PocketFlow is a data-and-knowledge-driven structure-based molecular generative model
Nature Machine Intelligence(2024)
摘要
Deep learning-based molecular generation has extensive applications in many fields, particularly drug discovery. However, the majority of current deep generative models are ligand-based and do not consider chemical knowledge in the molecular generation process, often resulting in a relatively low success rate. We herein propose a structure-based molecular generative framework with chemical knowledge explicitly considered (named PocketFlow), which generates novel ligand molecules inside protein binding pockets. In various computational evaluations, PocketFlow showed state-of-the-art performance, with generated molecules being 100% chemically valid and highly drug-like. Ablation experiments prove the critical role of chemical knowledge in ensuring the validity and drug-likeness of the generated molecules. We applied PocketFlow to two new target proteins that are related to epigenetic regulation, HAT1 and YTHDC1, and successfully obtained wet-lab validated bioactive compounds. The binding modes of the active compounds with target proteins are close to those predicted by molecular docking and further confirmed by the X-ray crystal structure. All the results suggest that PocketFlow is a useful deep generative model, capable of generating innovative bioactive molecules from scratch given a protein binding pocket.
更多查看译文
AI 理解论文
溯源树
样例
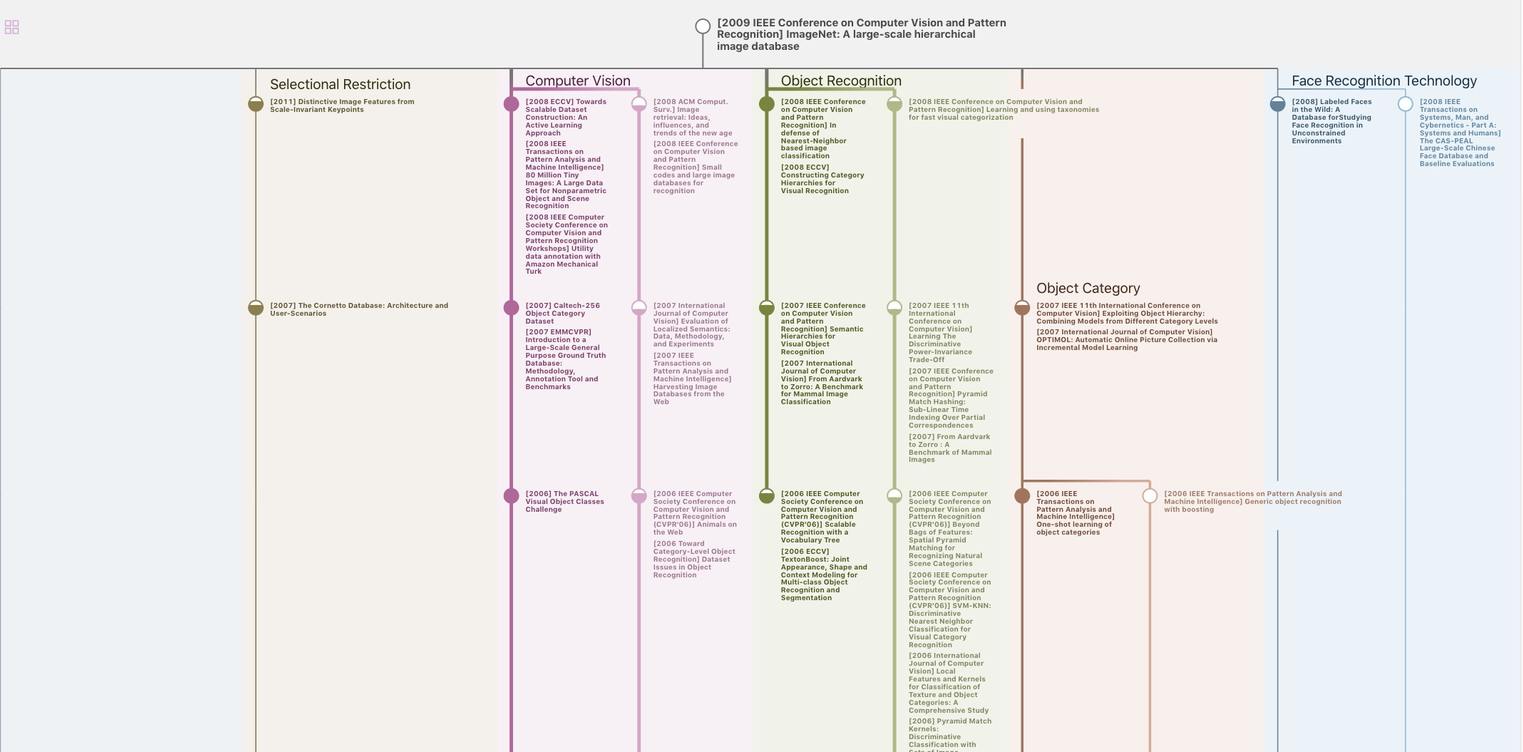
生成溯源树,研究论文发展脉络
Chat Paper
正在生成论文摘要