Neurosymbolic Grounding for Compositional Generalization
ICLR 2024(2024)
摘要
We introduce Cosmos, a framework for object-centric world modeling that is designed for compositional generalization (CG), i.e., high performance on unseen input scenes obtained through the composition of known visual "atoms." The central insight behind Cosmos is the use of a novel form of neurosymbolic grounding. Specifically, the framework introduces two new tools: (i) neurosymbolic scene encodings, which represent each entity in a scene using a real vector computed using a neural encoder, as well as a vector of composable symbols describing attributes of the entity, and (ii) a neurosymbolic attention mechanism that binds these entities to learned rules of interaction. Cosmos is end-to-end differentiable; also, unlike traditional neurosymbolic methods that require representations to be manually mapped to symbols, it computes an entity's symbolic attributes using vision-language foundation models. Through an evaluation that considers two different forms of CG on an established blocks-pushing domain, we show that the framework establishes a new state-of-the-art for CG in world modeling.
更多查看译文
关键词
neurosymbolic learning,machine learning,world modeling,compositional generalization
AI 理解论文
溯源树
样例
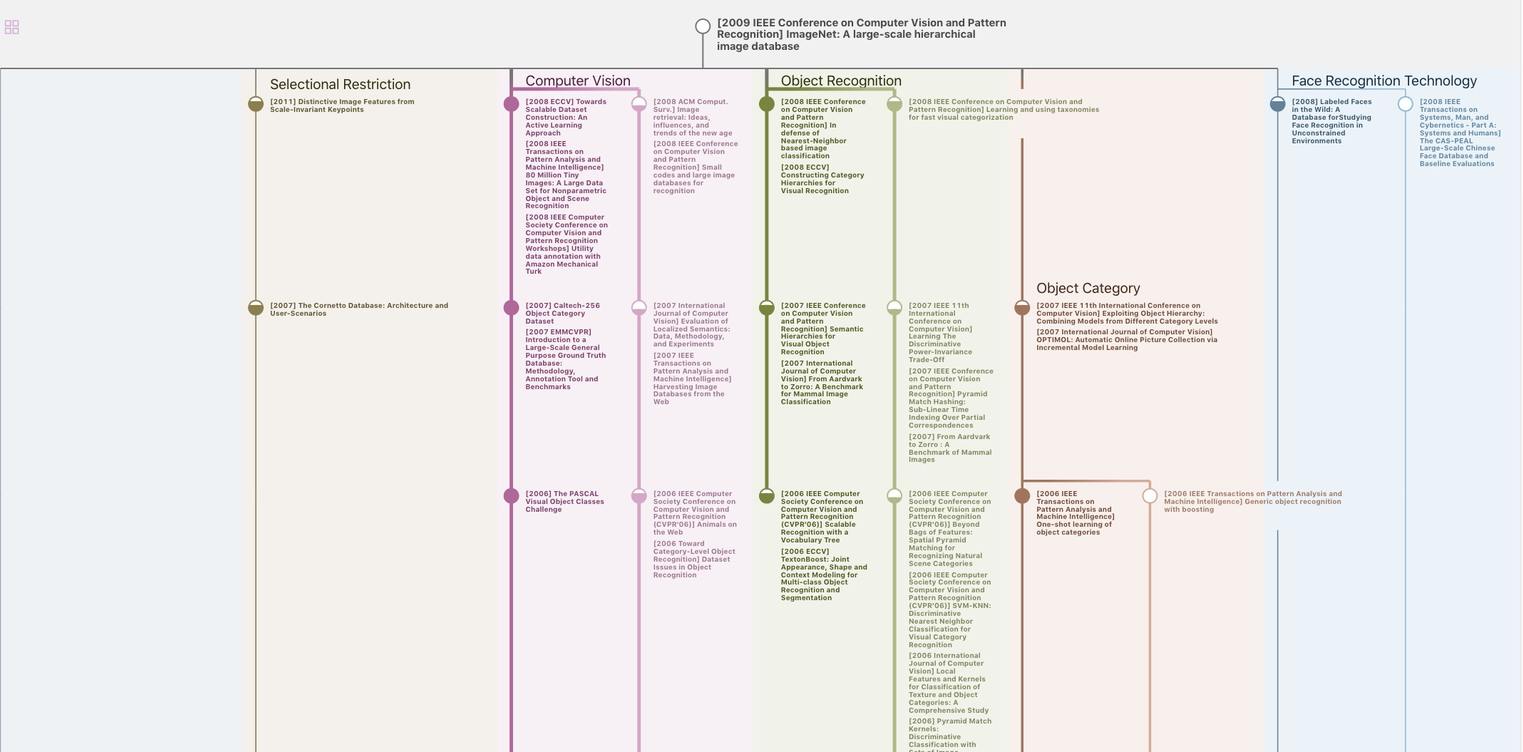
生成溯源树,研究论文发展脉络
Chat Paper
正在生成论文摘要