Advances of Deep Learning in Protein Science: A Comprehensive Survey
arXiv (Cornell University)(2024)
Key words
Proteins,Deep Learning,Support Vector Machines
AI Read Science
Must-Reading Tree
Example
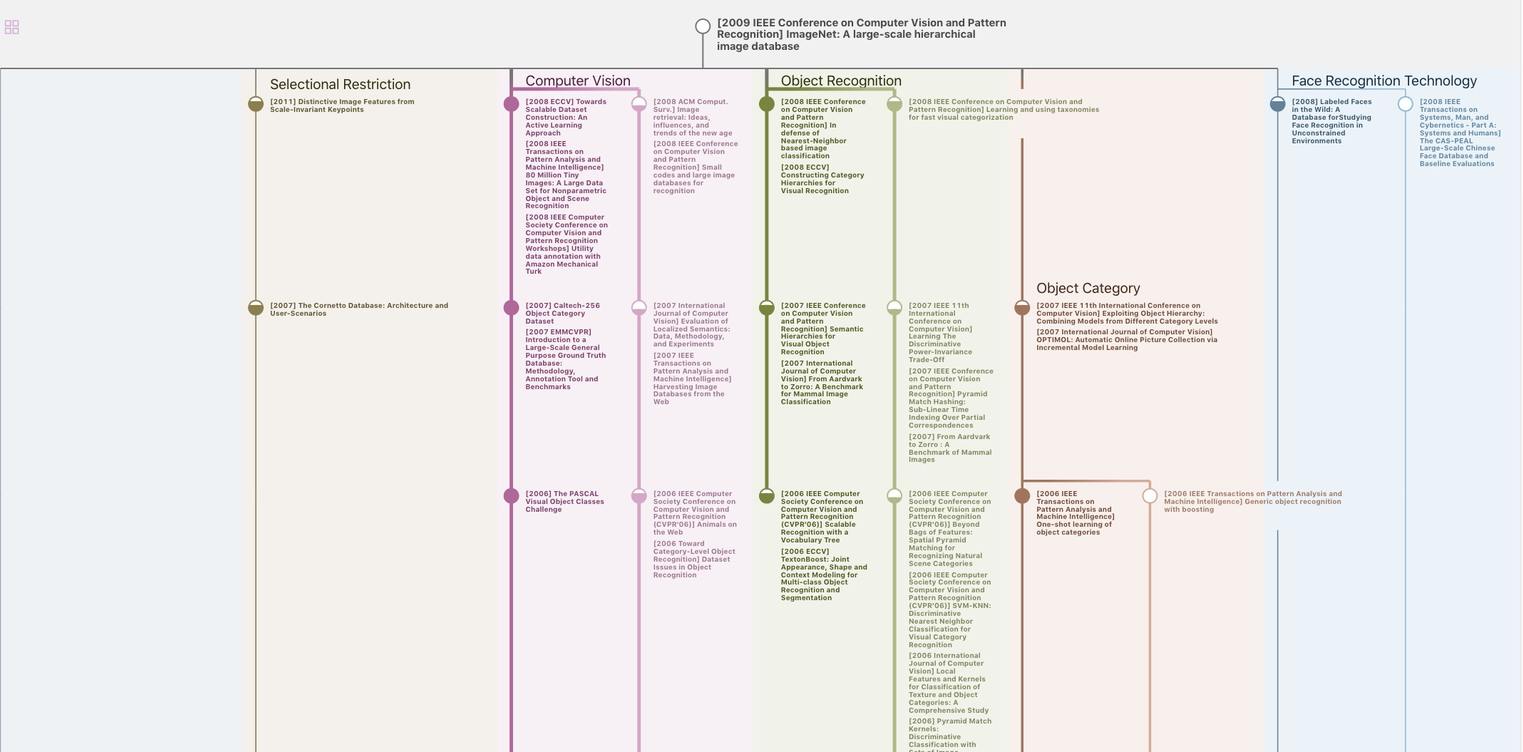
Generate MRT to find the research sequence of this paper
Chat Paper
Summary is being generated by the instructions you defined