SLICK: Strong Lensing Identification of Candidates Kindred in gravitational wave data
arxiv(2024)
摘要
By the end of the next decade, we hope to have detected strongly lensed
gravitational waves by galaxies or clusters. Although there exist optimal
methods for identifying lensed signal, it is shown that machine learning (ML)
algorithms can give comparable performance but are orders of magnitude faster
than non-ML methods. We present the SLICK pipeline which comprises a parallel
network based on deep learning. We analyse the Q-transform maps (QT maps) and
the Sine-Gaussian maps (SGP-maps) generated for the binary black hole signals
injected in Gaussian as well as real noise. We compare our network performance
with the previous work and find that the efficiency of our model is higher by a
factor of 5 at a false positive rate of 0.001. Further, we show that including
SGP maps with QT maps data results in a better performance than analysing QT
maps alone. When combined with sky localisation constraints, we hope to get
unprecedented accuracy in the predictions than previously possible. We also
evaluate our model on the real events detected by the LIGO–Virgo collaboration
and find that our network correctly classifies all of them, consistent with
non-detection of lensing.
更多查看译文
AI 理解论文
溯源树
样例
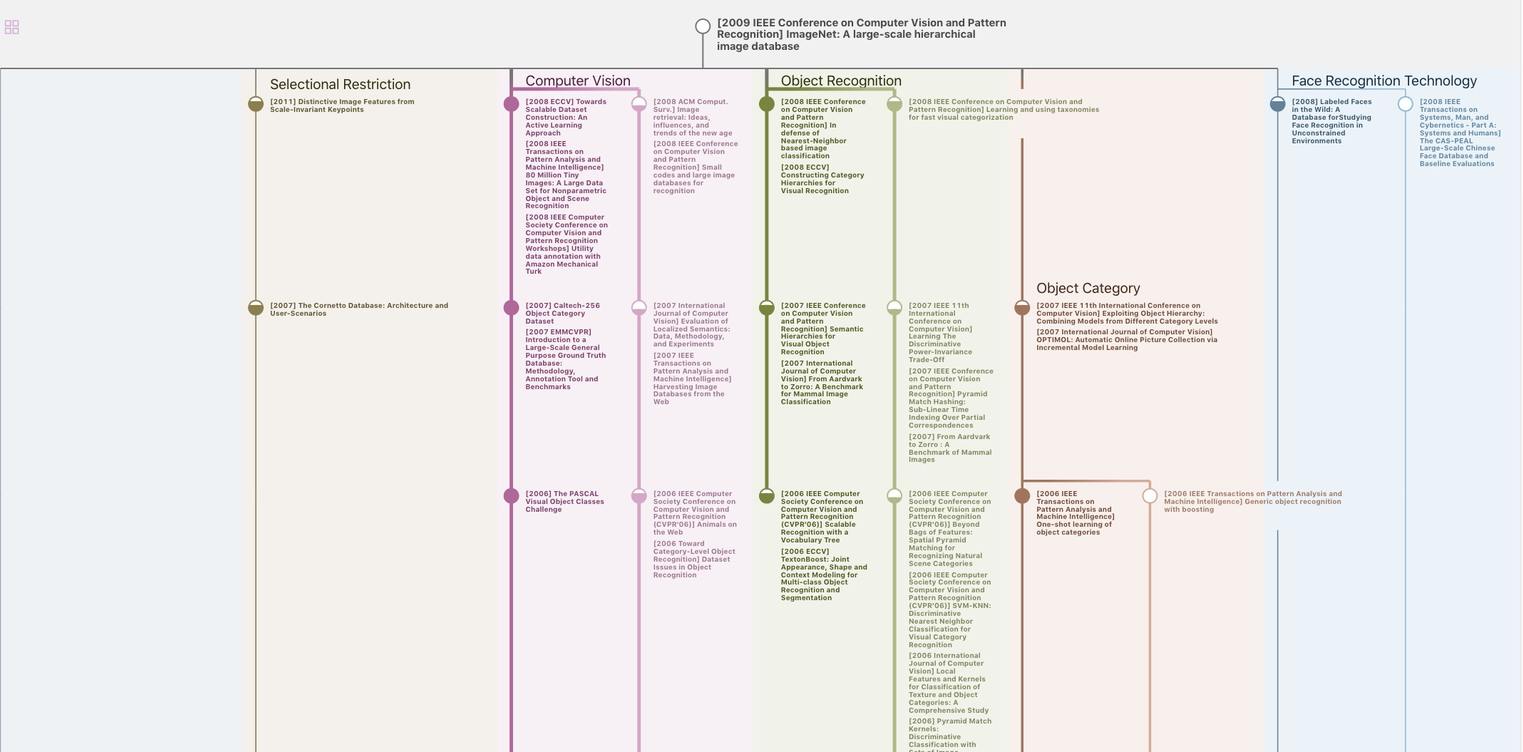
生成溯源树,研究论文发展脉络
Chat Paper
正在生成论文摘要