ViT-UperNet: a hybrid vision transformer with unified-perceptual-parsing network for medical image segmentation
Complex & Intelligent Systems(2024)
摘要
The existing image semantic segmentation models have low accuracy in detecting tiny targets or multi-targets at overlapping regions. This work proposes a hybrid vision transformer with unified-perceptual-parsing network (ViT-UperNet) for medical image segmentation. A self-attention mechanism is embedded in a vision transformer to extract multi-level features. The image features are extracted hierarchically from low to high dimensions using 4 groups of Transformer blocks with different numbers. Then, it uses a unified-perceptual-parsing network based on a feature pyramid network (FPN) and a pyramid pooling module (PPM) for the fusion of multi-scale contextual features and semantic segmentation. FPN can naturally use hierarchical features, and generate strong semantic information on all scales. PPM can better use the global prior knowledge to understand complex scenes, and extract features with global context information to improve segmentation results. In the training process, a scalable self-supervised learner named masked autoencoder is used for pre-training, which strengthens the visual representation ability and improves the efficiency of the feature learning. Experiments are conducted on cardiac magnetic resonance image segmentation where the left and right atrium and ventricle are selected for segmentation. The pixels accuracy is 93.85
更多查看译文
关键词
Medical image segmentation,Deep learning,Self-attention mechanism,Masked autoencoder
AI 理解论文
溯源树
样例
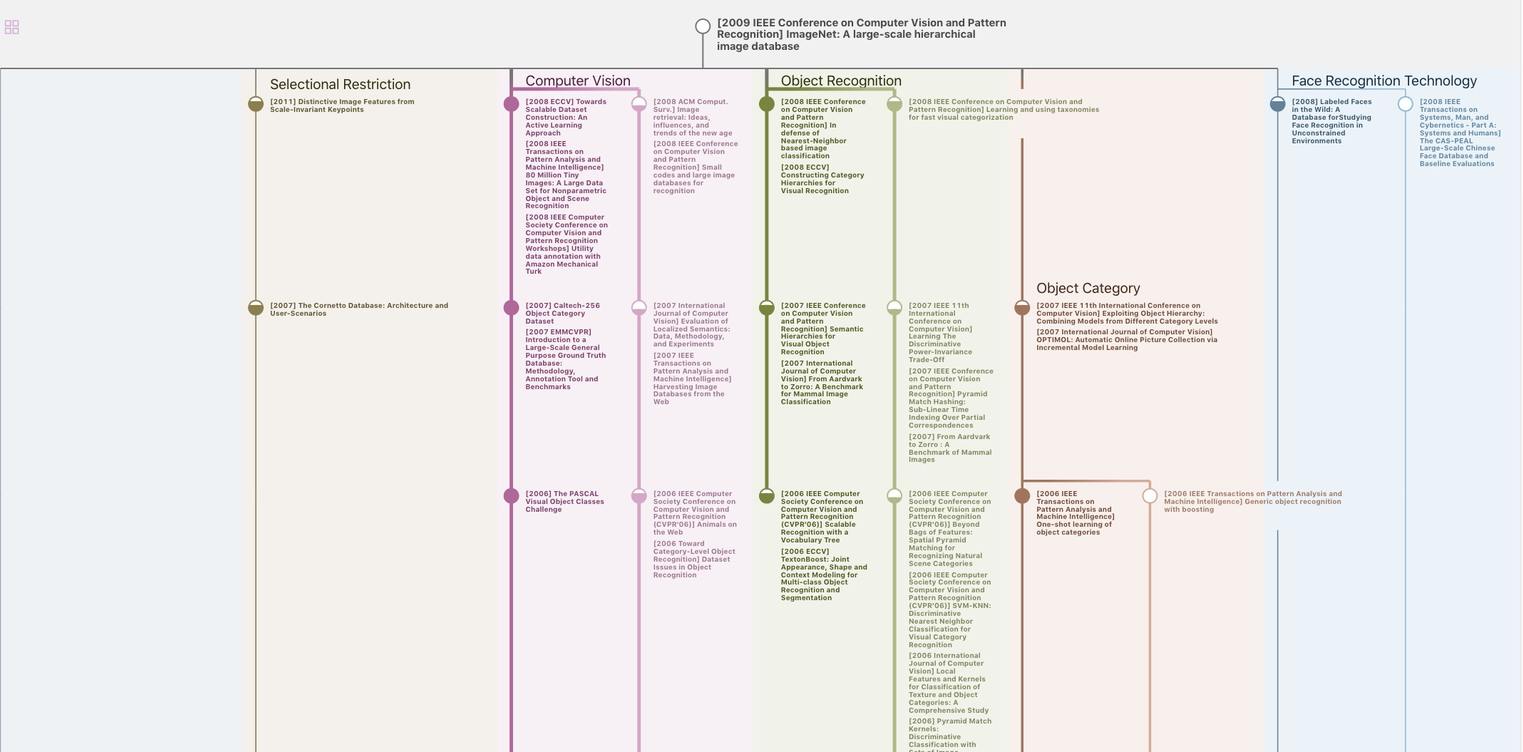
生成溯源树,研究论文发展脉络
Chat Paper
正在生成论文摘要