Super Resolution of Pulmonary Nodules Target Reconstruction Using a Two-Channel GAN Models
Academic Radiology(2024)
摘要
Rationale and Objectives
To develop a Dual generative-adversarial-network (GAN) Cascaded Network (DGCN) for generating super-resolution computed tomography (SRCT) images from normal-resolution CT (NRCT) images and evaluate the performance of DGCN in multi-center datasets.
Materials and Methods
This retrospective study included 278 patients with chest CT from two hospitals between January 2020 and June 2023, and each patient had all three NRCT (512×512 matrix CT images with a resolution of 0.70 mm, 0.70 mm,1.0 mm), high-resolution CT (HRCT, 1024×1024 matrix CT images with a resolution of 0.35 mm, 0.35 mm,1.0 mm), and ultra-high-resolution CT (UHRCT, 1024×1024 matrix CT images with a resolution of 0.17 mm, 0.17 mm, 0.5 mm) examinations. Initially, a deep chest CT super-resolution residual network (DCRN) was built to generate HRCT from NRCT. Subsequently, we employed the DCRN as a pre-trained model for the training of DGCN to further enhance resolution along all three axes, ultimately yielding SRCT. PSNR, SSIM, FID, subjective evaluation scores, and objective evaluation parameters related to pulmonary nodule segmentation in the testing set were recorded and analyzed.
Results
DCRN obtained a PSNR of 52.16, SSIM of 0.9941, FID of 137.713, and an average diameter difference of 0.0981 mm. DGCN obtained a PSNR of 46.50, SSIM of 0.9990, FID of 166.421, and an average diameter difference of 0.0981 mm on 39 testing cases. There were no significant differences between the SRCT and UHRCT images in subjective evaluation.
Conclusion
Our model exhibited a significant enhancement in generating HRCT and SRCT images and outperformed established methods regarding image quality and clinical segmentation accuracy across both internal and external testing datasets.
更多查看译文
关键词
Computed Tomography,Pulmonary Nodules,Deep Learning,Generative Adversarial Networks,Super Resolution
AI 理解论文
溯源树
样例
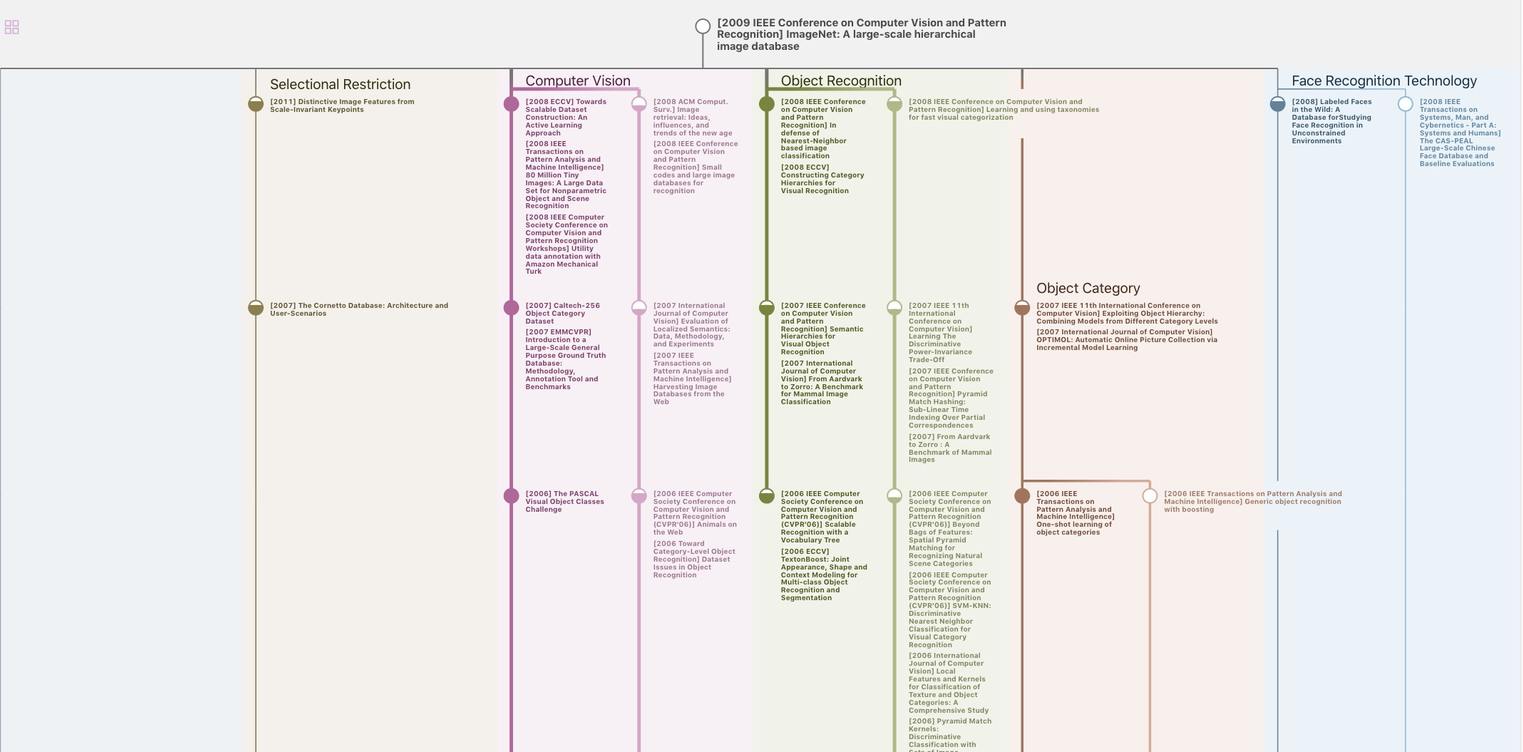
生成溯源树,研究论文发展脉络
Chat Paper
正在生成论文摘要