Self-Supervised Representation Learning with Meta Comprehensive Regularization
AAAI 2024(2024)
摘要
Self-Supervised Learning (SSL) methods harness the concept of semantic invariance by utilizing data augmentation strategies to produce similar representations for different deformations of the same input. Essentially, the model captures the shared information among multiple augmented views of samples, while disregarding the non-shared information that may be beneficial for downstream tasks. To address this issue, we introduce a module called CompMod with Meta Comprehensive Regularization (MCR), embedded into existing self-supervised frameworks, to make the learned representations more comprehensive. Specifically, we update our proposed model through a bi-level optimization mechanism, enabling it to capture comprehensive features. Additionally, guided by the constrained extraction of features using maximum entropy coding, the self-supervised learning model learns more comprehensive features on top of learning consistent features. In addition, we provide theoretical support for our proposed method from information theory and causal counterfactual perspective. Experimental results show that our method achieves significant improvement in classification, object detection and semantic segmentation tasks on multiple benchmark datasets.
更多查看译文
关键词
CV: Representation Learning for Vision,CV: Learning & Optimization for CV,ML: Classification and Regression,ML: Representation Learning
AI 理解论文
溯源树
样例
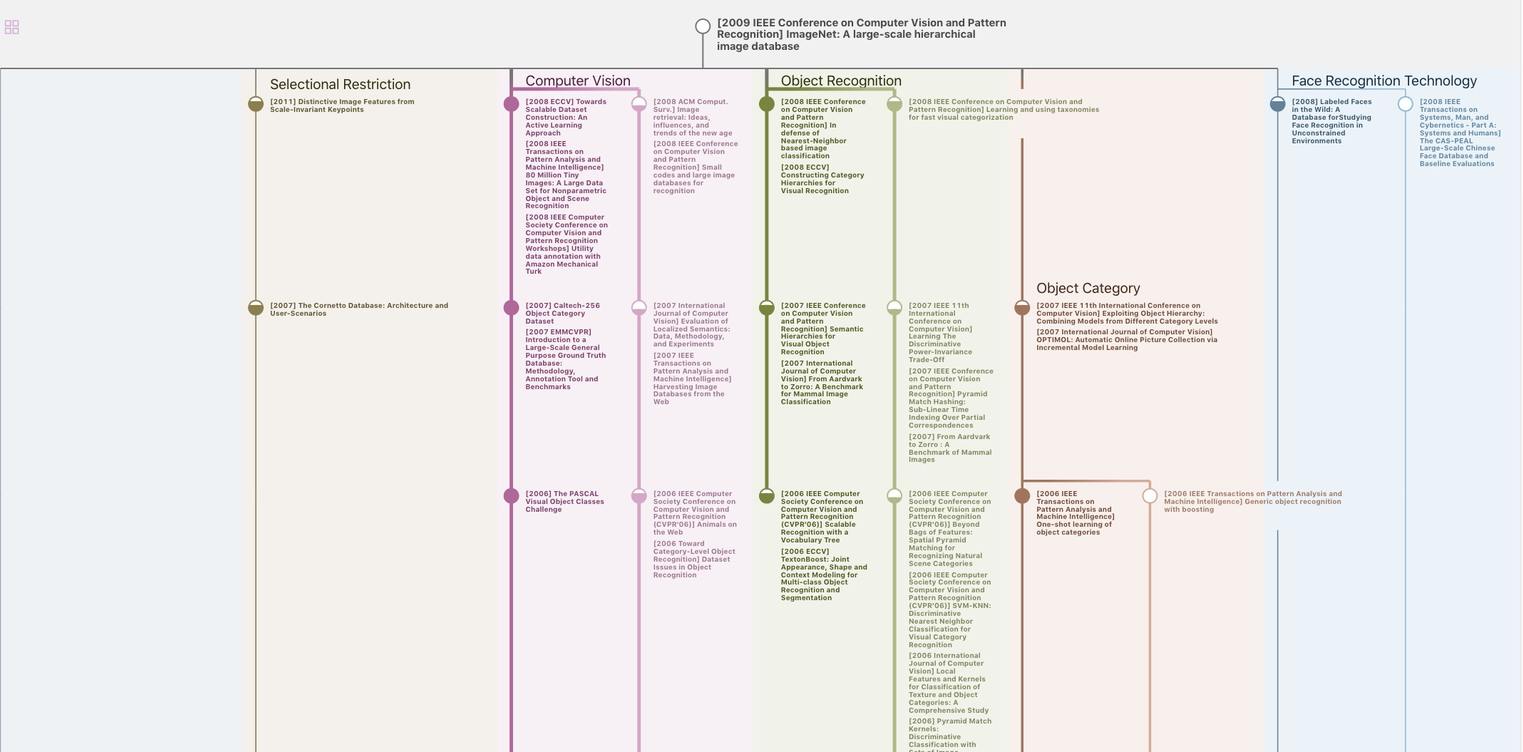
生成溯源树,研究论文发展脉络
Chat Paper
正在生成论文摘要