The Impact of GRACE Data Assimilation on Water Storage Dynamics in CLM3.5 and CLM5
crossref(2024)
摘要
The GRACE (Gravity Recovery And Climate Experiment) satellite mission as well as its successor GRACE Follow-On have monitored global and regional variability of total water storage (TWS) for the past two decades. Assimilating observations from these missions into hydrological models helps to improve modeled water storages and fluxes, to overcome deficits arising from simplifications or processes that are not considered in the model (e.g. unmodeled anthropogenic impacts), and to disaggregate GRACE observations temporally and spatially. Determining the optimal approach for assimilating these observations into hydrological models remains an ongoing area of research. The choice often depends on specific applications and the characteristics of the model itself. In this study, we analyze the water storage dynamics of two versions of the Community Land Model (CLM) - versions 3.5 and 5 - within a GRACE data assimilation framework over a 12.5 km grid covering Europe. The analysis focuses on assessing (i) the skill of both models without data assimilation, (ii) the impact of GRACE data assimilation on the model performance and (iii) the distribution of assimilation increments to different storage compartments. We evaluate water storages and fluxes simulated by both models against independent observations such as discharge from river gauges and satellite derived soil moisture. The results offer valuable insights into the impact of advancements made in biophysical processes and the representation of the carbon cycle in CLM5. Furthermore, we discuss the effectiveness of GRACE data assimilation and its influence on the behavior of CLM3.5 and CLM5, analyzing whether the assimilation helps to address differences between the two model versions - particularly considering the advancements in CLM5 - which would underline the ability of GRACE data assimilation in mitigating model deficits.
更多查看译文
AI 理解论文
溯源树
样例
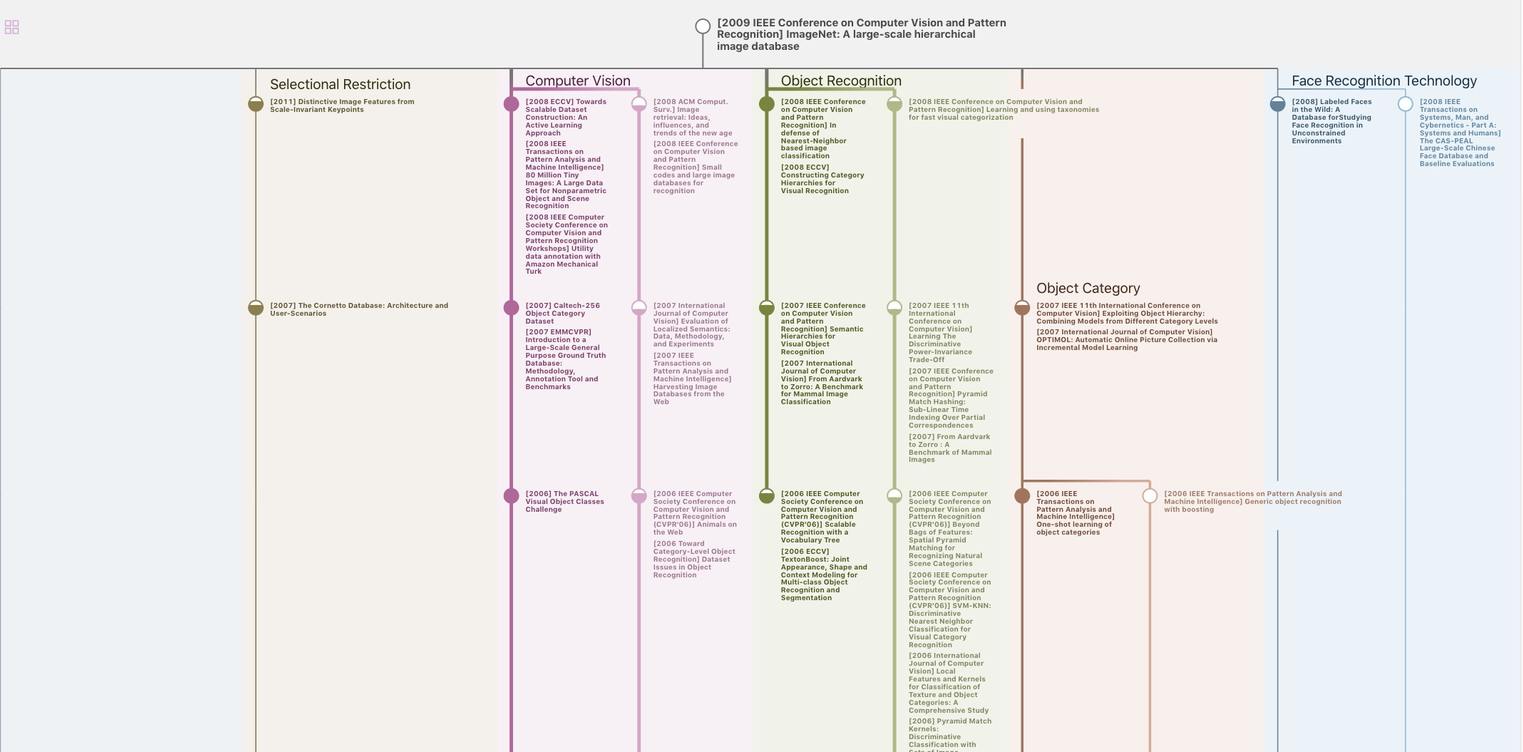
生成溯源树,研究论文发展脉络
Chat Paper
正在生成论文摘要