Estimators of various kappa coefficients based on the unbiased estimator of the expected index of agreements
Advances in Data Analysis and Classification(2024)
摘要
To measure the degree of agreement between R observers who independently classify n subjects within K categories, various kappa-type coefficients are often used. When R = 2, it is common to use the Cohen' kappa, Scott's pi, Gwet’s AC1/2, and Krippendorf's alpha coefficients (weighted or not). When R > 2, some pairwise version based on the aforementioned coefficients is normally used; with the same order as above: Hubert's kappa, Fleiss's kappa, Gwet's AC1/2, and Krippendorf's alpha. However, all these statistics are based on biased estimators of the expected index of agreements, since they estimate the product of two population proportions through the product of their sample estimators. The aims of this article are three. First, to provide statistics based on unbiased estimators of the expected index of agreements and determine their variance based on the variance of the original statistic. Second, to make pairwise extensions of some measures. And third, to show that the old and new estimators of the Cohen’s kappa and Hubert’s kappa coefficients match the well-known estimators of concordance and intraclass correlation coefficients, if the former are defined by assuming quadratic weights. The article shows that the new estimators are always greater than or equal the classic ones, except for the case of Gwet where it is the other way around, although these differences are only relevant with small sample sizes (e.g. n ≤ 30).
更多查看译文
关键词
Agreement,Cohen’s kappa,Concordance and intraclass correlation coefficients,Conger’s kappa,Fleiss’ kappa,Gwet's AC1/2,Hubert’s kappa,Krippendorf's alpha,Pairwise multi-rater kappa,Scott's pi,62F10,62F12,62H99,62P15
AI 理解论文
溯源树
样例
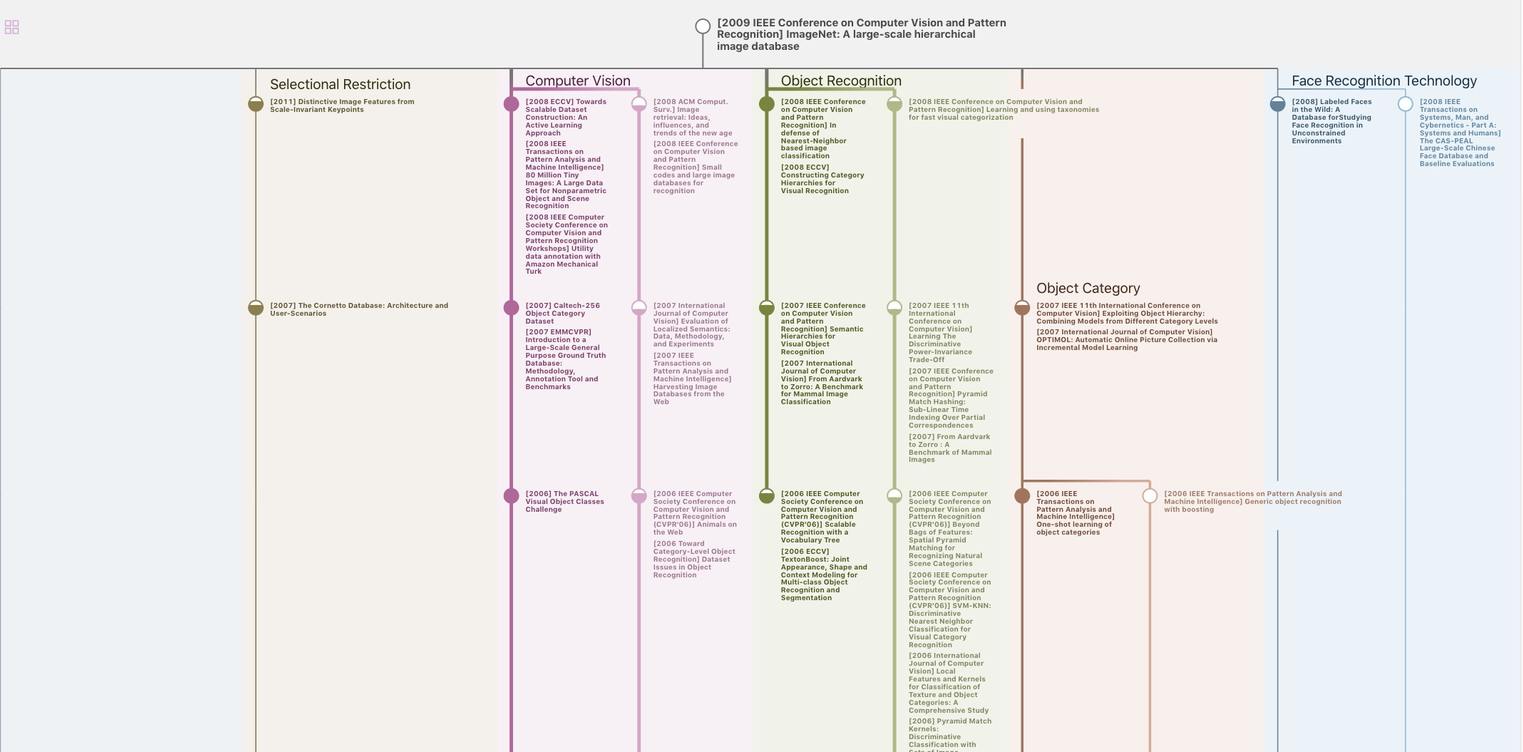
生成溯源树,研究论文发展脉络
Chat Paper
正在生成论文摘要