Domain-Inspired Sharpness Aware Minimization Under Domain Shifts
ICLR 2024(2024)
摘要
This paper presents a Domain-Inspired Sharpness Aware Minimization (DISAM) algorithm for optimization under domain shifts. It is motivated by the inconsistent convergence degree of SAM across different domains, which induces optimization bias towards certain domains and thus impairs the overall convergence. To address this issue, we consider the domain-level convergence consistency in the sharpness estimation to prevent the overwhelming (deficient) perturbations for less (well) optimized domains. Specifically, DISAM introduces the constraint of minimizing variance in the domain loss, which allows the elastic gradient calibration in perturbation generation: when one domain is optimized above the averaging level \textit{w.r.t.} loss, the gradient perturbation towards that domain will be weakened automatically, and vice versa. Under this mechanism, we theoretically show that DISAM can achieve faster overall convergence and improved generalization in principle when inconsistent convergence emerges. Extensive experiments on various domain generalization benchmarks show the superiority of DISAM over a range of state-of-the-art methods. Furthermore, we show the superior efficiency of DISAM in parameter-efficient fine-tuning combined with the pretraining models.
更多查看译文
关键词
generalization,sharpness-aware minimization,domain shift
AI 理解论文
溯源树
样例
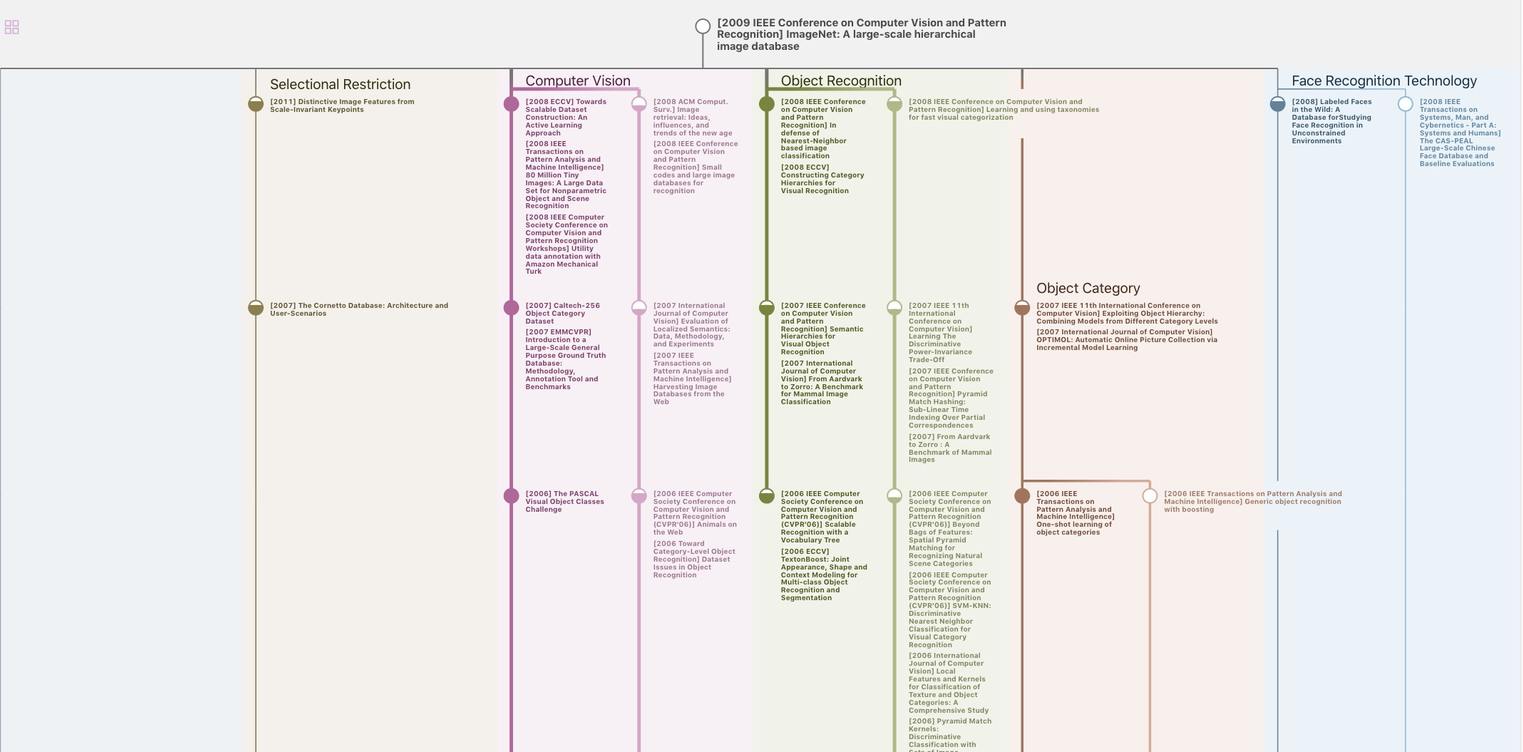
生成溯源树,研究论文发展脉络
Chat Paper
正在生成论文摘要