VisTFC: Vision-guided target-side future context learning for neural machine translation
Expert Systems with Applications(2024)
摘要
Visual features encompass visual information extracted from images or videos, serving as supplementary input to enhance the efficacy of neural machine translation (NMT) systems. By seamlessly integrating these visual features into the translation process, NMT models can tap into the expansive visual context, thereby making more precise predictions during translation. Nonetheless, limited efforts have been directed towards exploring the potential of learning target-side future context using visual features to augment NMT performance. To bridge this gap, this paper introduces the Vision-guided Target-side Future Context (VisTFC) learning framework for NMT. Our core objective is to refine translation quality by effectively harnessing and incorporating target-side future contextual insights derived from the visual modality. The VisTFC framework consists of three pivotal components. Firstly, a graph-based multimodal encoder–decoder is established, constructing a bipartite vision-source/target graph. This enables the acquisition of vision-fused textual representations, synthesizing both linguistic and visual attributes to enhance translation accuracy. Secondly, a target-side future context predictor, incorporating a dynamic routing mechanism, infers future context by synergizing visual information. This empowers the model to anticipate and assimilate contextual cues, ensuring more coherent and contextually coherent translations. Thirdly, a sigmoid update gate is introduced, facilitating controlled integration of predicted future context into the decoding process, enabling the decoder to flexibly adapt and utilize the inferred context. Moreover, the VisTFC framework is fortified with additional loss functions that enforce source/target-vision consistency, reinforcing its robustness and effectiveness. Our results demonstrate that our model can achieve substantial improvements, reaching up to 1.0/0.9/1.1 BLEU points beyond the performance of the most robust baselines. This superior performance is evident on both the Test2016 and Test2017 datasets, as well as on the MSCOCO test sets for English-French translation tasks. Furthermore, our experiments underscore the efficacy of VisTFC, even in scenarios with limited resources, such as the English-Hausa language pair. Here, the model achieves notable enhancements, with improvements of up to 1.8/1.9 BLEU points compared to the strongest text-only NMT models on E-Test and C-Test test sets. These findings provide strong evidence supporting our model’s ability to effectively harness target-side future contextual information from the visual modality, resulting in substantial enhancements in machine translation quality. Further analysis and visualization suggest that VisTFC is capable of learning target-side future context from visual signals for better translation. We also have open-sourced our work in Github.11https://github.com/nlpdl/VsiTFC.
更多查看译文
关键词
Machine translation,Vision and language,Transformer,Multimodal consistency
AI 理解论文
溯源树
样例
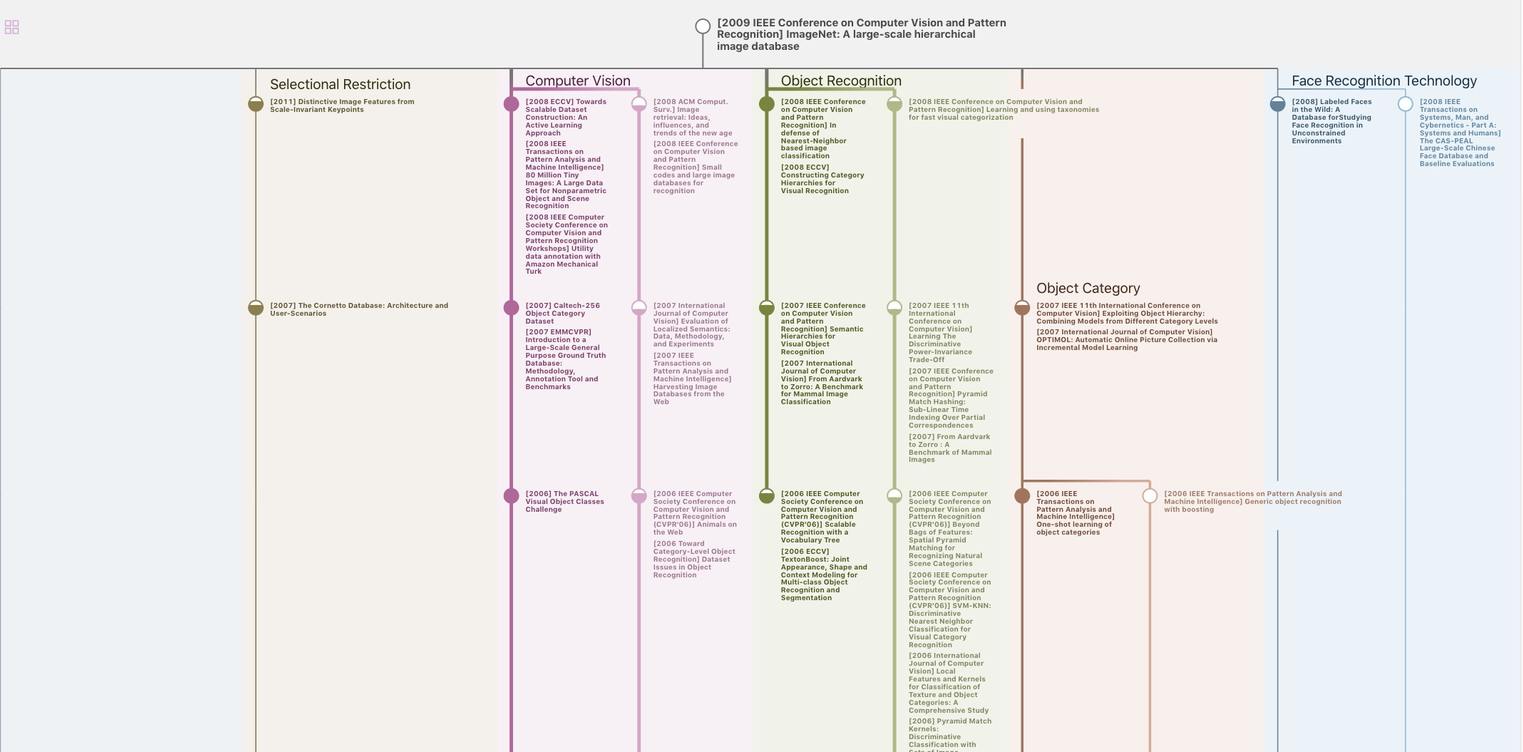
生成溯源树,研究论文发展脉络
Chat Paper
正在生成论文摘要