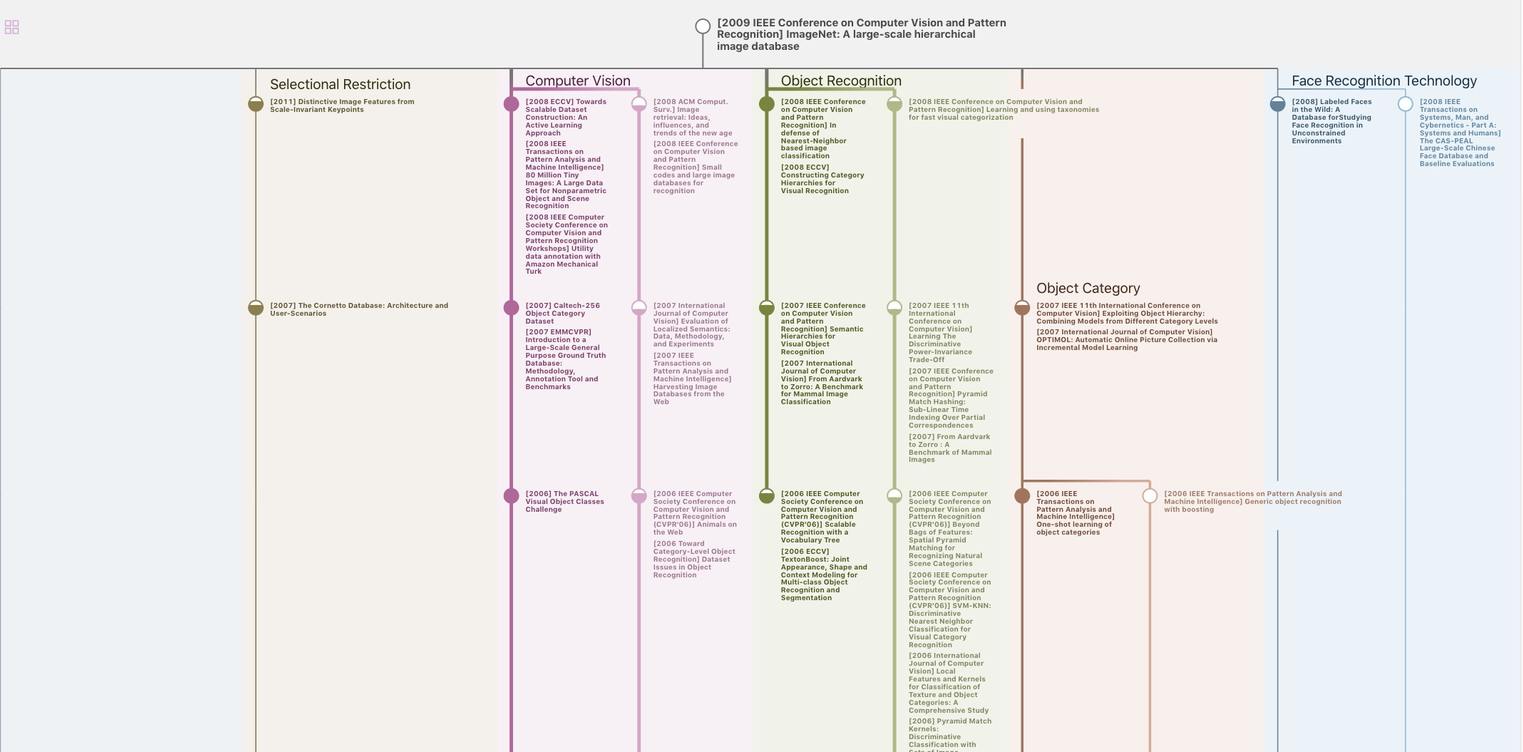
生成溯源树,研究论文发展脉络
Chat Paper
正在生成论文摘要