Weakly-semi supervised extraction of rooftop photovoltaics from high-resolution images based on segment anything model and class activation map
Applied Energy(2024)
摘要
Accurate extraction of rooftop photovoltaic from high-resolution remote sensing imagery is pivotal for propelling green energy planning and development. Conventional deep learning techniques often require labor-intensive pixel-level annotations, presenting substantial limitations. To overcome this hurdle, we introduce an innovative weakly-semi supervised segmentation framework that strategically employs both a “Segment Anything Model” (SAM) and “Class Activation Maps” (CAM) to produce high-precision and efficient pseudo-labels. To manage the unique error characteristics arising from the fusion of SAM and CAM-derived pseudo-labels, our framework incorporates semi-supervised learning algorithms and a boundary-aware loss function. We conducted experiments on a publicly available dataset, yielding an Intersection over Union (IoU) rate of 74% and an F1 score of 84%. Remarkably, this performance reaches approximately 88% of the benchmark established by fully-supervised methods. Our ablation studies further substantiate the effectiveness of our framework, thereby carving out a new trajectory in the realm of weakly-supervised segmentation. The study also delineates certain limitations, particularly focusing on the granularity of SAM-based segmentation and its implications for large-scale photovoltaic installations. Our methodology not only elevates segmentation accuracy but also substantially alleviates the manual labor required for dataset preparation.
更多查看译文
关键词
Segment anything model,Weakly supervised learning,Semi-supervised learning,Deep learning
AI 理解论文
溯源树
样例
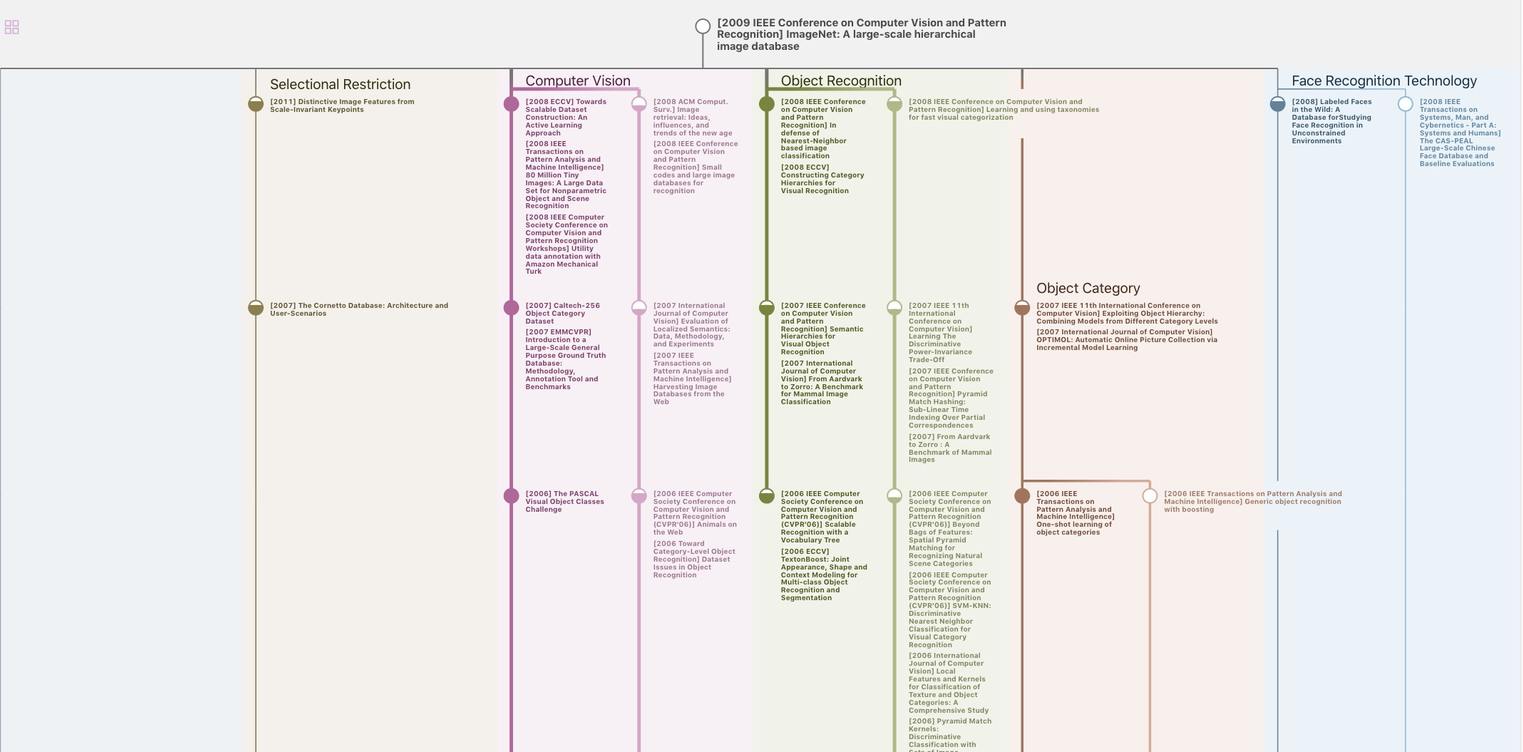
生成溯源树,研究论文发展脉络
Chat Paper
正在生成论文摘要