Estimating Gross Primary Production via Recurrent Neural Networks: A comparative analysis
crossref(2024)
摘要
Quantifying Gross Primary Production (GPP) is fundamental for understanding terrestrial carbon dynamics, particularly in forests. The overarching question we address here is whether integrating remote sensing (RS) with deep learning (DL) methodologies can enhance the estimation of daily forest GPP on a European scale. The Eddy Covariance (EC) method, although widely used to infer ecosystem-scale estimates of GPP from in situ CO2 exchange measurements, suffers from limited global coverage. When EC data are not available, RS data are often employed to estimate GPP by establishing statistical relationships with in situ observations. Recently, Machine Learning (ML) strategies, particularly involving RS and meteorological inputs, have been used for estimating GPP continuously in space and time. However, the potential of DL techniques, particularly those exploiting the sequential characteristics of time series data (i.e. recurrent neural network architectures) in estimating daily forest GPP has not been comprehensively explored. This includes their performance during photosynthetic downregulation (e.g. during climate extremes such as droughts) and an in-depth examination of the importance of the utilised features. This study presents a comparative analysis of three recurrent neural network architectures—Recurrent Neural Networks (RNNs), Gated Recurrent Units (GRUs), and Long-Short Term Memory (LSTMs)—for daily forest GPP estimation across ICOS ecosystem stations. We assess their performance throughout the entire year, during the growing season, and under photosynthetic downregulation events. This assessment utilises RS inputs —optical data from Sentinel-2, Land Surface Temperature (LST) from MODIS, and radar data from Sentinel-1—, combined with potential radiation data. Furthermore, we analysed the importance of these features to provide insights into the complex interactions and dependencies when estimating GPP. Our results indicate that all three architectures yield similar accuracy for full period and growing season GPP estimations, with mean NRMSE values of 0.136 and 0.170, respectively. All models exhibit increased errors while estimating GPP during photosynthetic downregulation events and their performance varies notably, with LSTMs showing the best results (NRMSE=0.202), followed by RNNs (NRMSE=0.214), and GRUs exhibiting the least efficacy (NRMSE=0.276). Additionally, our study underscores the importance of potential radiation as a critical feature influencing the GPP response, with LST data proving particularly valuable when estimating GPP during photosynthetic downregulation events, more so than optical or radar data. These findings suggest that recurrent neural network architectures, especially LSTMs, are effective in daily GPP estimations, with slight performance decreases during climate extreme conditions. The study also reveals the efficacy of combining RS data with potential radiation for accurate forest GPP estimation, with LST data being especially crucial when estimating GPP during photosynthetic downregulation events. Our research paves the way for further exploration into recurrent models and innovative architectures, with an emphasis on overcoming the challenges in modelling climate-induced GPP extremes.
更多查看译文
AI 理解论文
溯源树
样例
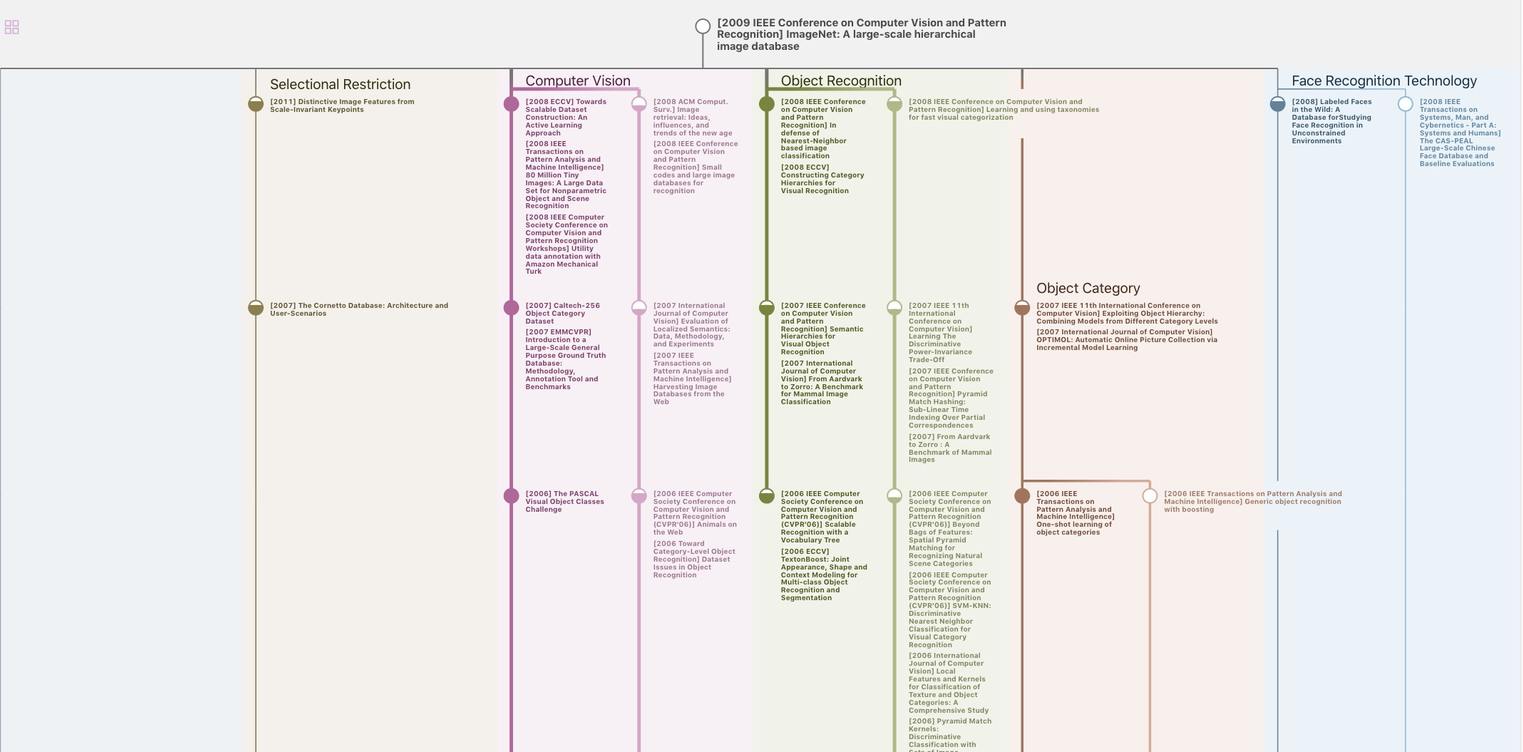
生成溯源树,研究论文发展脉络
Chat Paper
正在生成论文摘要