MiM-ISTD: Mamba-in-Mamba for Efficient Infrared Small Target Detection
arxiv(2024)
摘要
Thanks to the development of basic models, infrared small target detection
(ISTD) algorithms have made significant progress. Specifically, the structures
combining convolutional networks with transformers can well extract both local
and global features. At the same time, they also inherit defects from the basic
model, e.g., the quadratic computational complexity of transformers, which
impacts efficiency. Inspired by a recent basic model with linear complexity for
long-distance modeling, called Mamba, we explore the potential of this state
space model in ISTD in this paper. However, direct application is unsuitable
since local features, which are critical to detecting small targets, cannot be
fully exploited. Instead, we tailor a Mamba-in-Mamba (MiM-ISTD) structure for
efficient ISTD. For example, we treat the local patches as "visual sentences"
and further decompose them into sub-patches as "visual words" to further
explore the locality. The interactions among each word in a given visual
sentence will be calculated with negligible computational costs. By aggregating
the word and sentence features, the representation ability of MiM-ISTD can be
significantly bolstered. Experiments on NUAA-SIRST and IRSTD-1k prove the
superior accuracy and efficiency of our method. Specifically, MiM-ISTD is 10
× faster than the SOTA and reduces GPU memory usage by 73.4% per 2048
× 2048 image during inference, overcoming the computation&memory
constraints on performing Mamba-based understanding on high-resolution infrared
images.Source code is available at https://github.com/txchen-USTC/MiM-ISTD.
更多查看译文
AI 理解论文
溯源树
样例
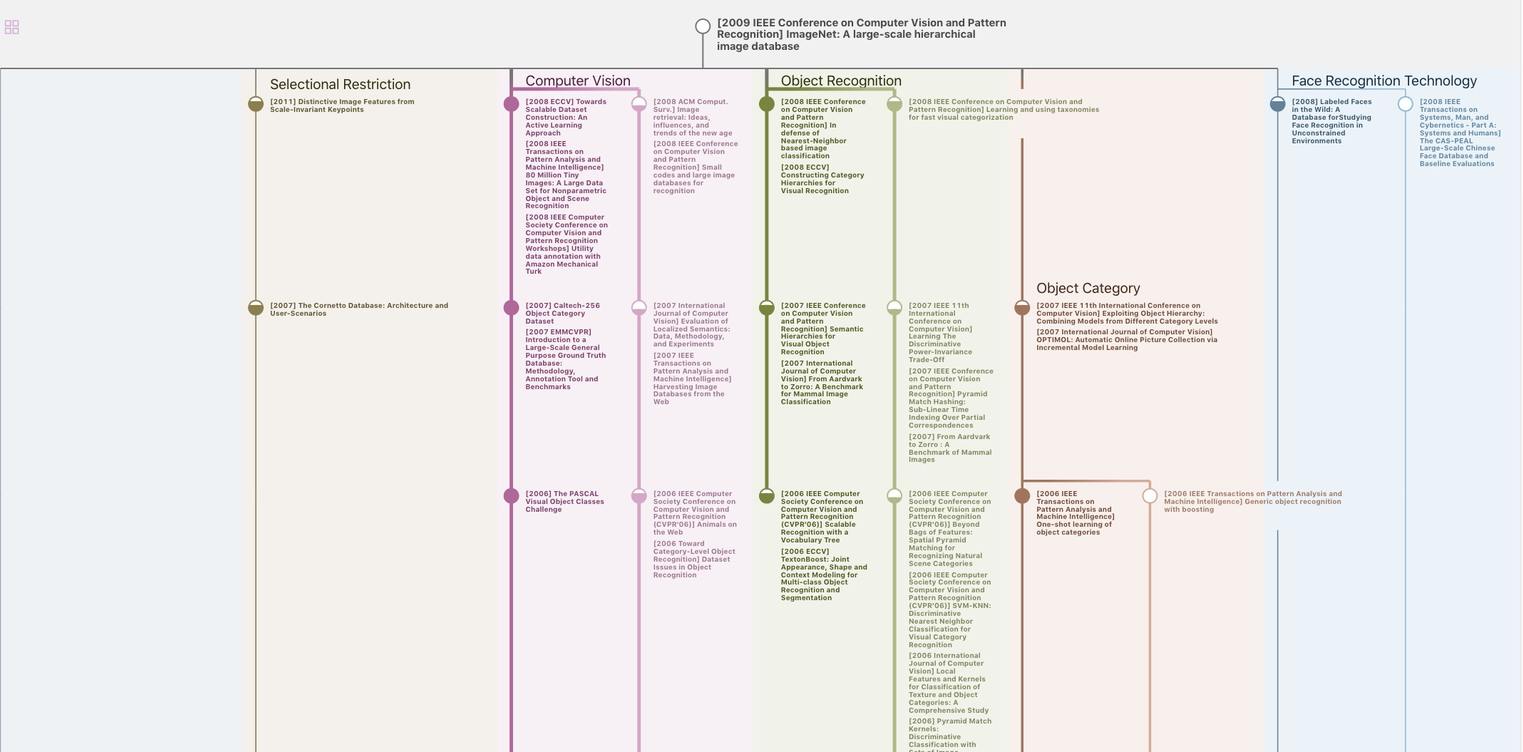
生成溯源树,研究论文发展脉络
Chat Paper
正在生成论文摘要