A Shrinkage Approach to Improve Direct Bootstrap Resampling Under Input Uncertainty
INFORMS JOURNAL ON COMPUTING(2024)
摘要
Discrete -event simulation models generate random variates from input distributions and compute outputs according to the simulation logic. The input distributions are typically fitted to finite real -world data and thus are subject to estimation errors that can propagate to the simulation outputs: an issue commonly known as input uncertainty (IU). This paper investigates quantifying IU using the output confidence intervals (CIs) computed from bootstrap quantile estimators. The standard direct bootstrap method has overcoverage due to convolution of the simulation error and IU; however, the brute -force way of washing away the former is computationally demanding. We present two new bootstrap methods to enhance direct resampling in both statistical and computational efficiencies using shrinkage strategies to down -scale the variabilities encapsulated in the CIs. Our asymptotic analysis shows how both approaches produce tight CIs accounting for IU under limited input data and simulation effort along with the simulation sample -size requirements relative to the input data size. We demonstrate performances of the shrinkage strategies with several numerical experiments and investigate the conditions under which each method performs well. We also show advantages of nonparametric approaches over parametric bootstrap when the distribution family is misspecified and over metamodel approaches when the dimension of the distribution parameters is high.
更多查看译文
关键词
bootstrap resampling,input uncertainty,nonparametric,simulation,shrinkage
AI 理解论文
溯源树
样例
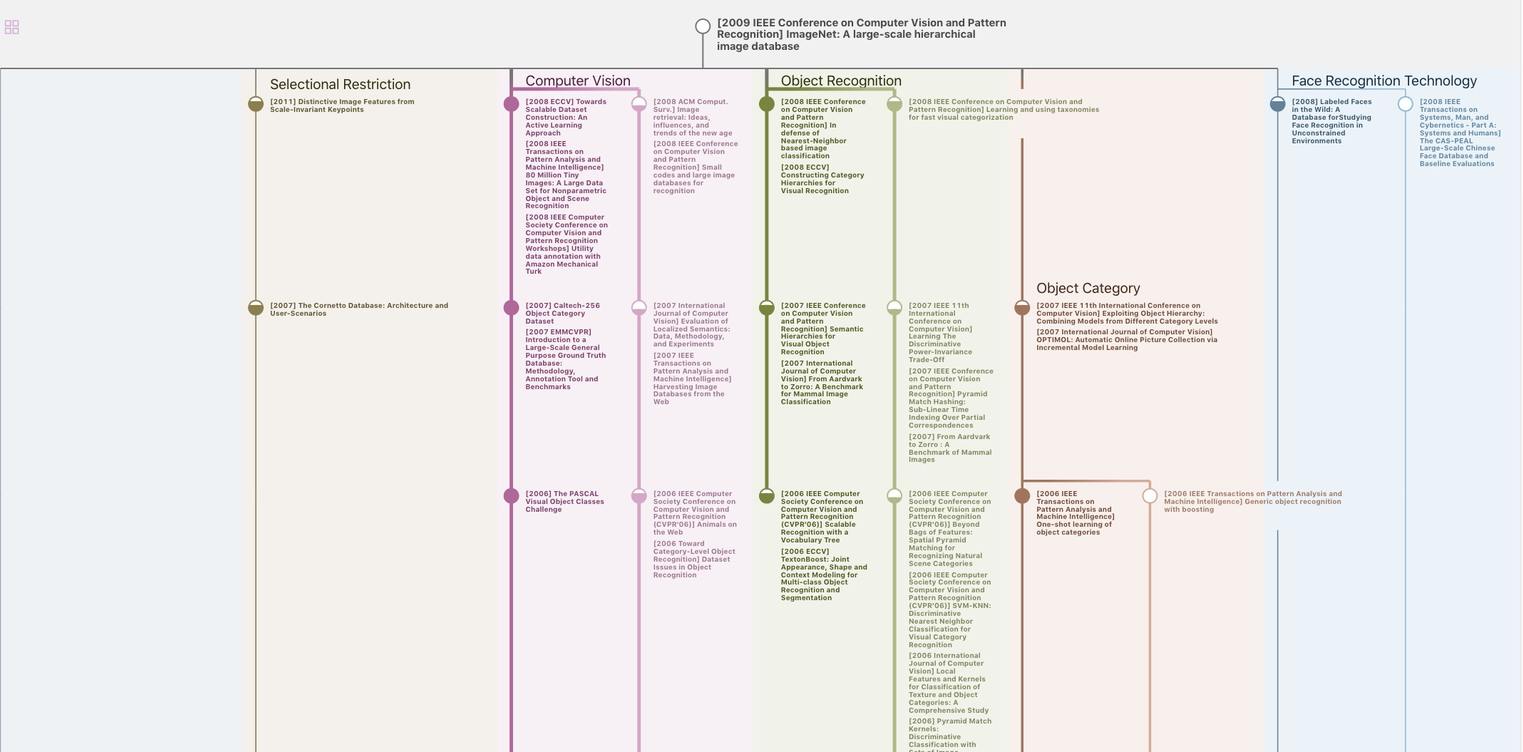
生成溯源树,研究论文发展脉络
Chat Paper
正在生成论文摘要