Small-world properties of eye-movement time series assisted in identifying children at high risk for dyslexia
Biomedical Signal Processing and Control(2024)
摘要
Previous studies suggested constructing machine learning based classification (MLC) models for classifying children with high risk (HR) and those with low risk (LR) of reading difficulties, whose features were standard eye-movement metrics and their derived variables. However, these standard eye-movement metrics were primarily concerned with counting the number and duration of eye-movement events in specific stimulus regions, rather than accounting for the moment-to-moment changes in gaze behaviors during reading tasks. This study proposed an idea of converting eye-movement time-series into a complex network [referred to as eye-gaze network (EGN)], allowing us to extract features underlying the moment-to-moment changes in gaze behaviors during reading tasks. To illustrate its feasibility, we adopted a publicly accessible database, involving eye-movement data collected from 97 HR and 88 LR children. For each participant, we first reorganized the whole eye-movement time-series into different segments and mapped each segment of time-series into an EGN, then calculated the small-world index (SWI) of each EGN as feature parameter, and finally constructed a number of MLC models using the linear discriminant analysis. Aberrant (weaker) small-world architecture, as a potential biological mechanism, was observed in the HR group. Additionally, our results illustrated the superior performance of MLC models with SWI values as feature parameters, compared to those using standard eye-movement metrics and others using different machine learning algorithms.
更多查看译文
关键词
Dyslexia,Eye-movement time-series,Complex network,Small-world architecture,Pattern classification
AI 理解论文
溯源树
样例
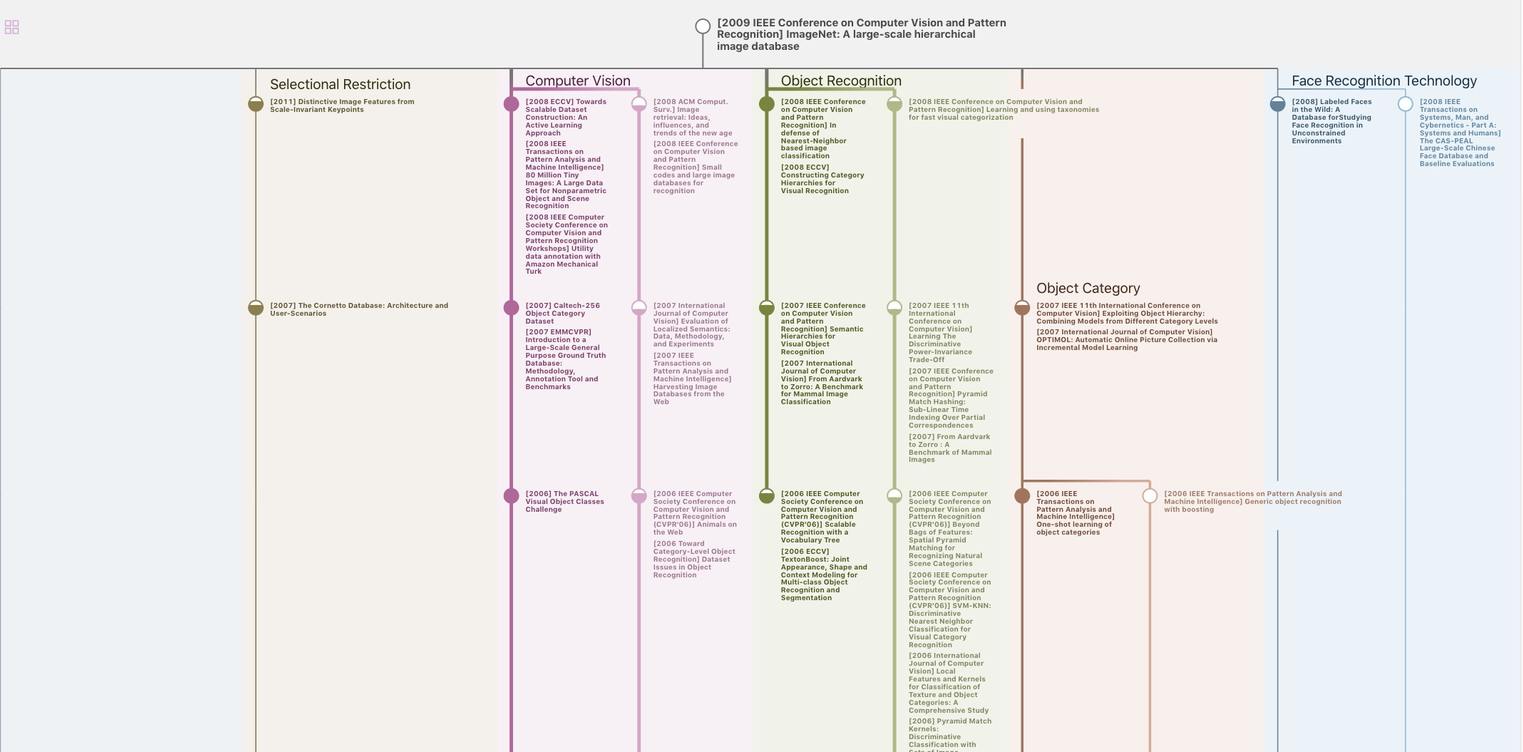
生成溯源树,研究论文发展脉络
Chat Paper
正在生成论文摘要