Enhanced SARS-CoV-2 case prediction using public health data and machine learning models
JAMIA OPEN(2024)
摘要
Objectives The goal of this study is to propose and test a scalable framework for machine learning (ML) algorithms to predict near-term severe acute respiratory syndrome coronavirus 2 (SARS-CoV-2) cases by incorporating and evaluating the impact of real-time dynamic public health data.Materials and Methods Data used in this study include patient-level results, procurement, and location information of all SARS-CoV-2 tests reported in West Virginia as part of their mandatory reporting system from January 2021 to March 2022. We propose a method for incorporating and comparing widely available public health metrics inside of a ML framework, specifically a long-short-term memory network, to forecast SARS-CoV-2 cases across various feature sets.Results Our approach provides better prediction of localized case counts and indicates the impact of the dynamic elements of the pandemic on predictions, such as the influence of the mixture of viral variants in the population and variable testing and vaccination rates during various eras of the pandemic.Discussion Utilizing real-time public health metrics, including estimated Rt from multiple SARS-CoV-2 variants, vaccination rates, and testing information, provided a significant increase in the accuracy of the model during the Omicron and Delta period, thus providing more precise forecasting of daily case counts at the county level. This work provides insights on the influence of various features on predictive performance in rural and non-rural areas.Conclusion Our proposed framework incorporates available public health metrics with operational data on the impact of testing, vaccination, and current viral variant mixtures in the population to provide a foundation for combining dynamic public health metrics and ML models to deliver forecasting and insights in healthcare domains. It also shows the importance of developing and deploying ML frameworks in rural settings. This study aims to propose and test a scalable framework for machine learning (ML) algorithms to predict near-term severe acute respiratory syndrome coronavirus 2 (SARS-CoV-2) cases by county by incorporating and evaluating the impact of real-time dynamic public health data. Data used in this study include patient-level results, procurement, and location information of all SARS-CoV-2 tests reported in West Virginia as part of their mandatory reporting system from January 2021 to March 2022. We propose a method for incorporating and comparing widely available public health metrics inside of a ML framework, specifically a long-short-term memory network, to forecast SARS-CoV-2 cases across various feature sets. Our approach provides better prediction of localized case counts, recommendation of locations of outbreaks, and indicates the impact of the dynamic elements of the pandemic on predictions, such as the influence of the mixture of viral variants in the population and variable testing and vaccination rates during various eras of the pandemic. Incorporating available public health metrics with operational data on the impact of testing, vaccination, and current viral variant mixtures in the population provides a foundation for combining dynamic public health metrics and ML models to deliver improved forecasting and insights in healthcare domains. This approach provides a model for utilizing ML to forecast, deploy, and understand the impact of public health data during coronavirus disease and other pandemics.
更多查看译文
关键词
public health data,machine learning,SARS-CoV-2 prediction
AI 理解论文
溯源树
样例
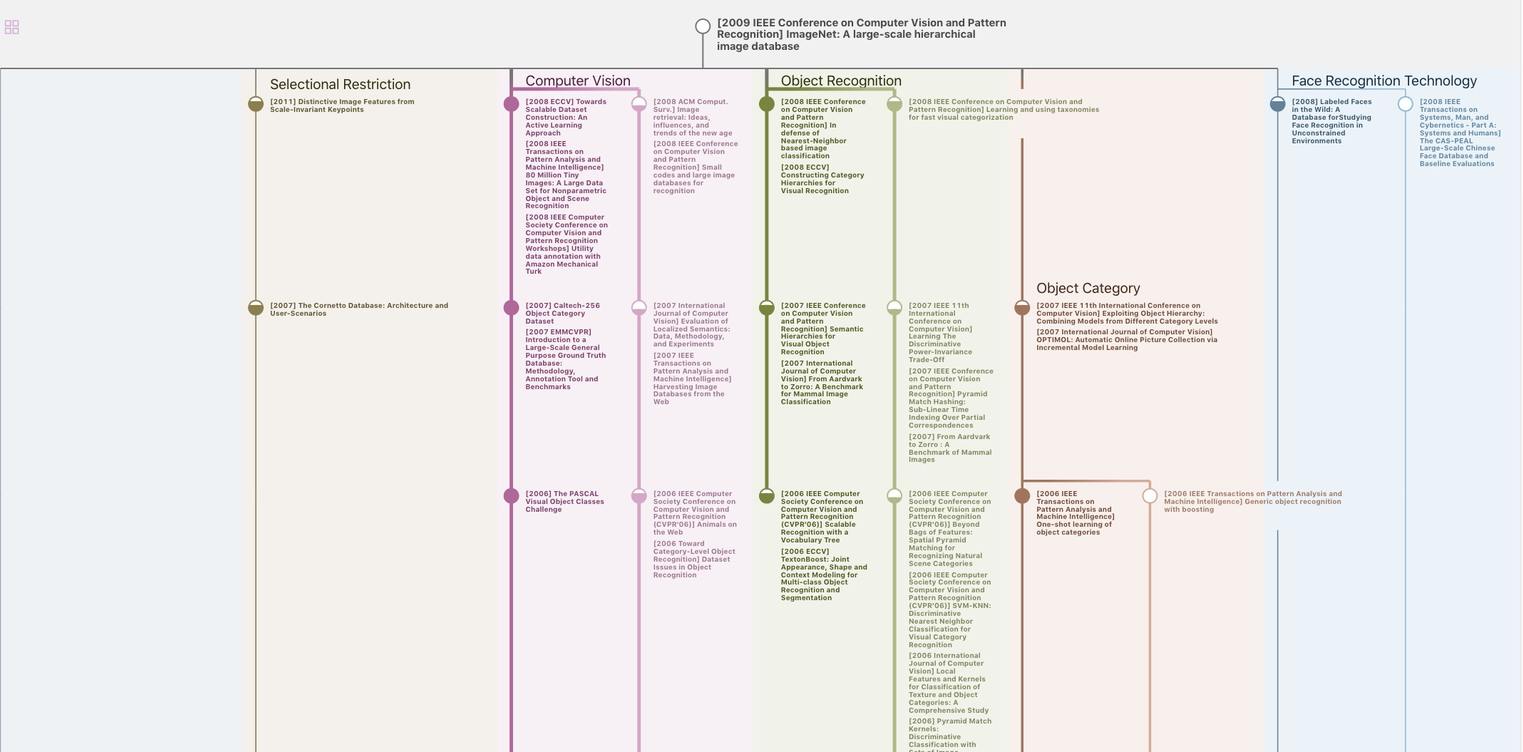
生成溯源树,研究论文发展脉络
Chat Paper
正在生成论文摘要