Multivariate analysis for data mining to characterize poultry house environment in winter
Poultry Science(2024)
摘要
The processing and analysis of massive high-dimensional datasets are important issues in precision livestock farming (PLF). This study explored the use of multivariate analysis tools to analyze environmental data from multiple sensors located throughout a broiler house. An experiment was conducted to collect a comprehensive set of environmental data including particulate matter (TSP, PM10, and PM2.5), ammonia, carbon dioxide, air temperature, relative humidity, and in-cage and aisle wind speeds from 60 locations in a typical commercial broiler house. The dataset was divided into three growth phases (weeks 1–3, 4–6, and 7–9). Spearman's correlation analysis and principal component analysis (PCA) were used to investigate the latent associations between environmental variables resulting in the identification of variables that played important roles in indoor air quality. Three cluster analysis methods; k-means, k-medoids, and fuzzy c-means cluster analysis (FCM), were used to group the measured parameters based on their environmental impact in the broiler house. In general, the Spearman and PCA results showed that the in-cage wind speed, aisle wind speed, and relative humidity played critical roles in indoor air quality distribution during broiler rearing. All three clustering methods were found to be suitable for grouping data, with FCM outperforming the other two. Using data clustering, the broiler house spaces were divided into three, two, and two subspaces (clusters) for weeks 1–3, 4–6, and 7–9, respectively. The subspace in the center of the house had a poorer air quality than other subspaces.
更多查看译文
关键词
broiler house,microclimate,air quality,multivariate analysis,data mining
AI 理解论文
溯源树
样例
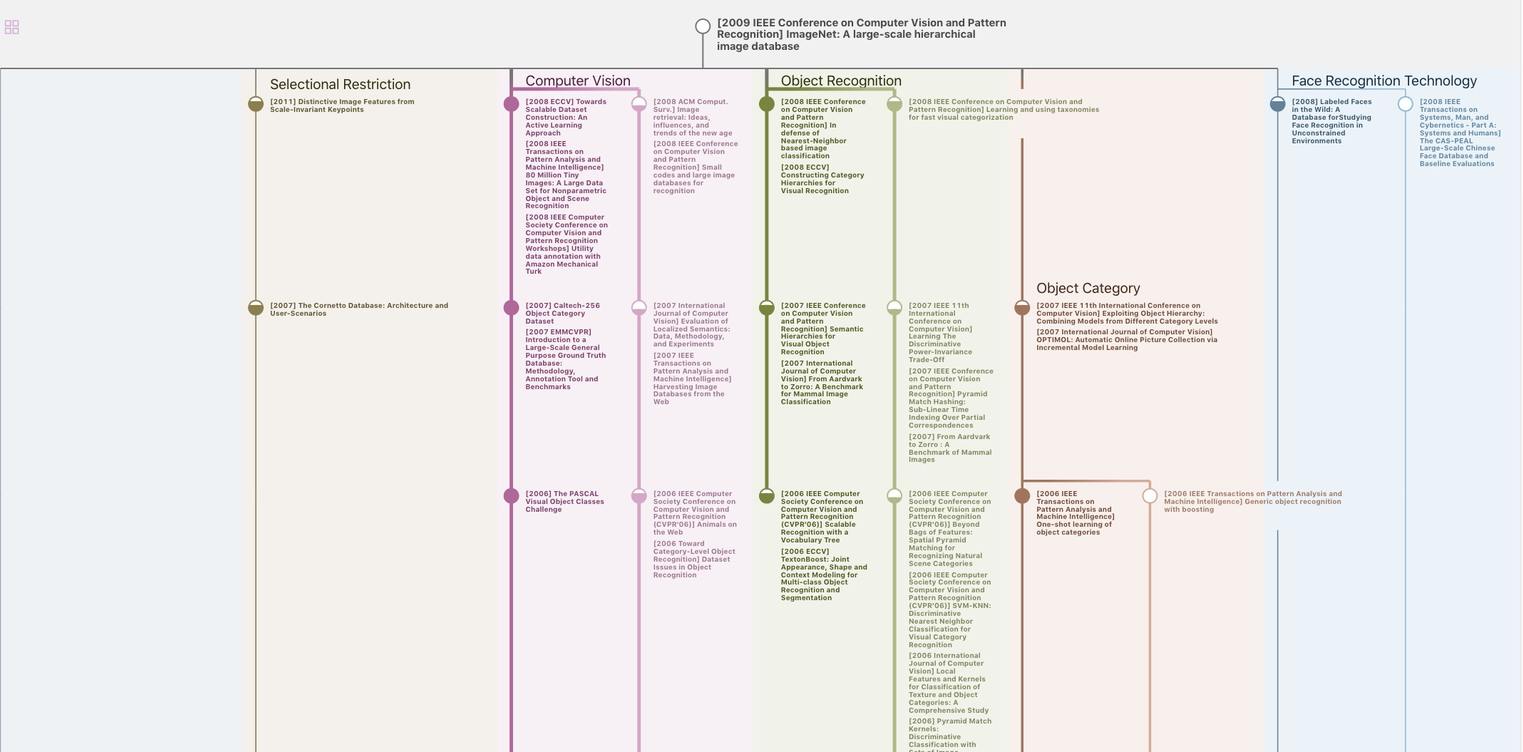
生成溯源树,研究论文发展脉络
Chat Paper
正在生成论文摘要