Ground motion prediction using Physics-Informed Symbolic Learning (PISL)
crossref(2024)
摘要
Ground Motion Models (GMM) are an essential component in seismic hazard analysis. In recent years, Machine Learning (ML) methods have made significant progress in advancing GMM research. However, their effectiveness is hindered by certain limitations. Firstly, some ML methods, such as neural networks, lack interpretability, which poses challenges for researchers and engineers. Secondly, these methods may have limited extrapolation capabilities and may struggle to accurately capture data distribution characteristics when predicting scenarios beyond the scope of the training data. To address these shortcomings, we propose a physics-informed symbolic learning (PISL) approach for constructing GMMs. Symbolic learning extracts an interpretable expression from data using sparse regression. To tackle sparse data issues in the near-field of large earthquakes, we have introduced a near-field saturation factor into the model. This factor contains physics information and guides the model to accurately capture near-field saturation properties even with limited data. We used the NGA-West2 database to develop GMMs for Peak Ground Acceleration (PGA), Peak Ground Velocity (PGV), and Pseudo-Spectral Acceleration (PSA) within the period range of 0.01s-10s. The study conducted comparative analyses using Mw-scaling, RJB-scaling, and VS30-scaling cases with empirical regression models. Model stability was assessed through residual calculations and standard deviation analysis. The results indicate that our model predictions are comparable to those of classical empirical models, with no significant differences in residual distribution and standard deviations. The extrapolation ability of the symbolic learning approach was validated using seismic events outside the training data, including the Wenchuan earthquake and Turkey earthquake. In conclusion, the method described above integrates physical knowledge and data-driven approaches, simplifying the equations while achieving similar results. Symbolic learning methods then provide a new perspective for the application of ML in engineering seismology.
更多查看译文
AI 理解论文
溯源树
样例
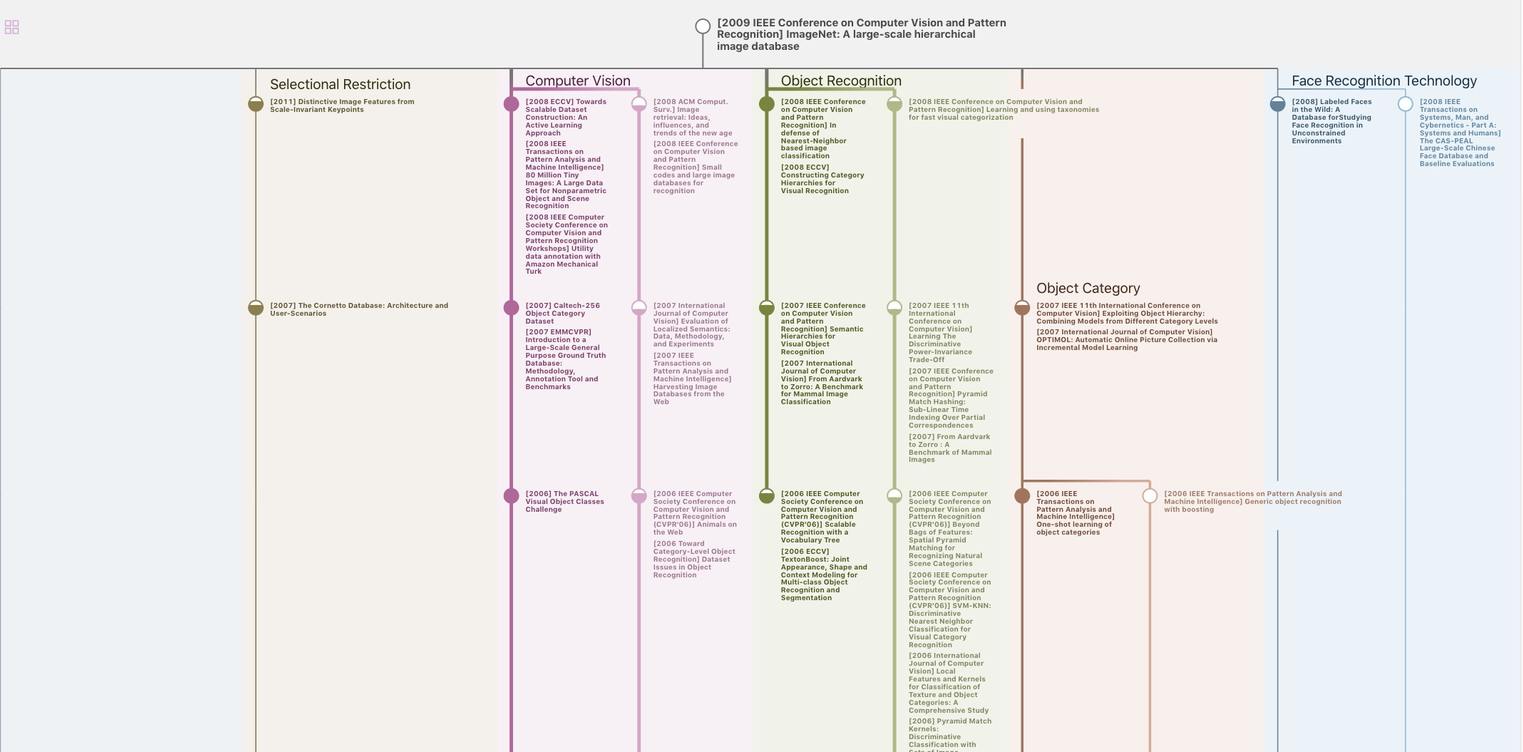
生成溯源树,研究论文发展脉络
Chat Paper
正在生成论文摘要