Improving potato AGB estimation to mitigate phenological stage impacts through depth features from hyperspectral data
COMPUTERS AND ELECTRONICS IN AGRICULTURE(2024)
摘要
The accurate estimation of above ground biomass (AGB) is valuable in grasping the status of potato growth and assessing yield. Spectral analysis techniques play an important role in AGB estimation by virtue of its nondestructive and rapid advantages. However, the models constructed based on spectral features at the entire growth stages due to differences in phenological stages, such as vegetation indices (VIs) and locations at fixed wavelengths, can be not applicable to single growth stages. Therefore, the main objective of this study is to improve the applicability of the AGB estimation model to mitigate the impacts of phenological stages by mining depth features from hyperspectral data. The canopy hyperspectral and AGB data of potato tuber formation, tuber growth and starch accumulation stages were measured in 2017-2019. The acquired canopy hyperspectral reflectance was smoothed by Savitzky-Golay (SG) filter, and the green edge (GE), the red edge (RE), red edge chlorophyll index (CIred-edge), optimized soil adjusted vegetation index (OSAVI), green normalized difference vegetation index (GNDVI), and normalized difference vegetation index (NDVI) were extracted. The spectral changes were analyzed at different growth stages of each year. To obtain features in response to AGB changes, a new approach is proposed to analyze the hidden depth features between sensitive wavelengths by combining a successive projections algorithm (SPA) and a long-short-term memory network (LSTM). The AGB estimation models were constructed by partial least squares regression (PLSR) based on traditional VIs + GE + RE, full spectrum, sensitive wavelengths obtained by SPA, and depth features obtained by SPA-LSTM. The results showed that GE, RE and VIs alone explained only 24 %-36 % of the variation in AGB. The accuracy of estimating AGB at sensitive wavelengths obtained after SPA was higher (R2 = 0.70, RMSE = 406 kg/hm2, and NRMSE = 27.14 %) than that of conventional VIs + GE + RE (R2 = 0.51, RMSE = 536 kg/hm2, and NRMSE = 35.81 %) and full spectrum (R2 = 0.63, RMSE = 452 kg/hm2, and NRMSE = 30.19 %) in the three-year dataset. The depth features extracted by the SPA-LSTM method proposed in this study achieved the best AGB estimation (R2 = 0.82, RMSE = 318 kg/hm2, and NRMSE = 21.25 %). The applicability of the model was validated in different years and growth stages. The R2 of each year was in the range of 0.48-0.77, the RMSE was in the range of 290-367 kg/hm2, and the NRMSE was in the range of 16.69 %-25.81 %. The R2 of each growth stage within three year was in the range of 0.48-0.78, the RMSE was in the range of 184-386 kg/hm2, and the NRMSE was in the range of 14.91 %-29.45 %. The method proposed in this study mitigates the impacts of phenological stage and improves the robustness and accuracy of the AGB estimation model, which provides remote sensing technical support for realizing potato growth monitoring and yield assessment in the field.
更多查看译文
关键词
AGB,Hyperspectral features,Deep features,SPA,LSTM,PLSR
AI 理解论文
溯源树
样例
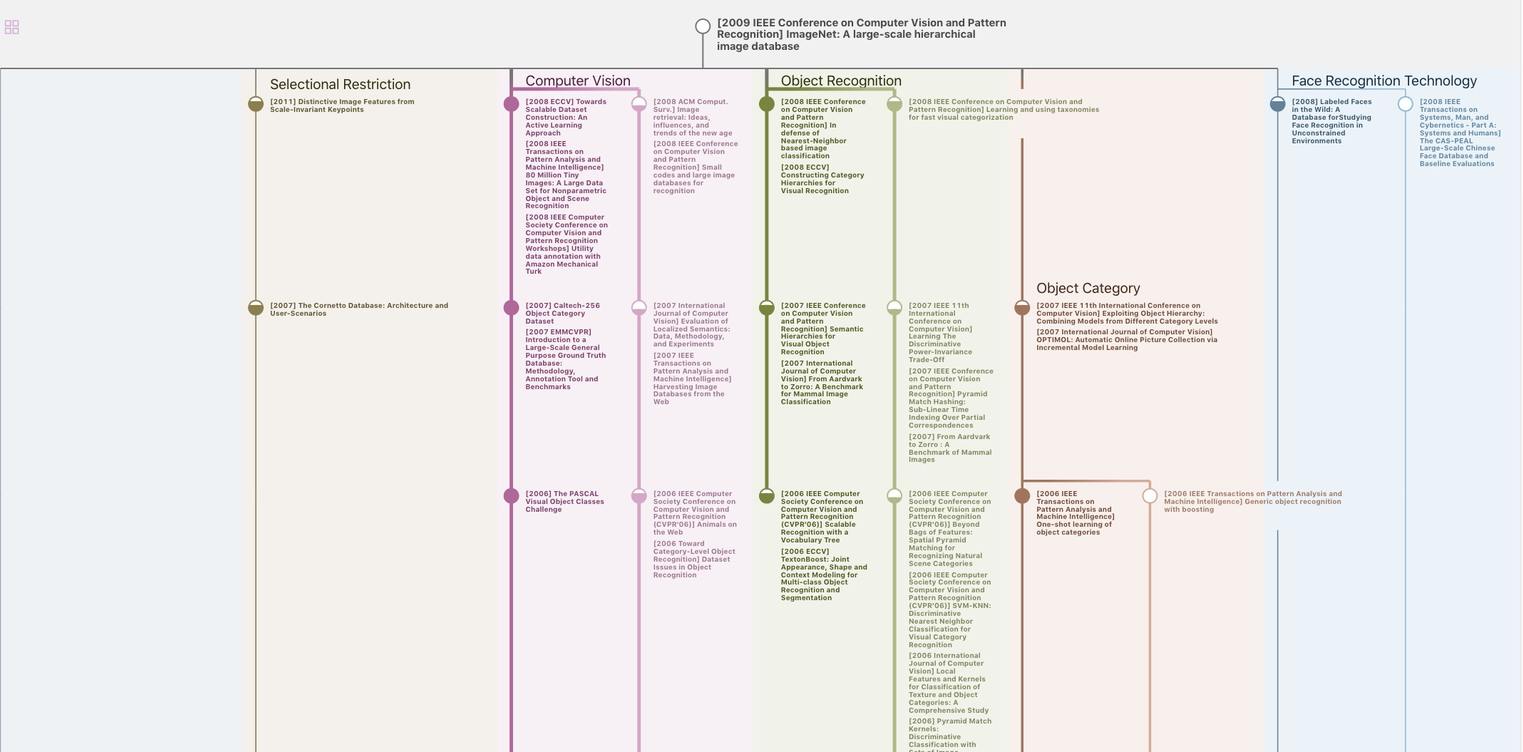
生成溯源树,研究论文发展脉络
Chat Paper
正在生成论文摘要