A Progressively-Passing-then-Disentangling Approach to Recipe Recommendation
IEEE Transactions on Multimedia(2024)
摘要
The increasing popularity of online food blogs and food ordering services has made personalized recipe recommendation a vital aspect of our emotional well-being. However, existing solutions, mainly based on graph neural networks, still face significant challenges, such as (a) focusing on exploiting the user-recipe interactions while neglecting other crucial pairwise and high-order relationships, and (b) failing to explicitly distinguish the distinct factors, e.g., hedonic and healthy, that influence recipe selection. To address these issues, we propose a progressively-passing-then-disentangling approach named P2D. Our approach utilizes a three-stage progressive message-passing mechanism for better representation learning. Specifically, we incorporate the extra pairwise relationships between recipes and nutrients, ingredients, and visual contents to create fine-grained and multimodal recipe representations. We next refine these representations via message passing between high-order recipe relationships to learn people's shared food preferences. Based on them, we could derive comprehensive user representations, which are subsequently transformed into disentangled forms that correspond to various decision factors through contrastive and mutual information regularization. Experimental results demonstrate both the superiority and the rationality of our method: (a) P2D outperforms the state-of-the-art recipe recommendation methods by a large margin under various metrics, (b) ablation studies confirm the positive impact of each of its components, and (c) our visualization analysis empirically supports the advantage of explicitly disentangling decision factors.
更多查看译文
关键词
Recipe recommendation,hypergraph neural network,disentangled representation learning
AI 理解论文
溯源树
样例
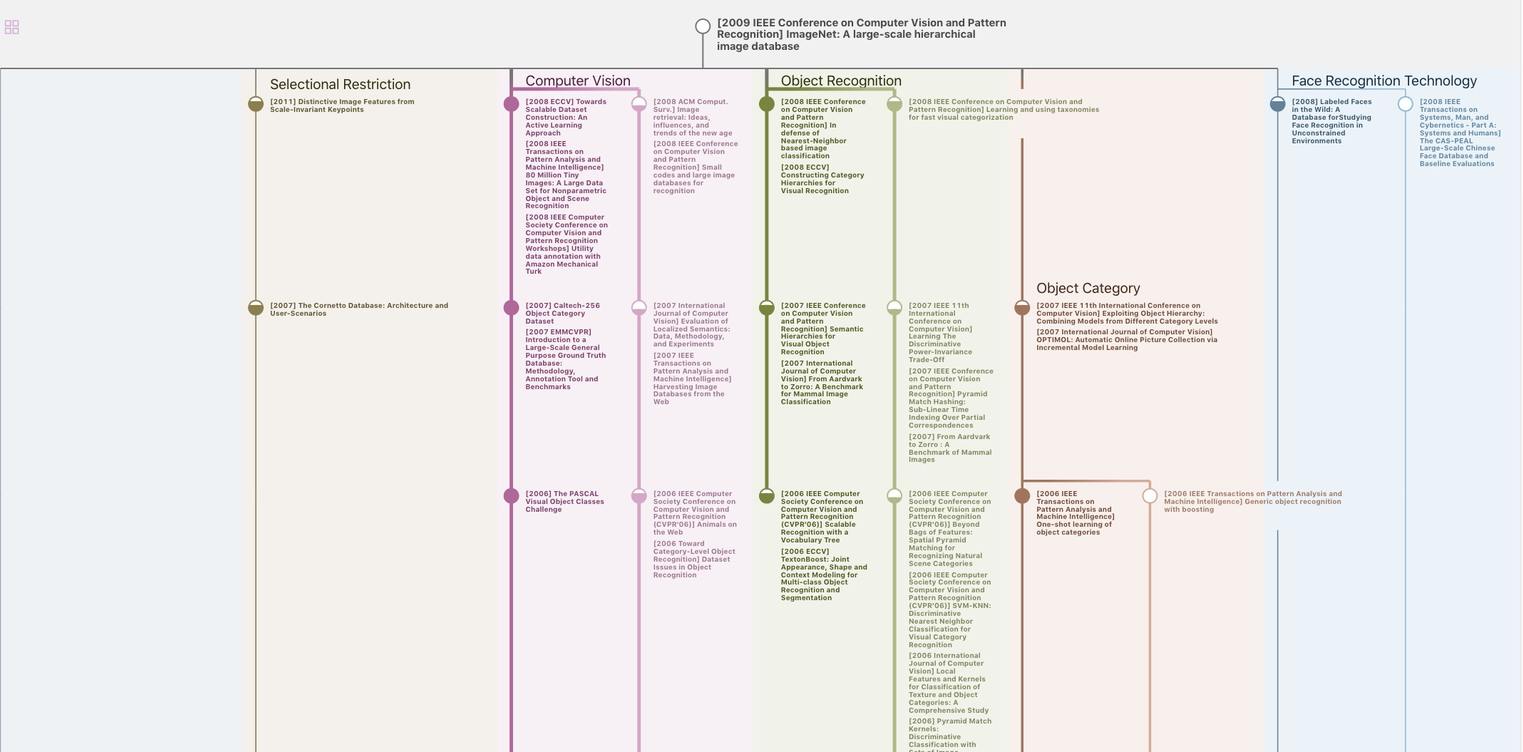
生成溯源树,研究论文发展脉络
Chat Paper
正在生成论文摘要