The global interdependence patterns of extreme-rainfall events
crossref(2024)
摘要
As a powerful data-driven technology, the complex network paradigm has contributed significantly to the studies of spatio-temporal patterns of climate phenomena at different scales, such as El Niño–Southern Oscillation, Indian Ocean Dipole, and monsoon. In this work, we study the global extreme-rainfall patterns, which can potentially be used to improve the predictability of extreme events. The idea is to identify regions of similar extreme-rainfall patterns. For this, we propose a network-based clustering workflow which includes unsupervised learning. More precisely, this workflow combines consensus clustering and mutual correspondences. By applying this workflow to two satellite-derived precipitation datasets, we identify two main global interdependence structures of extreme rainfall, during boreal summer. These two structures are consistent and robust. From a climatological point view, they explicitly manifest the primary intraseasonal variability in the context of the global monsoon, in particular, the “monsoon jump” over both East Asia and West Africa, and the mid-summer drought over Central America and southern Mexico. We highlight the advantage of network-based clustering in (i) decoding the spatio-temporal patterns of climate variability and in (ii) the intercomparison of these patterns, especially regarding their spatial distributions over different datasets.
更多查看译文
AI 理解论文
溯源树
样例
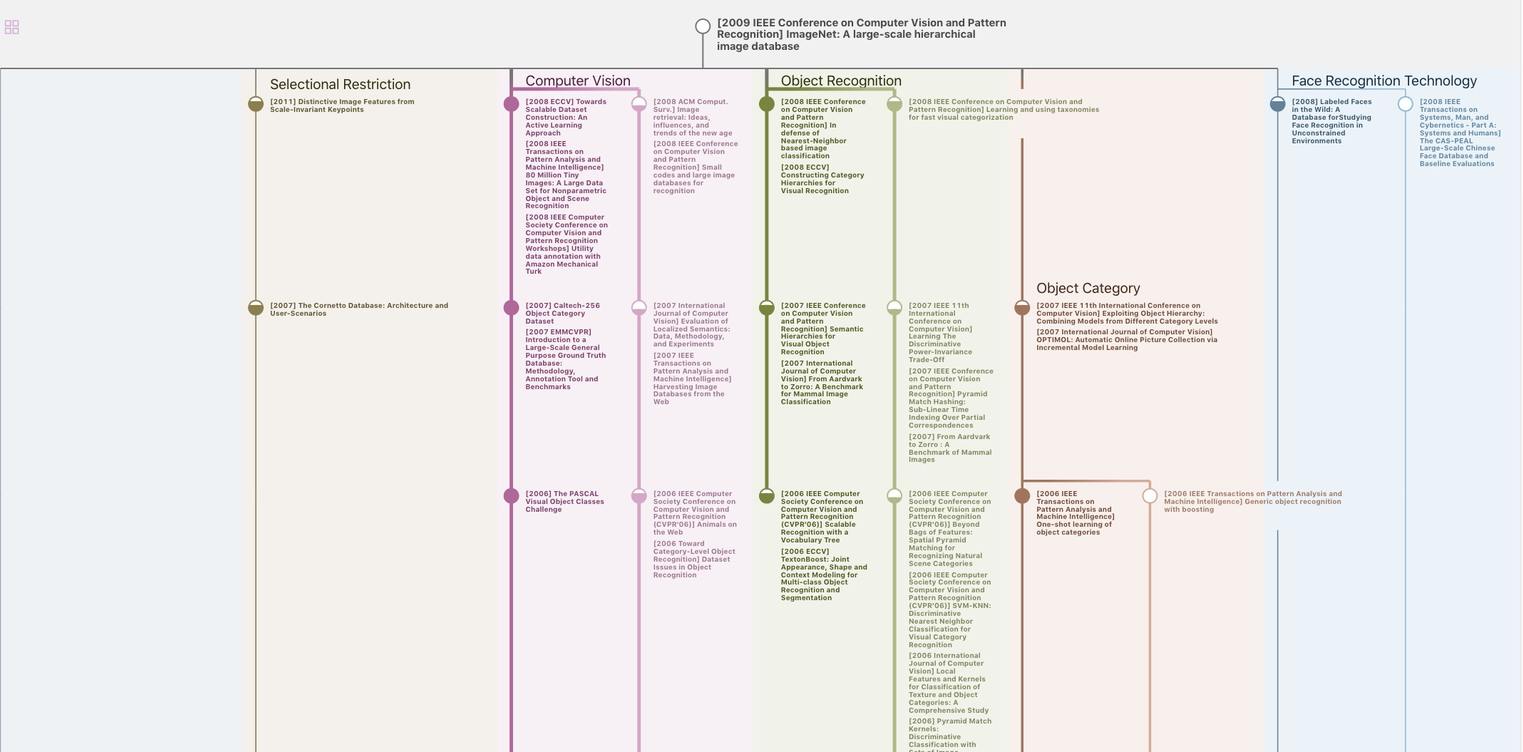
生成溯源树,研究论文发展脉络
Chat Paper
正在生成论文摘要