Decoupled domain-specific and domain-conditional representation learning for cross-domain recommendation
Information Processing & Management(2024)
摘要
Cross-domain recommendation (CDR) has become popular to alleviate the sparsity problem in target-domain recommendation by utilizing auxiliary domain knowledge. A basic assumption of CDR is that users have shared preferences across domains, but most existing CDR models do not distinguish between users’ unique preferences and shared preferences. We propose a new CDR model, called DRLCDR, which adopts a variational bipartite graph encoder to learn domain-specific representations and domain-shared representations, respectively. To make the domain-shared representation learned from different domains similar, the domain-specific representations learned from one domain is used as conditional information to guide the domain-shared representations (which is also called domain-conditional representation in our model) in another domain. In addition, a bridge function loss is adopted to further encourage the proximity of domain-conditional representations in the embedding space. Experiments on four public datasets show that DRLCDR outperforms strong baselines, including the recent CDR method using disentangled learning, with an average improvement of 3.32% and 3.01% for HR and NDCG, respectively.
更多查看译文
关键词
Cross-domain recommendation,Collaborative filtering,GCN,Disentangled representation learning
AI 理解论文
溯源树
样例
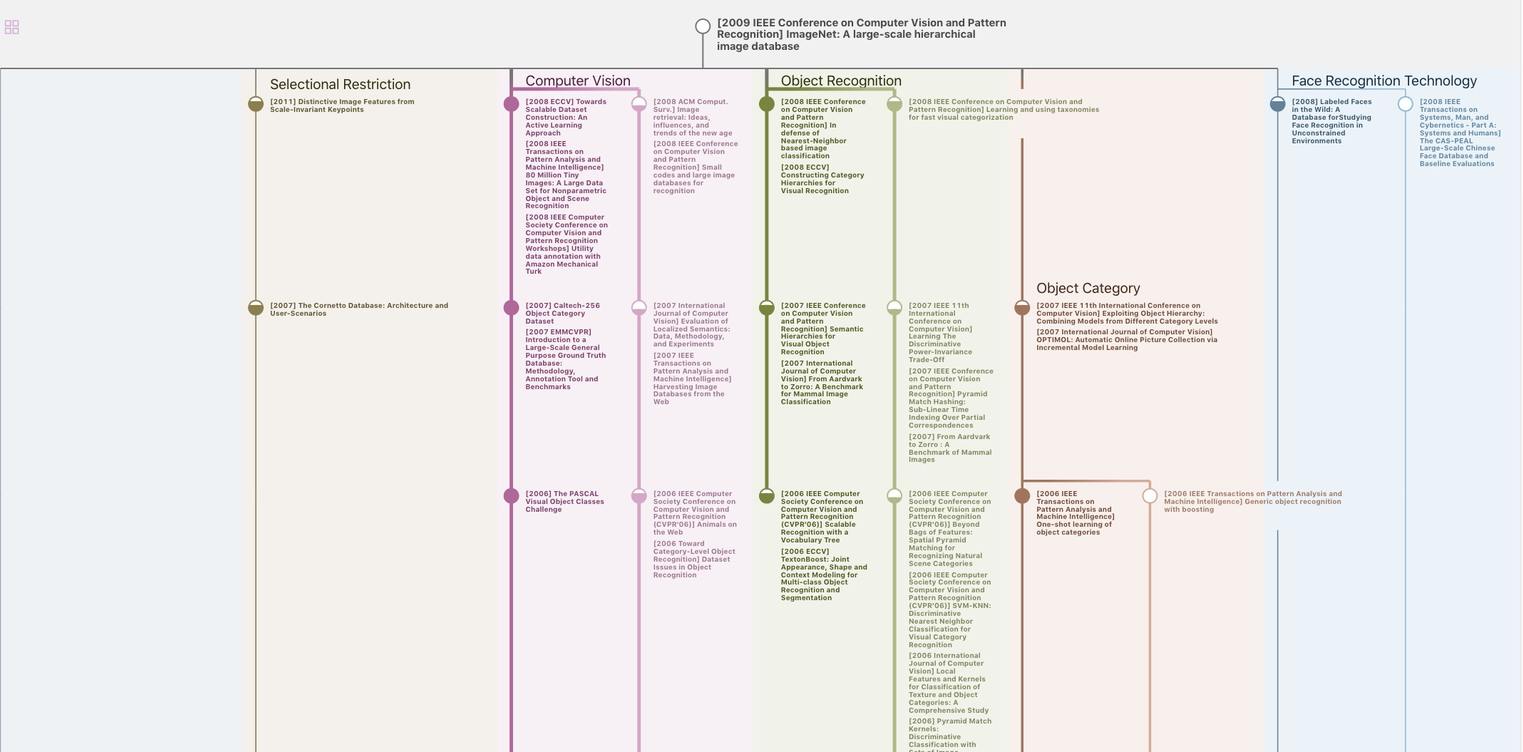
生成溯源树,研究论文发展脉络
Chat Paper
正在生成论文摘要