A deep reinforcement learning control strategy to improve the operating flexibility of CHP units under variable load conditions
Thermal Science and Engineering Progress(2024)
摘要
With the renewable energy generation into the power grid, the flexibility in operation and control becomes vital for combined heat and power (CHP) units under variable load conditions. This paper proposes a model-free deep reinforcement learning (DRL) strategy to enhance the operational flexibility and load regulation capability of CHP units in electricity-heat coordinated control. Firstly, the electricity-heat coordination problem is modeled as a Markov decision process (MDP), with the main steam pressure and electric power serving as the state space, the valve opening accounting for dynamic characteristics forming the action space, and the deviation of the main steam pressure being the reward function. Secondly, the training and solution for the electricity-heat coordinated control strategy of CHP units is accomplished by the deep deterministic policy gradient from demonstrations (DDPGfD) algorithm combining pre-training and offline training. Finally, a case study of a 300MW CHP unit under variable load conditions demonstrates that the proposed model-free DRL control strategy improves the power ramp rate of CHP units while maintaining the stability of the main steam pressure and heat supply, showcasing enhanced exploration capabilities of the agent.
更多查看译文
关键词
Combined heat and power,Deep reinforcement learning,Expert demonstrations,Operating flexibility,Steam extraction heating
AI 理解论文
溯源树
样例
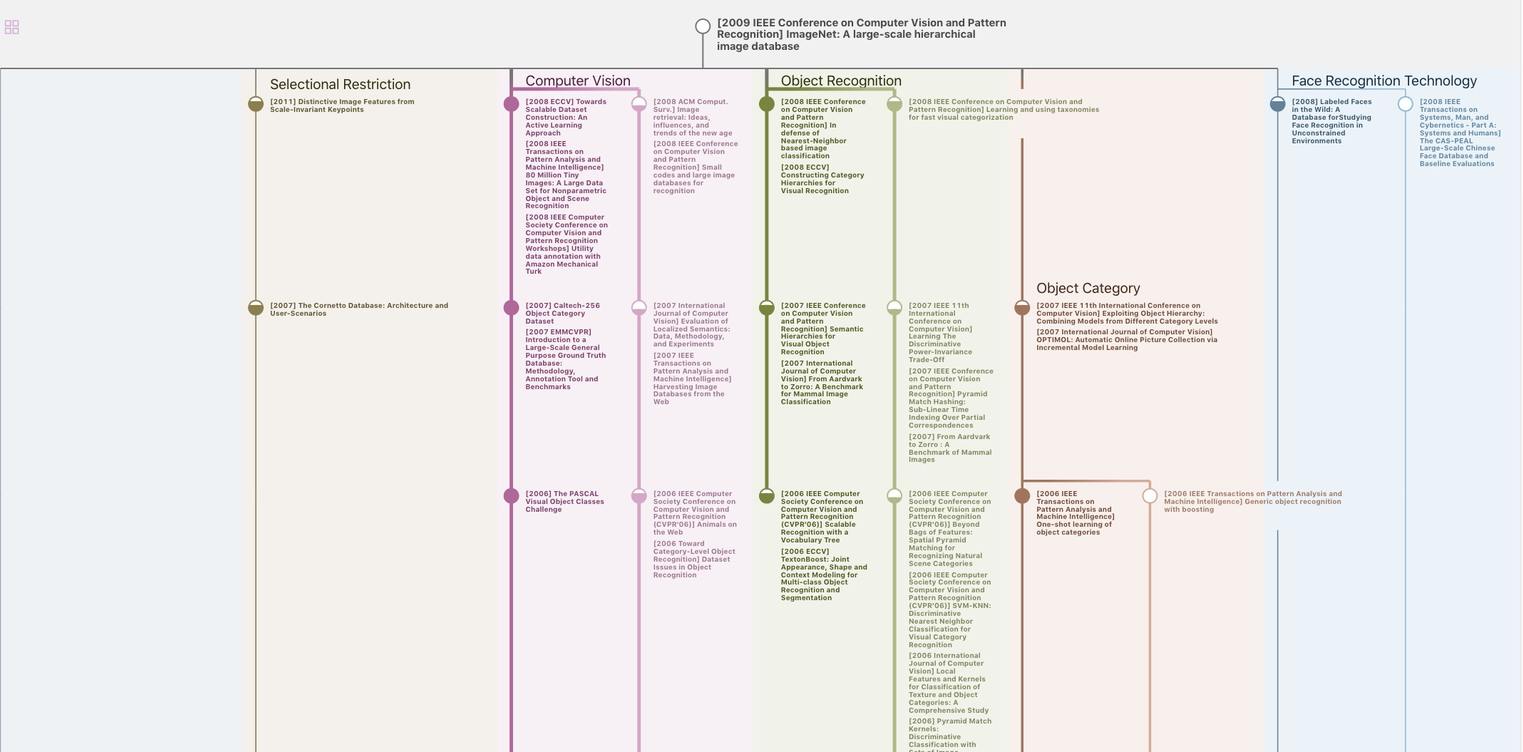
生成溯源树,研究论文发展脉络
Chat Paper
正在生成论文摘要