Large Language Multimodal Models for 5-Year Chronic Disease Cohort Prediction Using EHR Data
arxiv(2024)
摘要
Chronic diseases such as diabetes are the leading causes of morbidity and
mortality worldwide. Numerous research studies have been attempted with various
deep learning models in diagnosis. However, most previous studies had certain
limitations, including using publicly available datasets (e.g. MIMIC), and
imbalanced data. In this study, we collected five-year electronic health
records (EHRs) from the Taiwan hospital database, including 1,420,596 clinical
notes, 387,392 laboratory test results, and more than 1,505 laboratory test
items, focusing on research pre-training large language models. We proposed a
novel Large Language Multimodal Models (LLMMs) framework incorporating
multimodal data from clinical notes and laboratory test results for the
prediction of chronic disease risk. Our method combined a text embedding
encoder and multi-head attention layer to learn laboratory test values,
utilizing a deep neural network (DNN) module to merge blood features with
chronic disease semantics into a latent space. In our experiments, we observe
that clinicalBERT and PubMed-BERT, when combined with attention fusion, can
achieve an accuracy of 73
prediction. By transforming laboratory test values into textual descriptions
and employing the Flan T-5 model, we achieved a 76
(AUROC), demonstrating the effectiveness of leveraging numerical text data for
training and inference in language models. This approach significantly improves
the accuracy of early-stage diabetes prediction.
更多查看译文
AI 理解论文
溯源树
样例
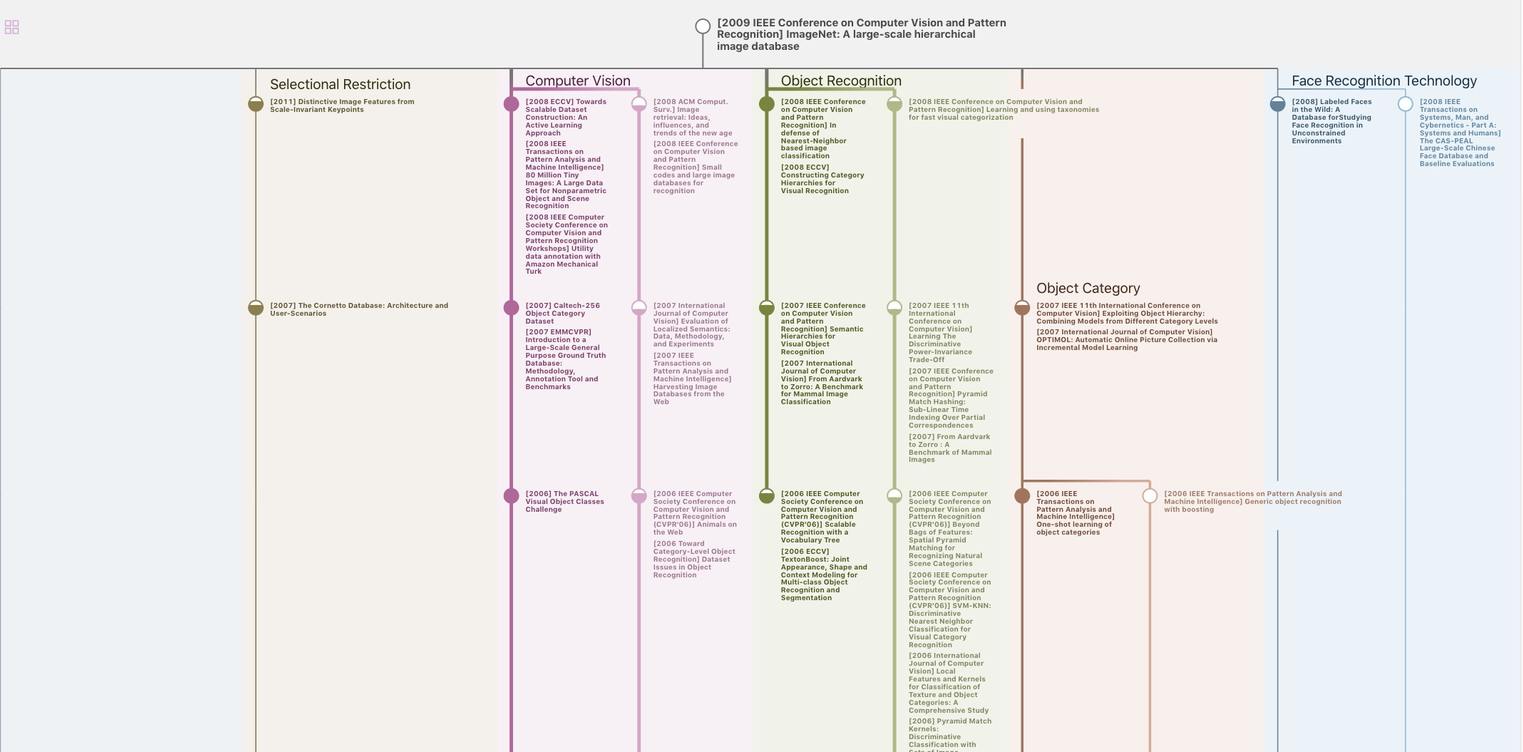
生成溯源树,研究论文发展脉络
Chat Paper
正在生成论文摘要