CfCV: Towards algorithmic debiasing in machine learning experiment
Intelligent Systems with Applications(2024)
摘要
Algorithmic failure in application leading to unfairness or bias typically results from data-inconsistent, diversity biases, inclusion biases, and under-representation, among many others, leading to imbalances in representation for training and validation. Cross-validation (CV) techniques are relevant in addressing this inconsistency from training to validation point. As a result, the essence of CV is to validate algorithm abilities to predict new data that are not part of the training set and to prompt issues such as overfitting or selection bias. This study considered the linkage of Inclusion, Participation, and Reciprocity (IPR) in data splitting for a sensitive attribute and caters for population grouping representativeness in splitting. The study remodified the Pre-In-Post (P-I-P) processing approach to accommodate various sensitive attribute levels within any training set and performed simulation experiments and real-life applications. It then conducted a comparative performance analysis with the two most notable CV techniques. The study innovative approach (CfCV) outperformed the existing CVs - Vfold and HoldOut; in experiments [RMSECfCV = 0.88; RMSEVfold = 0.98; RMSEHoldout = 4.69 | AccuracyCfCV = 99.75%; AccuracyVfold = 99.50%; AccuracyHoldOut = 85.50%] and applications [RMSECfCV = 0.59; RMSEVfold = 1.61; RMSEHoldout = 1.96 | AccuracyCfCV = 84%; AccuracyVfold = 81%; AccuracyHoldOut = 52%]. This study recommends the adoption of IPR in data splitting for machine experiments built for human-machine intelligence systems and concludes that machine learning experiments would be fairer if the concept of IPR formed the foundation of the human-machine intelligence framework.
更多查看译文
关键词
Algorithmic debiasing,Algorithmic fairness,Human-machine intelligence,Cross-Validation,IPR - Inclusion,Participation,& Reciprocity,[P-I-P] - Pre-In-Post Processing
AI 理解论文
溯源树
样例
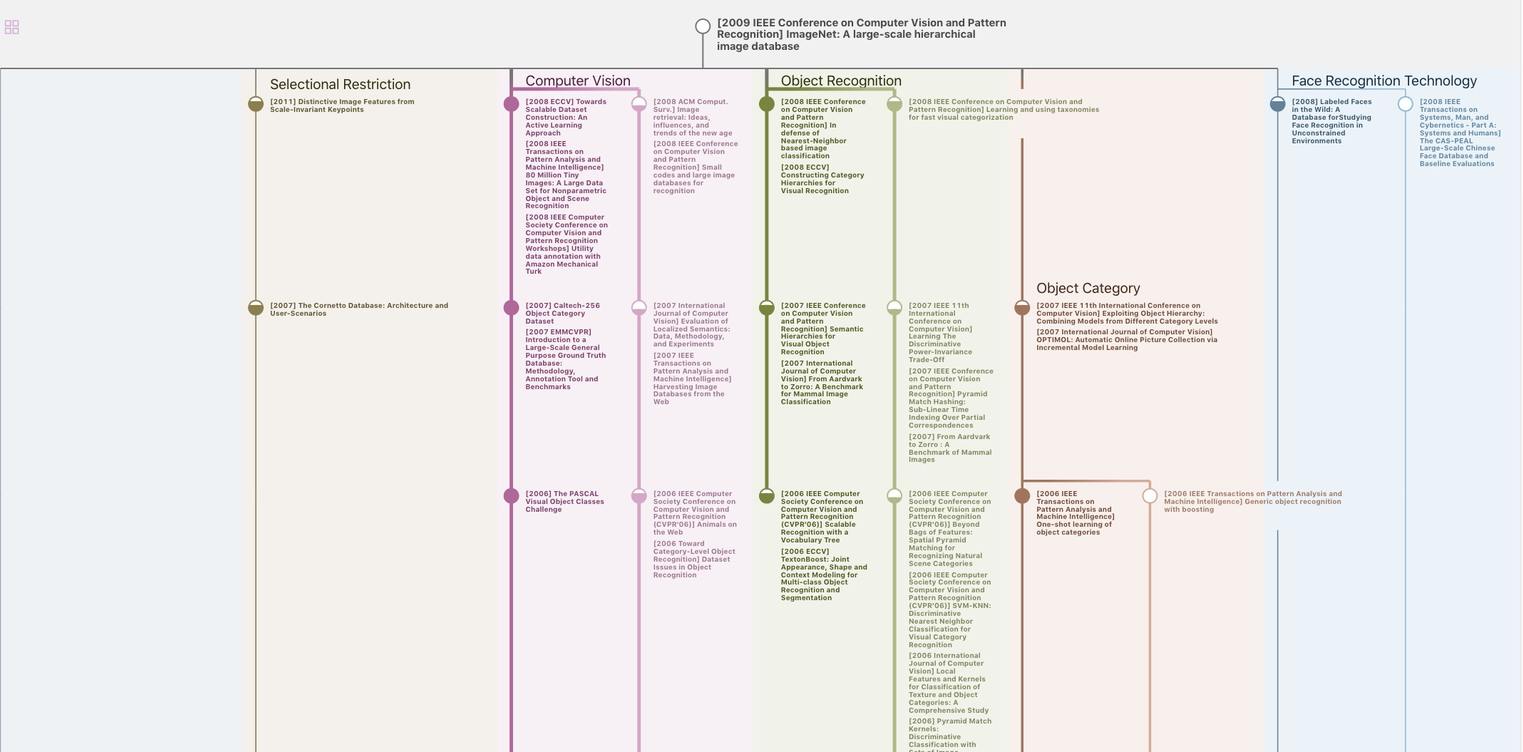
生成溯源树,研究论文发展脉络
Chat Paper
正在生成论文摘要