A Machine Learning Approach to Forecast 5G Data in a Commercial and Operational 5G Platform.
Wireless on Demand Network Systems and Service(2024)
摘要
The demand for more secure, available, reliable, and fast networks emerges in a more interconnected society. In this context, 5G networks aim to revolutionize how we communicate and interact. However, studies using 5G data are sparse since there are only a few number of publicly available 5G datasets (especially about commercial 5G network metrics with real users). In this work, we analyze the data of a commercial 5G deployment with real users, and propose forecasting techniques to help understand the trends and to manage 5G networks. We propose the creation of a metric to measure the traffic load. We forecast the metric using several machine learning models, and we choose LightGBM as the best approach. We observe that this approach obtains results with a good accuracy, and better than other machine learning approaches, but its performance decreases if the patterns contain unexpected events. Taking advantage of the lower accuracy in the performance, this is used to detect changes in the patterns and manage the network in real-time, supporting network resource elasticity by generating alarms and automating the scaling during these unpredictable fluctuations.
更多查看译文
关键词
SG Networks,NWDAF,Dimensionality Reduction,PCA,Forecasting,LightGBM
AI 理解论文
溯源树
样例
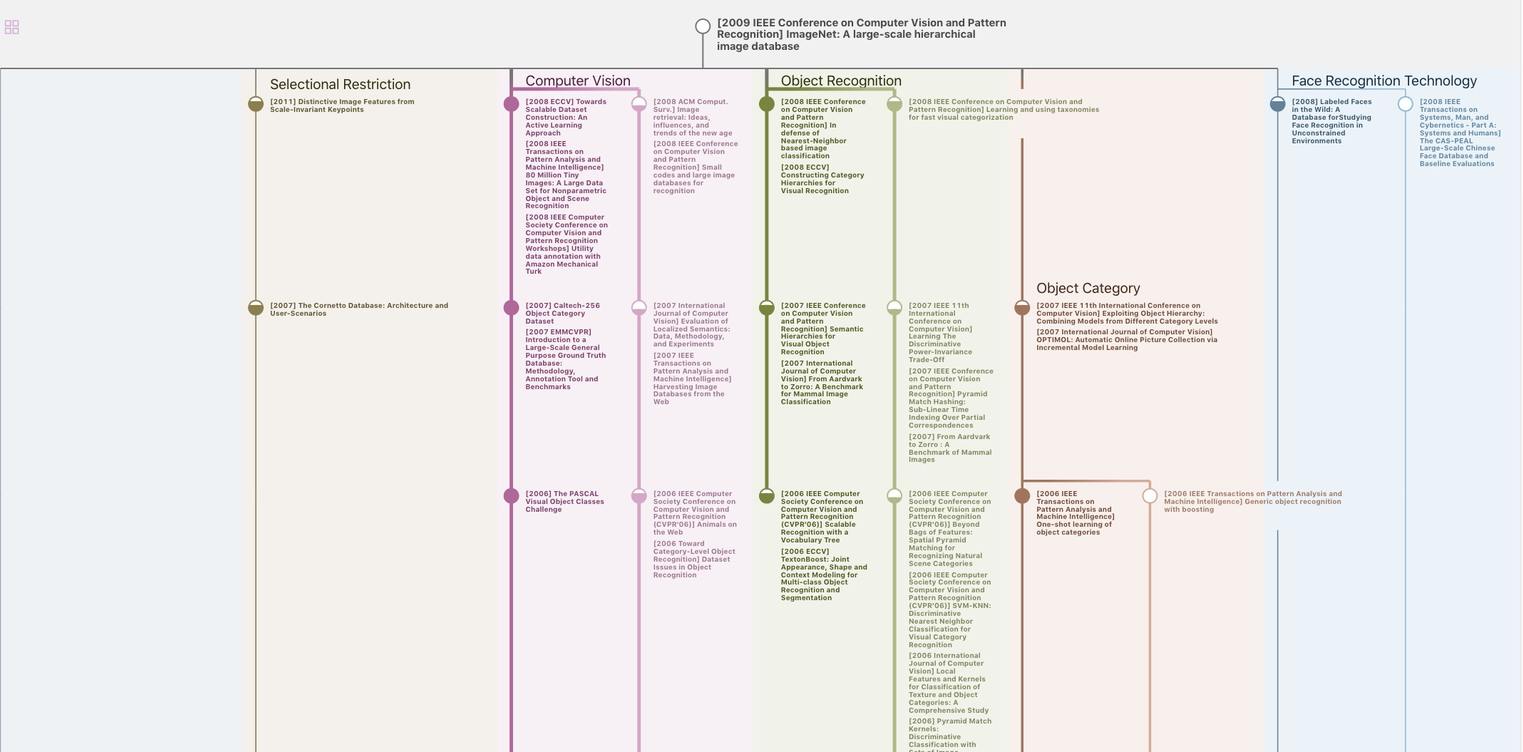
生成溯源树,研究论文发展脉络
Chat Paper
正在生成论文摘要