Efficient and effective (k, P)-core-based community search over attributed heterogeneous information networks.
Inf. Sci.(2024)
摘要
Given a heterogeneous information network (HIN) G and a query node q , community search (CS) over an HIN identifies a cohesive subgraph from G that contains q . Although HINs with attributes on nodes (called AHINs) are prevalent today, the CS over AHINs (CS-AHIN) is ignored in the literature. Though we can convert an AHIN to an attributed homogeneous graph given a meta-path, then apply the CS approaches for attributed homogeneous graphs to solve CS-AHIN, it is problematic for two reasons. (1) Complete graph conversion is time-consuming and unnecessary, because the search only involves the query node's neighborhood, not the entire graph. (2) Existing attribute cohesiveness metrics are not strict enough to reflect substantial similarities among the community's pairwise nodes. To resolve this, we define the CS-AHIN problem atop a strict attribute cohesiveness metric that supports textual and numerical attributes simultaneously. We show the problem is NP-hard. To address it, we propose an exact baseline to return the global optimal result. Then, we propose three heuristic algorithms using a general greedy search framework to speed up the efficiency. Moreover, we present a cohesiveness-aware proximity graph-based index to boost the performance. Comprehensive experimental studies on various real-world datasets demonstrate our method's superiority. • We define a CS-AHIN problem based on ( k , P )-core model, which is NP-hard. • We present a general greedy search framework with three efficient heuristic methods. • We improve heuristic methods via a cohesiveness-aware index atop proximity graph. • Extensive experiments show our method's superiority in effectiveness and efficiency.
更多查看译文
关键词
Community search,Heterogeneous information network,( k,P )-core,Proximity graph
AI 理解论文
溯源树
样例
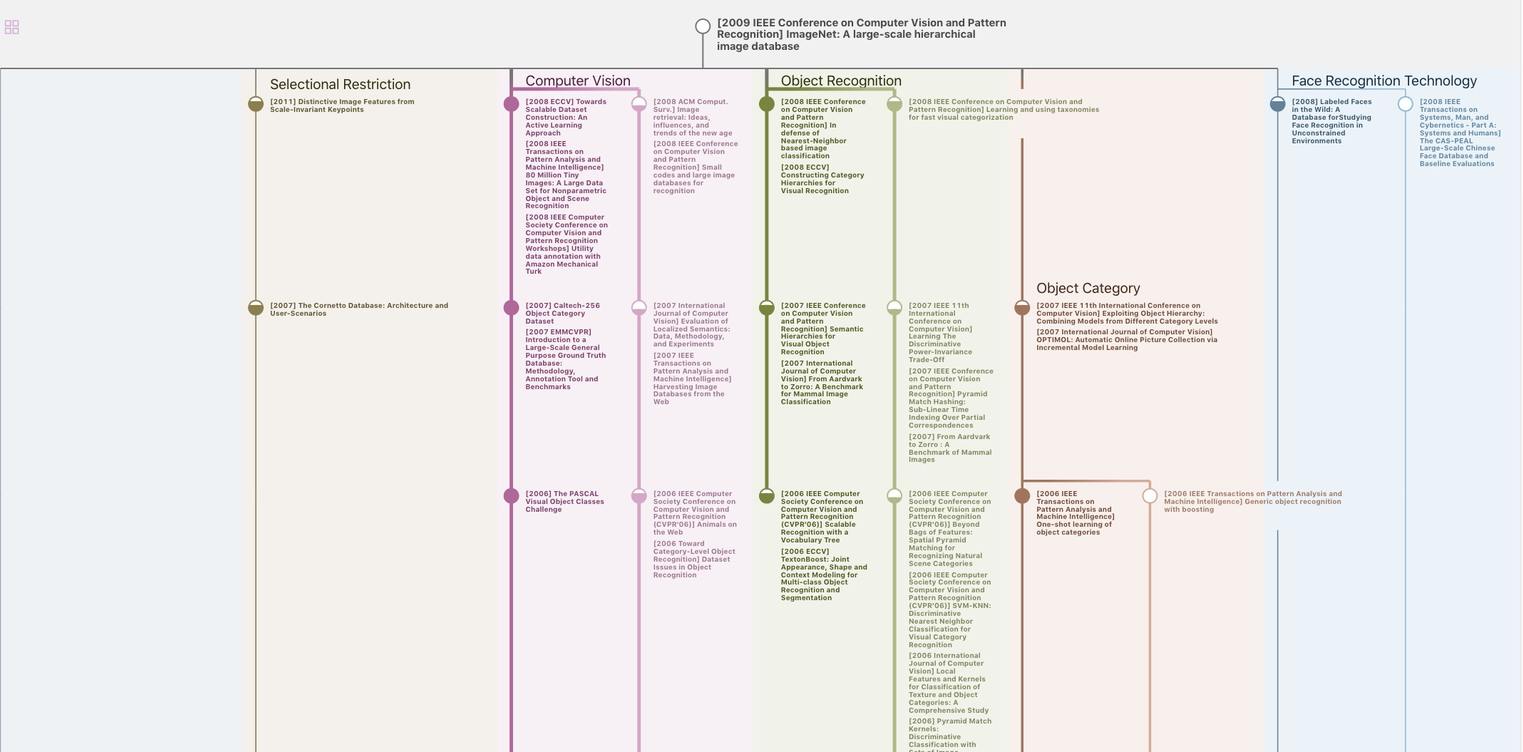
生成溯源树,研究论文发展脉络
Chat Paper
正在生成论文摘要