A novel Elman neural network based on Gaussian kernel and improved SOA and its applications
EXPERT SYSTEMS WITH APPLICATIONS(2024)
摘要
To address challenges encountered in traditional Elman neural networks (ENNs), such as low convergence accuracy, difficulties in hyperparameter selection, and issues with gradient disappearance, a new ENN based on the Gaussian kernel and an improved seagull optimization algorithm (SOA) named GSENN is proposed. First, the principle of the ENN is introduced, and the effects of the network structure, parameters, and gradient descent algorithm on its output are analyzed. Building upon the analysis results, an input layer for the ENN using the Gaussian kernel is designed. The outstanding local feature extraction capability of the Gaussian kernel is used to enhance convergence accuracy, and the predicted trends are more in line with real data. In addition, we propose an SOA incorporating a nonlinear factor to optimize the weights and thresholds of the ENN. This approach aims to resolve the challenges in hyperparameter selection and problems of gradient vanishing in the ENN. Seven commonly used neural network structures and the latest improved techniques were chosen for comparative experiments across different datasets. The experimental results show that GSENN achieves a mean relative error rate of 4.286 %. The MSE results indicate a 45.600 % improvement over the original ENN and a 37.142 % improvement over BP neural networks. The experimental results demonstrate that GSENN exhibits efficient forecasting capability and offers reliable and effective forecasting for the relevant departments.
更多查看译文
关键词
Elman neural network,Gaussian kernel,Seagull optimization algorithm,Power load forecasting
AI 理解论文
溯源树
样例
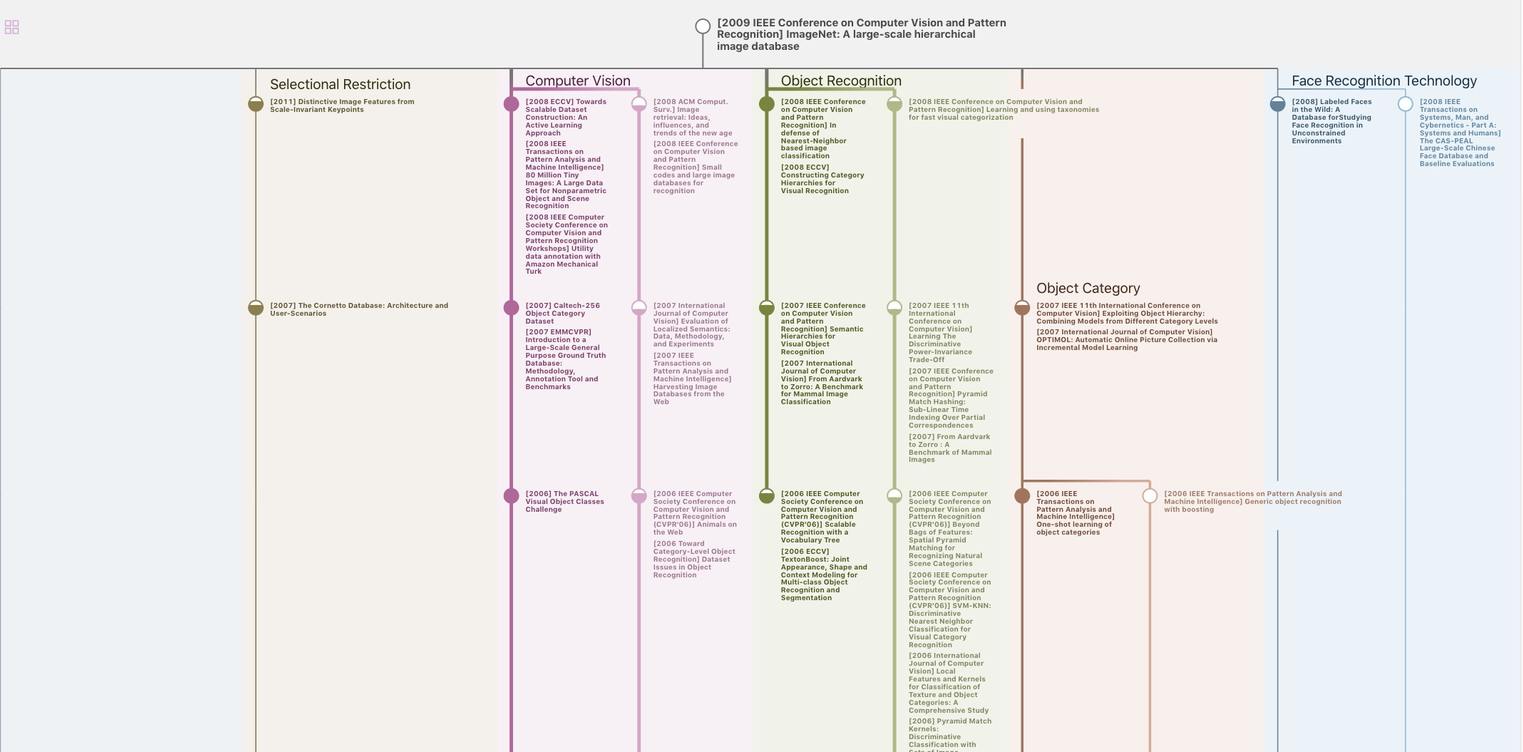
生成溯源树,研究论文发展脉络
Chat Paper
正在生成论文摘要