SA-SOT: Speaker-Aware Serialized Output Training for Multi-Talker ASR
ICASSP 2024 - 2024 IEEE International Conference on Acoustics, Speech and Signal Processing (ICASSP)(2024)
摘要
Multi-talker automatic speech recognition plays a crucial role in scenarios
involving multi-party interactions, such as meetings and conversations. Due to
its inherent complexity, this task has been receiving increasing attention.
Notably, the serialized output training (SOT) stands out among various
approaches because of its simplistic architecture and exceptional performance.
However, the frequent speaker changes in token-level SOT (t-SOT) present
challenges for the autoregressive decoder in effectively utilizing context to
predict output sequences. To address this issue, we introduce a masked t-SOT
label, which serves as the cornerstone of an auxiliary training loss.
Additionally, we utilize a speaker similarity matrix to refine the
self-attention mechanism of the decoder. This strategic adjustment enhances
contextual relationships within the same speaker's tokens while minimizing
interactions between different speakers' tokens. We denote our method as
speaker-aware SOT (SA-SOT). Experiments on the Librispeech datasets demonstrate
that our SA-SOT obtains a relative cpWER reduction ranging from 12.75
22.03
training, our method achieves an impressive cpWER of 3.41
state-of-the-art result on the LibrispeechMix dataset.
更多查看译文
关键词
Multi-talker,automatic speech recognition,speaker-aware,serialized output training
AI 理解论文
溯源树
样例
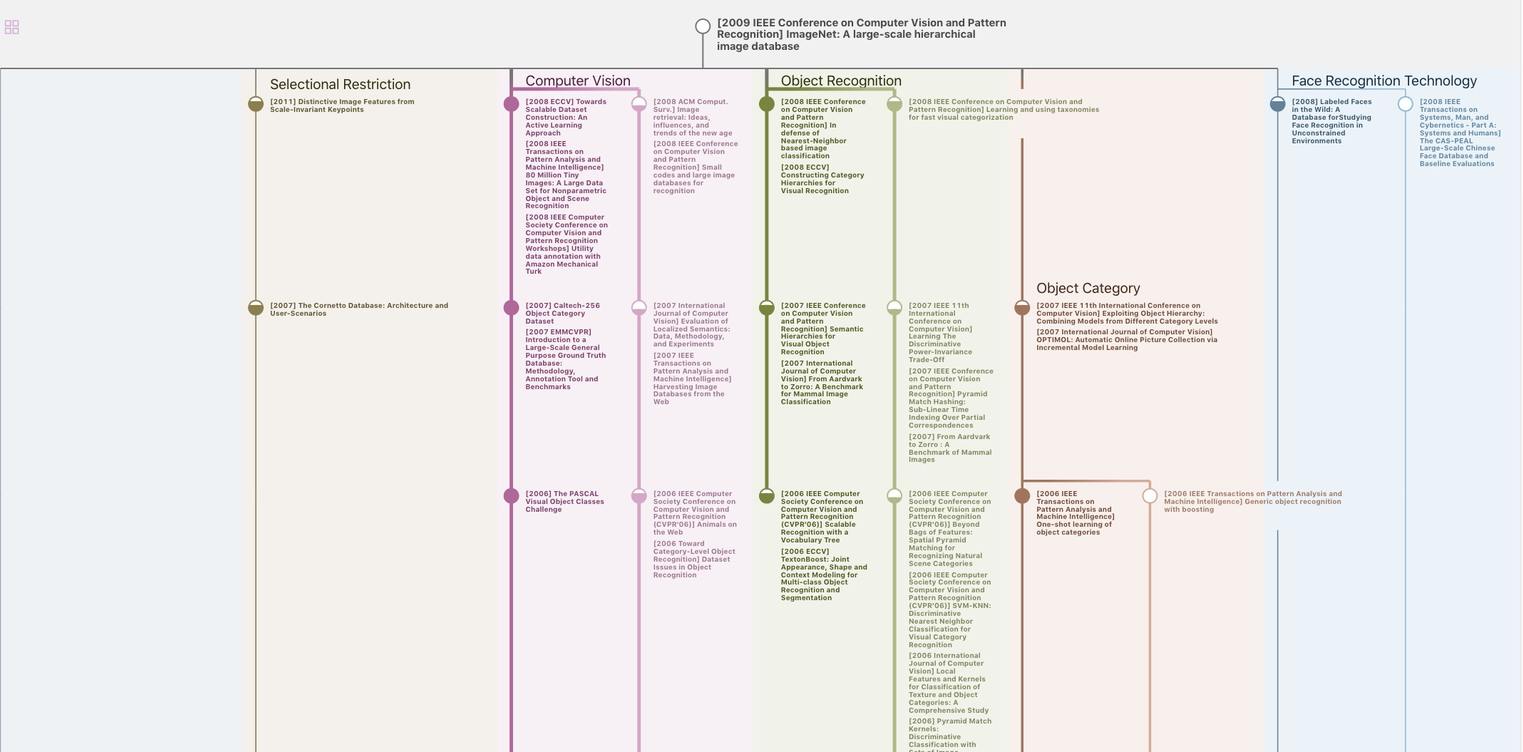
生成溯源树,研究论文发展脉络
Chat Paper
正在生成论文摘要