Who to imitate: Imitating desired behavior from divserse multi-agent datasets
ICLR 2024(2024)
摘要
AI agents are commonly trained with large datasets of demonstrations of human behavior.
However, not all behaviors are equally safe or desirable.
Desired characteristics for an AI agent can be expressed by assigning desirability scores, which we assume are assigned to collective trajectories, but not to individual behaviors.
For example, in a dataset of vehicle interactions, these scores might relate to the number of incidents that occurred.
We first assess the effect of each individual agent's behavior on the collective desirability score, e.g., assessing how likely an agent is to cause incidents.
This allows us to afterward only imitate agents with desired behavior, e.g., only imitating agents that are unlikely to cause incidents.
To enable this, we propose the concept of an agent's \textit{Exchange Value}, which quantifies an individual agent's contribution to the collective desirability score. This is expressed as the expected change in desirability score when substituting the agent for a randomly selected agent.
We propose additional methods for estimating Exchange Values from real-world datasets, enabling us to learn aligned imitation policies that outperform relevant baselines.
更多查看译文
关键词
multi-agent systems,imitation learning,credit assignment,learning from human data
AI 理解论文
溯源树
样例
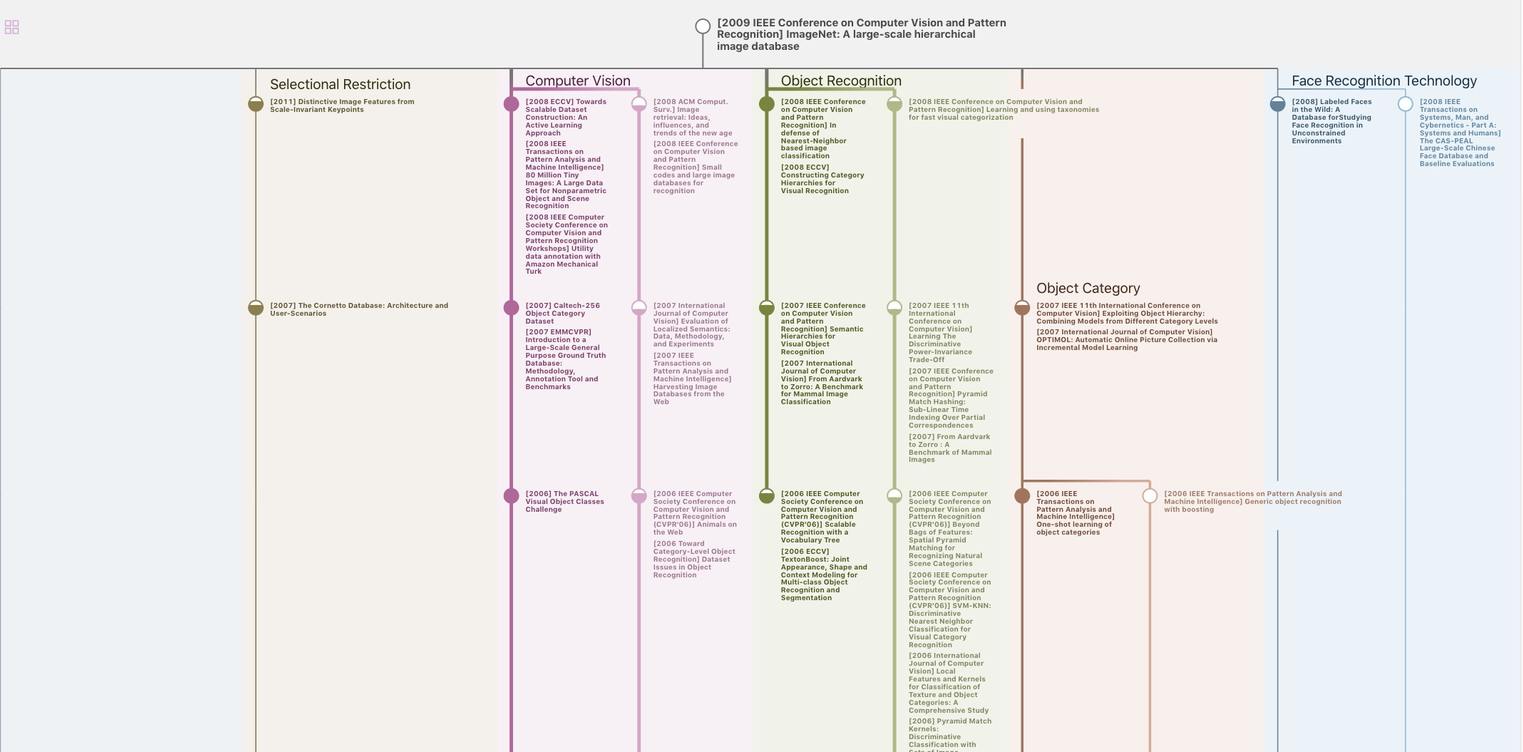
生成溯源树,研究论文发展脉络
Chat Paper
正在生成论文摘要