A Sub-Quadratic Time Algorithm for Robust Sparse Mean Estimation
arxiv(2024)
摘要
We study the algorithmic problem of sparse mean estimation in the presence of
adversarial outliers. Specifically, the algorithm observes a corrupted
set of samples from 𝒩(μ,𝐈_d), where the unknown mean
μ∈ℝ^d is constrained to be k-sparse. A series of prior works
has developed efficient algorithms for robust sparse mean estimation with
sample complexity poly(k,log d, 1/ϵ) and runtime d^2
poly(k,log d,1/ϵ), where ϵ is the fraction of
contamination. In particular, the fastest runtime of existing algorithms is
quadratic (Ω(d^2)), which can be prohibitive in high dimensions. This
quadratic barrier in the runtime stems from the reliance of these algorithms on
the sample covariance matrix, which is of size d^2. Our main contribution is
an algorithm for robust sparse mean estimation which runs in
subquadratic time using poly(k,log d,1/ϵ) samples. We
also provide analogous results for robust sparse PCA. Our results build on
algorithmic advances in detecting weak correlations, a generalized version of
the light-bulb problem by Valiant.
更多查看译文
AI 理解论文
溯源树
样例
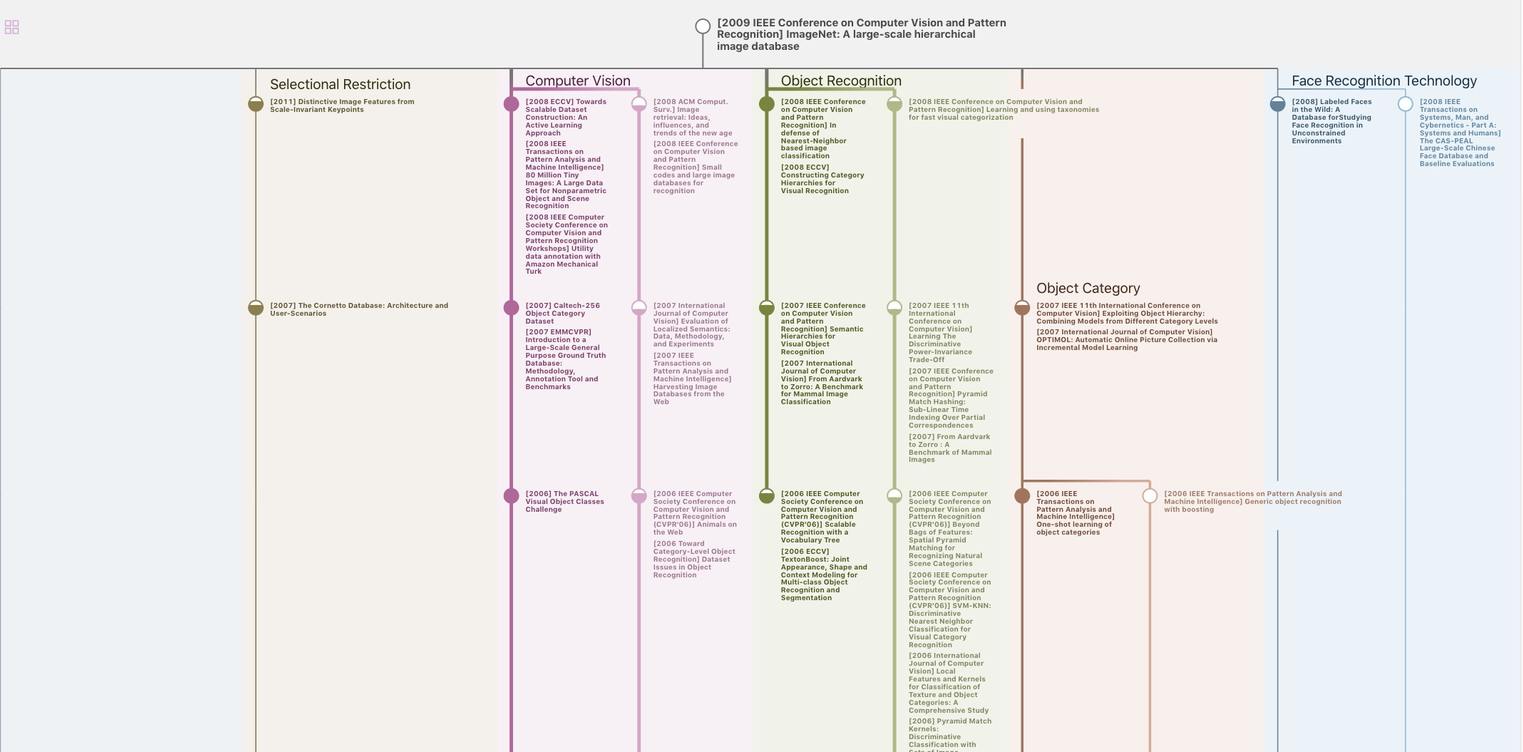
生成溯源树,研究论文发展脉络
Chat Paper
正在生成论文摘要