Differentiation of COVID-19 pneumonia from other lung diseases using CT radiomic features and machine learning: A large multicentric cohort study
INTERNATIONAL JOURNAL OF IMAGING SYSTEMS AND TECHNOLOGY(2024)
摘要
To derive and validate an effective machine learning and radiomics-based model to differentiate COVID-19 pneumonia from other lung diseases using a large multi-centric dataset. In this retrospective study, we collected 19 private and five public datasets of chest CT images, accumulating to 26 307 images (15 148 COVID-19; 9657 other lung diseases including non-COVID-19 pneumonia, lung cancer, pulmonary embolism; 1502 normal cases). We tested 96 machine learning-based models by cross-combining four feature selectors (FSs) and eight dimensionality reduction techniques with eight classifiers. We trained and evaluated our models using three different strategies: #1, the whole dataset (15 148 COVID-19 and 11 159 other); #2, a new dataset after excluding healthy individuals and COVID-19 patients who did not have RT-PCR results (12 419 COVID-19 and 8278 other); and #3 only non-COVID-19 pneumonia patients and a random sample of COVID-19 patients (3000 COVID-19 and 2582 others) to provide balanced classes. The best models were chosen by one-standard-deviation rule in 10-fold cross-validation and evaluated on the hold out test sets for reporting. In strategy#1, Relief FS combined with random forest (RF) classifier resulted in the highest performance (accuracy = 0.96, AUC = 0.99, sensitivity = 0.98, specificity = 0.94, PPV = 0.96, and NPV = 0.96). In strategy#2, Recursive Feature Elimination (RFE) FS and RF classifier combination resulted in the highest performance (accuracy = 0.97, AUC = 0.99, sensitivity = 0.98, specificity = 0.95, PPV = 0.96, NPV = 0.98). Finally, in strategy #3, the ANOVA FS and RF classifier combination resulted in the highest performance (accuracy = 0.94, AUC =0.98, sensitivity = 0.96, specificity = 0.93, PPV = 0.93, NPV = 0.96). Lung radiomic features combined with machine learning algorithms can enable the effective diagnosis of COVID-19 pneumonia in CT images without the use of additional tests.
更多查看译文
关键词
computed tomography,COVID-19,differential diagnosis,machine learning,radiomics
AI 理解论文
溯源树
样例
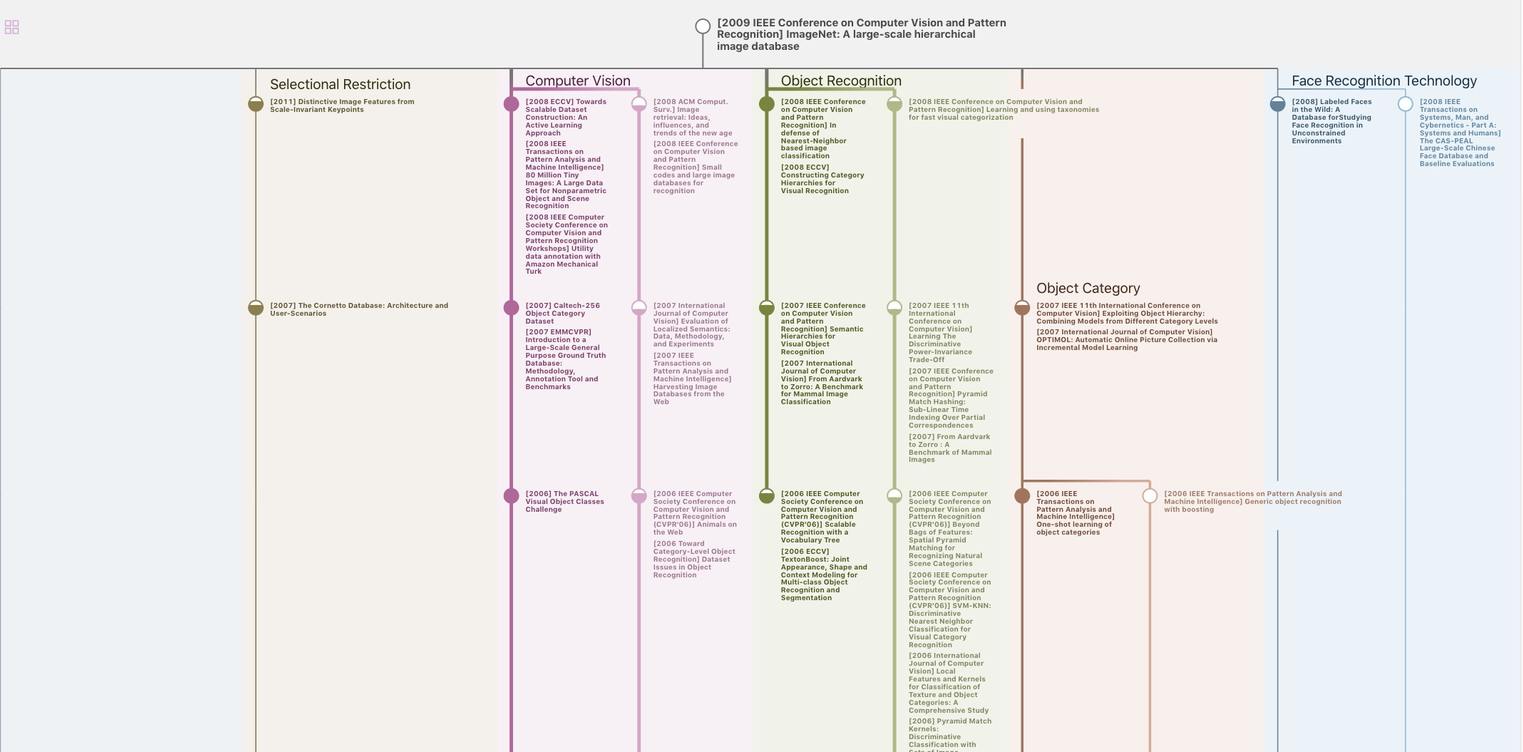
生成溯源树,研究论文发展脉络
Chat Paper
正在生成论文摘要