Memory-based gradient-guided progressive propagation network for video deblurring
The Visual Computer(2024)
摘要
Video deblurring is a challenging visual task because it requires handling temporal correlations among frames and dealing with various sources of uncertainty in motion blur. To tackle these challenges and effectively capture the spatiotemporal relationships in video sequences, we propose a memory-based gradient-guided progressive propagation network. Our network combines the memory and deblurring branches to restore sharp frames. The memory branch is responsible for storing blurry–sharp feature pairs to provide valuable information for the deblurring process. Within the deblurring branch, we propose two key modules, the progressive feature propagation (PFP) module and the gradient-guided reconstruction (GGR) module , to extract better features that help recover image details. The PFP module is designed to differentiate and align diverse features across different frames. By grouping and progressively propagating these features, the module integrates video information from multiple scales. The GGR module utilizes structural priors present in gradient features to guide the fusion of features. This module leverages the inherent structural information in the gradient features to enhance the recovery of fine details and reduce geometric distortions in the deblurred frames. Experimental results demonstrate that our proposed method achieves competitive results with state-of-the-art methods, while our model is less complex and requires relatively fewer parameters.
更多查看译文
关键词
Video deblurring,Memory network,RNN,Gradient map
AI 理解论文
溯源树
样例
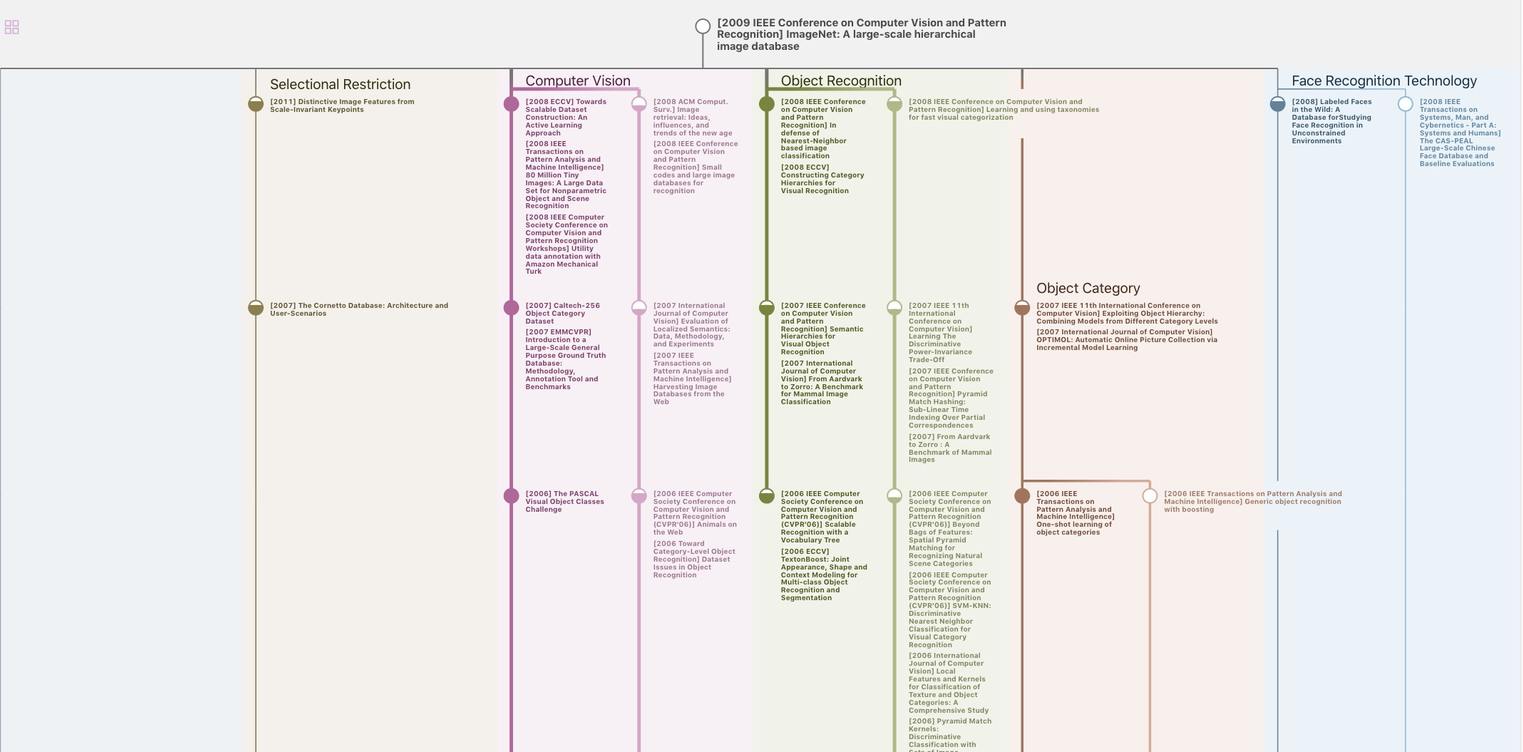
生成溯源树,研究论文发展脉络
Chat Paper
正在生成论文摘要