Two-dimensional temperature field inversion of turbine blade based on physics-informed neural networks
PHYSICS OF FLUIDS(2024)
摘要
Correct evaluation of the blades' surface temperature field is crucial to the structural design and operational safety of aero-engine turbine blades. Current high-temperature measurement technology can only measure the limited discrete points temperature of the hot-end turbine blades. If the boundary conditions are completely unknown, it is still difficult to solve equations through traditional computational fluid dynamics methods. It is an inverse problem to predict the temperature field of the turbine engine blade with unknown boundary conditions. This paper proposes a two-dimensional temperature field inversion of turbine blades based on physics-informed neural networks (PINN) and finite discrete temperature measurement points. The PINN is used to model the nonlinear mapping of input variables and output variables. Only a small amount of data is used to train the neural network. It can be found that adding the loss term of the physical governing equation during training can make the neural network better predict the temperature field of the turbine blade, which can also avoid anomalies such as large temperature errors in some areas that may occur when training with only a small amount of data. When the boundary conditions are completely unknown, the average relative error of PINN trained with only 62 points of temperature data on the test set is below 2%, and the R-2 - Score is above 0.95. When the training data reaches 122 points of temperature data, the average relative error of PINN on the test set is less than 1%, and the R-2 - Score reaches 0.99.
更多查看译文
AI 理解论文
溯源树
样例
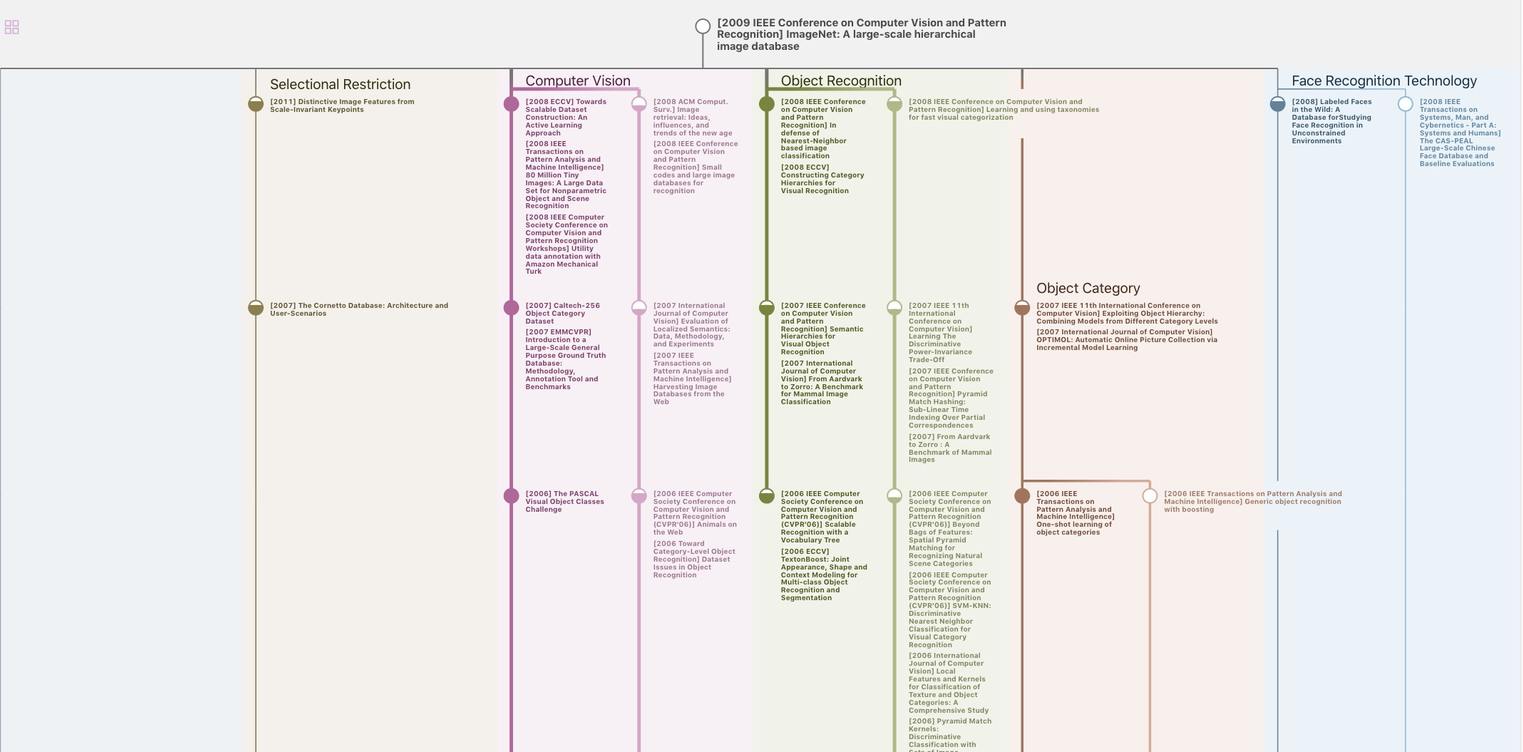
生成溯源树,研究论文发展脉络
Chat Paper
正在生成论文摘要