Inferring soil water movement from soil moisture distribution with deep learning method
crossref(2024)
摘要
Soil moisture is an essential variable that influencing hydrological processes and water cycles within the climate system. Soil moisture movement alters the spatial and temporal distribution of soil moisture and further influences the water and heat cycle. The relationship between them is usually described by Richardson–Richards equation (RRE). However, the dual nonlinearity of this equation and the stringent requirements for initial and boundary conditions have posed challenges for the development of unsaturated soil water simulation based on the RRE. The development of machine learning brings new opportunities, yet most studies focused on the prediction of soil moisture, only a few used it to explore the underlying mechanism. Here, we implemented recurrent neural network to predict soil moisture movement at three dimensions. Soil moisture distribution at three dimensions, simulated by a physical-based hydrological model, the Integrated Hydrology model (InHM) based on RRE, was used as input, along with climate forcing as well as topographical characteristics. The model provided reasonable prediction of soil water movement at all three dimensions across the points in the study catchment. This provides a framework for an alternative way to predict soil water movement and subsurface flow path, with more field measurements, it could help us improve our understanding in subsurface water movement, providing a novel and viable solution to further promote unsaturated soil moisture simulation.
更多查看译文
AI 理解论文
溯源树
样例
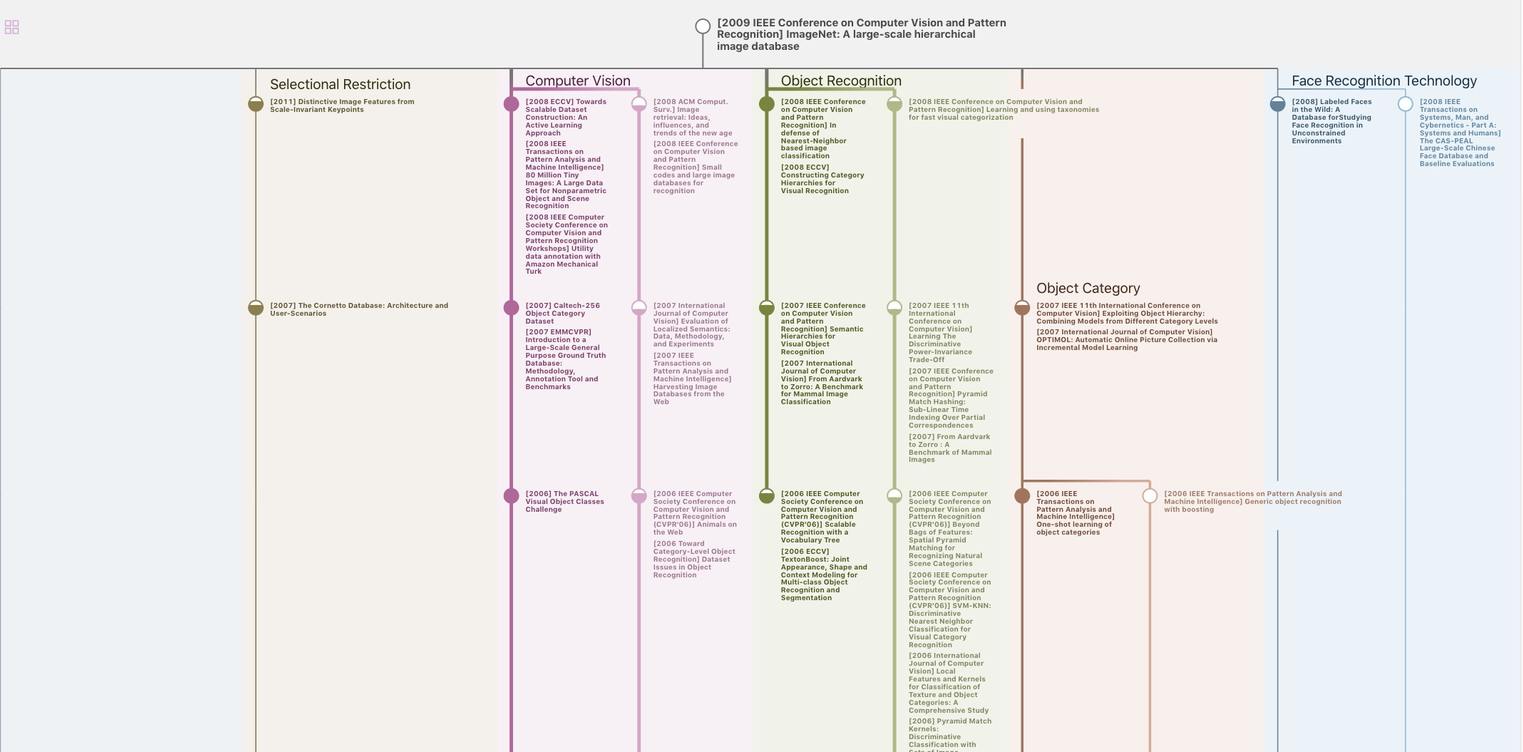
生成溯源树,研究论文发展脉络
Chat Paper
正在生成论文摘要