One-stop multiscale reconciliation attention network with scribble supervision for salient object detection in optical remote sensing images
Applied Intelligence(2024)
摘要
Salient object detection in optical remote sensing images (RSI-SOD) faces significant challenges due to the unique characteristics of RSI imaging. Existing methods heavily rely on labor-intensive pixel-level annotations and overlook the potential of low-cost sparse annotations. Moreover, weakly supervised RSI-SOD methods introduce multiple sparse annotations and training processes, leading to a multistaged SOD task and considerable performance gaps compared to fully supervised approaches. To address these issues, we propose a one-stop end-to-end RSI-SOD method that solely relies on scribble annotations. Our framework, named the one-stop multiscale reconciliation attention network (OMRA-Net), features encoding, reconciliation, polishing, and convergence layers for effective feature extraction, reconciliation, polishing, and object structure restoration. Evaluation on publicly available datasets demonstrates that OMRA-Net outperforms existing weakly supervised and unsupervised SOD methods, achieving comparable or superior performance to fully supervised models. Ablation studies further validate the effectiveness of our proposed model design.
更多查看译文
关键词
Salient object detection,Optical remote sensing images,Weakly supervised learning,Scribble supervision,Attention mechanism
AI 理解论文
溯源树
样例
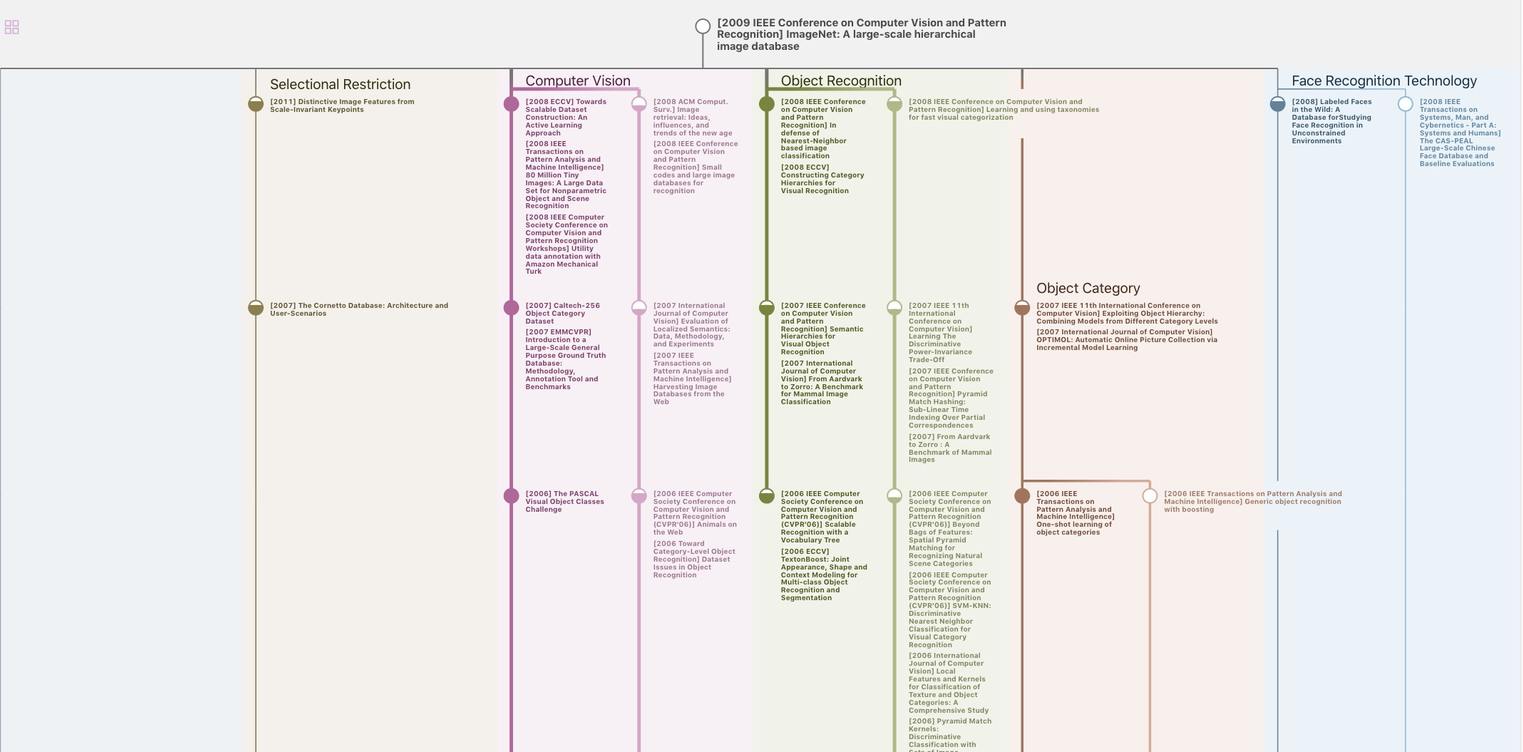
生成溯源树,研究论文发展脉络
Chat Paper
正在生成论文摘要