Learning Nighttime Semantic Segmentation the Hard Way
ACM Transactions on Multimedia Computing, Communications, and Applications(2023)
摘要
Nighttime semantic segmentation is an important but challenging research problem for autonomous driving. The major challenges lie in the small objects or regions from the under-/over-exposed areas or suffer from motion blur caused by the camera deployed on moving vehicles. To resolve this, we propose a novel hard-class-aware module that bridges the main network for full-class segmentation and the hard-class network for segmenting aforementioned hard-class objects. In specific, it exploits the shared focus of hard-class objects from the dual-stream network, enabling the contextual information flow to guide the model to concentrate on the pixels that are hard to classify. In the end, the estimated hard-class segmentation results will be utilized to infer the final results via an adaptive probabilistic fusion refinement scheme. Moreover, to overcome over-smoothing and noise caused by extreme exposures, our model is modulated by a carefully crafted pretext task of constructing an exposure-aware semantic gradient map, which guides the model to faithfully perceive the structural and semantic information of hard-class objects while mitigating the negative impact of noises and uneven exposures. In experiments, we demonstrate that our unique network design leads to superior segmentation performance over existing methods, featuring with the strong ability of perceiving hard-class objects under adverse condition.
更多查看译文
关键词
Nighttime semantic segmentation,dual-branch,hard class,pretext task,fusion refinement scheme
AI 理解论文
溯源树
样例
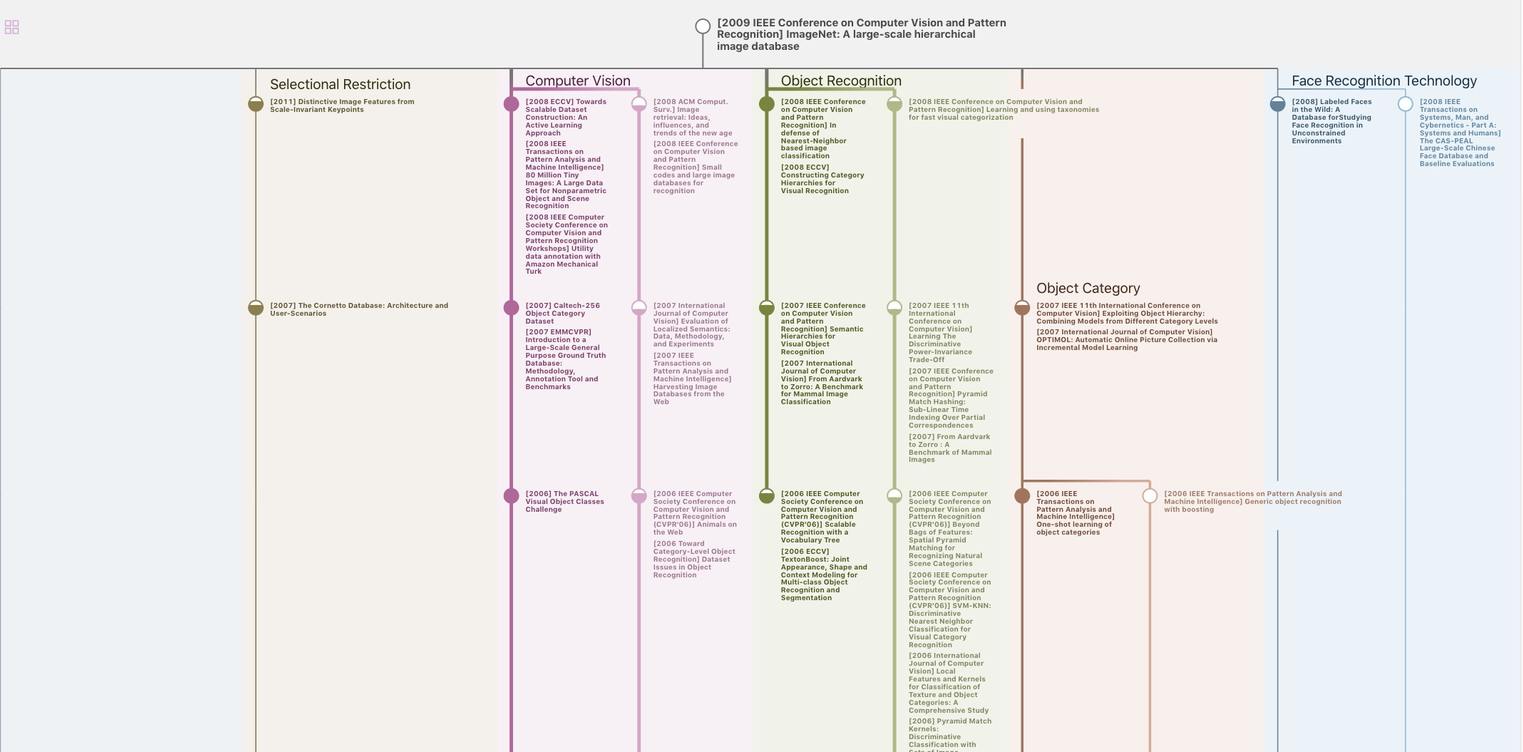
生成溯源树,研究论文发展脉络
Chat Paper
正在生成论文摘要