Operational low-flow forecasting using Long Short-Term Memory networks
crossref(2024)
摘要
This study delves into the potential application of Long Short-Term Memory networks (LSTMs) for low-flow forecasting for the Rhine River at Lobith on a daily basis, extending up to 46 days ahead. The study introduces an innovative LSTM-based model architecture which incorporates both historical observations and forecasted meteorological data for multi-step discharge time series forecasting. The feature and target selection experiment reveals that subbasin-averaged meteorological variables significantly improves model performance compared to a basin-averaged approach, and using time-differenced discharge as the target variable boosts short-term forecast accuracy compared to using discharge. While incorporating historical discharge improves discharge forecasting, its impact on predicting time-differenced discharge is less pronounced for short lead times. The model is then trained using the ERA5 dataset as meteorological forcing, and employed for operational forecast with ECMWF seasonal forecast data. In the operational forecast, compared to the benchmark process-based model wflow_sbm, the DL model exhibits skill in forecasting low flows and better accuracy in forecasting drought events within short lead times. This study also explores the flexibility of the DL model through fine-tuning the pretrained model with limited seasonal forecast dataset. The fine-tuning approach reveals marginal short-term enhancements in forecasting low-flow events compared to a non-fine-tuned model. Overall, this study contributes to advancing the field of low-flow forecasting using deep learning approach.
更多查看译文
AI 理解论文
溯源树
样例
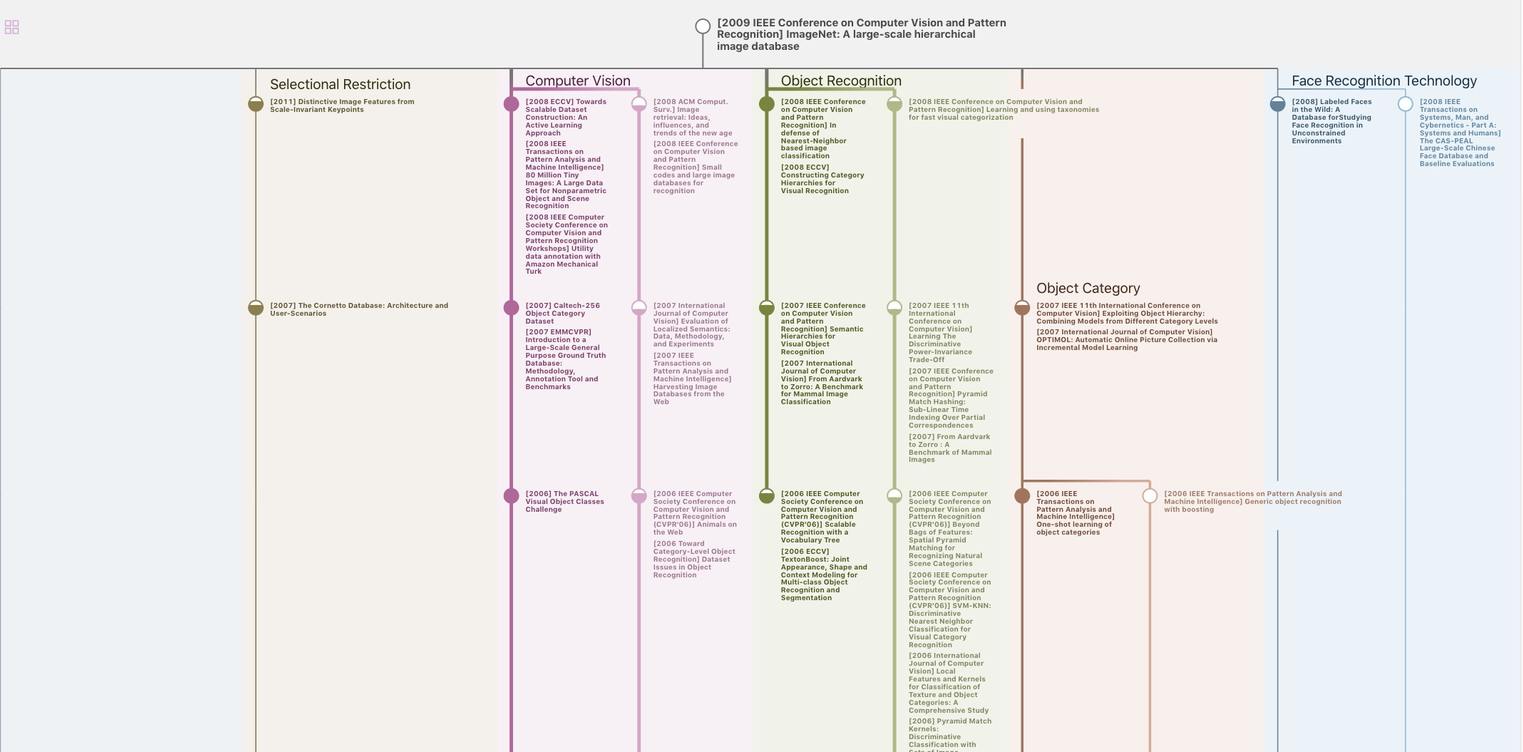
生成溯源树,研究论文发展脉络
Chat Paper
正在生成论文摘要