MixSegNet: Fusing multiple mixed-supervisory signals with multiple views of networks for mixed-supervised medical image segmentation
Engineering Applications of Artificial Intelligence(2024)
摘要
Deep learning has driven remarkable advancements in medical image segmentation. The requirement for comprehensive annotations, however, poses a significant challenge due to the labor-intensive and expensive nature of expert annotation. Addressing this challenge, we introduce a multiple mixed-supervisory signals learning (MSL) strategy, MixSegNet, that synergistically harnesses the benefits of Fully-Supervised (FSL), Weakly-Supervised (WSL), and Semi-Supervised Learning (SSL). This approach enables the utilization of various data-efficient annotations for network training, promoting efficient medical image segmentation within realistic clinical scenarios. MixSegNet concurrently trains networks with a combination of limited dense labels, a larger proportion of cost-efficient sparse labels, and unlabeled data. The networks utilized in this system comprise Vision Transformer (ViT) and Convolutional Neural Networks (CNN), which work together via an effective strategy including network self-ensembling and label dynamic-ensembling. This strategy adeptly handles the training challenges arising from datasets with limited or absent supervisory signals. We validated MixSegNet on a public Magnetic Resonance Imaging (MRI) cardiac segmentation benchmark dataset. It demonstrated superior performance compared to 21 other SSL or WSL baseline methods under similar labeling-cost conditions, as supported by comprehensive evaluation metrics, and slightly outperform classical FSL methods. The code for MixSegNet, all baseline methods, and the data pre-processing techniques with the datasets for different annotation situations are available at https://github.com/ziyangwang007/MixSegNet.
更多查看译文
关键词
Vision transformer,Medical image segmentation,Mixed-supervised learning
AI 理解论文
溯源树
样例
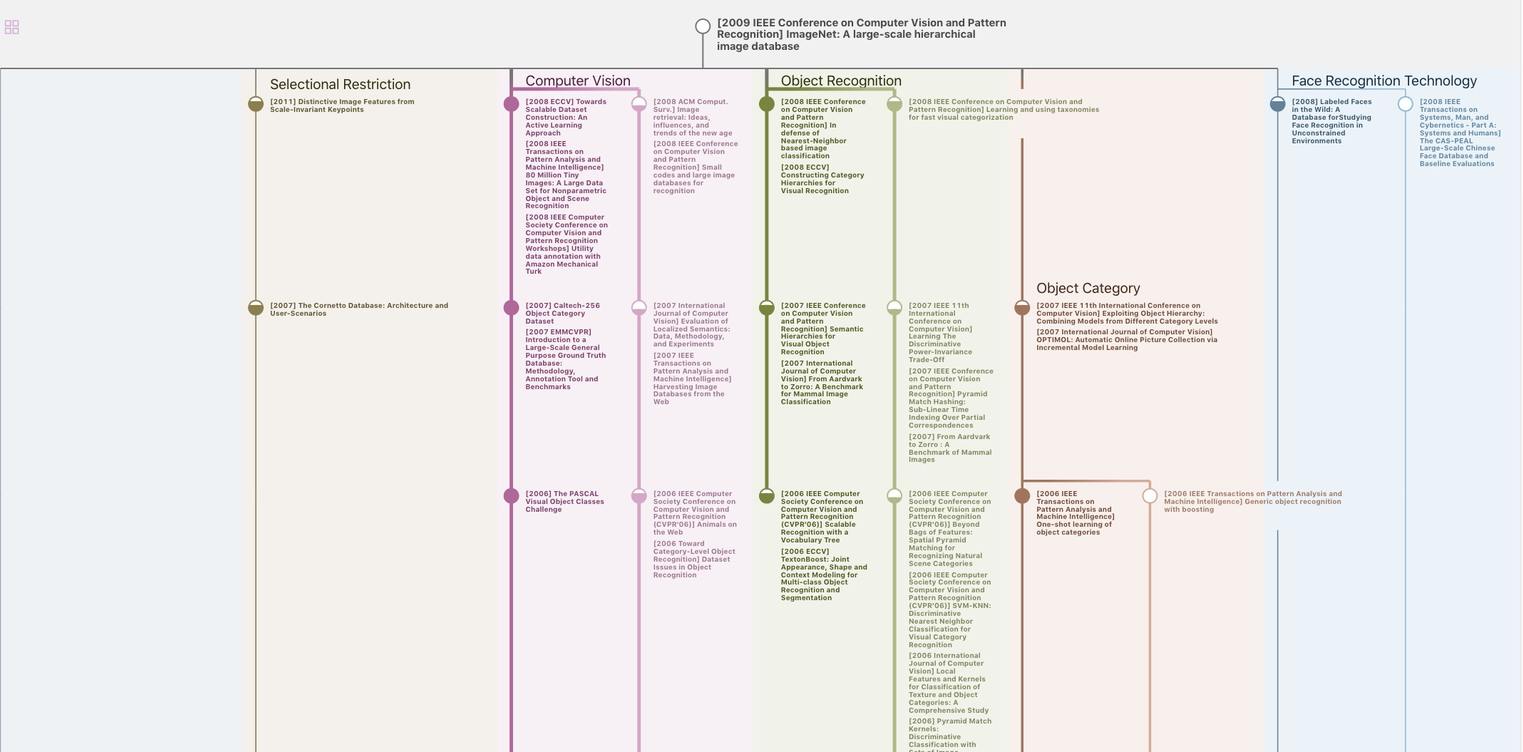
生成溯源树,研究论文发展脉络
Chat Paper
正在生成论文摘要