MamMIL: Multiple Instance Learning for Whole Slide Images with State Space Models
arxiv(2024)
摘要
Recently, pathological diagnosis, the gold standard for cancer diagnosis, has
achieved superior performance by combining the Transformer with the multiple
instance learning (MIL) framework using whole slide images (WSIs). However, the
giga-pixel nature of WSIs poses a great challenge for the quadratic-complexity
self-attention mechanism in Transformer to be applied in MIL. Existing studies
usually use linear attention to improve computing efficiency but inevitably
bring performance bottlenecks. To tackle this challenge, we propose a MamMIL
framework for WSI classification by cooperating the selective structured state
space model (i.e., Mamba) with MIL for the first time, enabling the modeling of
instance dependencies while maintaining linear complexity. Specifically, to
solve the problem that Mamba can only conduct unidirectional one-dimensional
(1D) sequence modeling, we innovatively introduce a bidirectional state space
model and a 2D context-aware block to enable MamMIL to learn the bidirectional
instance dependencies with 2D spatial relationships. Experiments on two
datasets show that MamMIL can achieve advanced classification performance with
smaller memory footprints than the state-of-the-art MIL frameworks based on the
Transformer. The code will be open-sourced if accepted.
更多查看译文
AI 理解论文
溯源树
样例
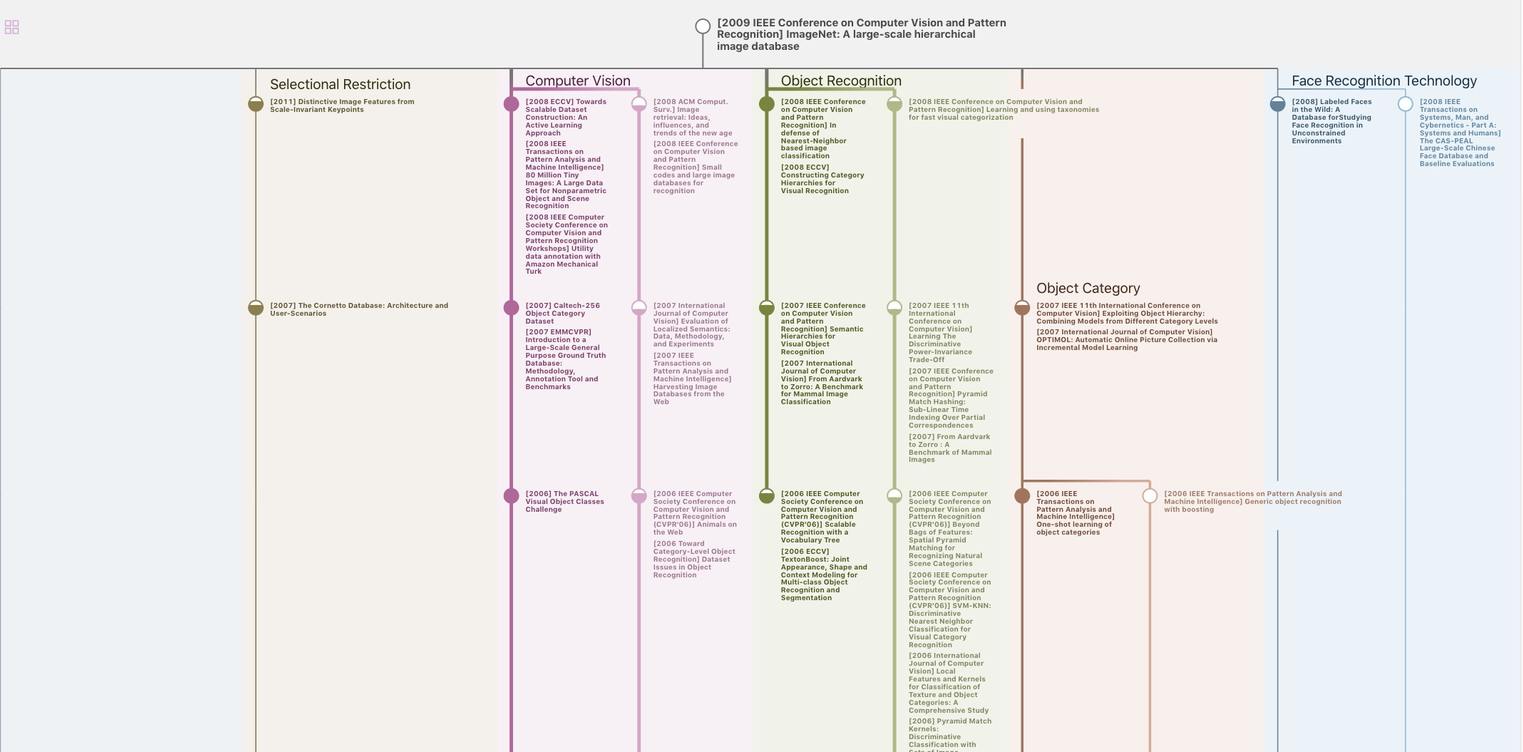
生成溯源树,研究论文发展脉络
Chat Paper
正在生成论文摘要