Trans-Net: A transferable pretrained neural networks based on temporal domain decomposition for solving partial differential equations
Computer Physics Communications(2024)
摘要
Physics-Informed Neural Networks (PINNs) has provided a novel direction for solving partial differential equations (PDEs) and has achieved significant advancements in the field of scientific computing. PINNs effectively incorporate the physical constraints of equations into the loss function, enabling neural networks to learn and approximate the behavior of physical systems by optimizing the loss function. According to the existing research, we propose a transferable pretrained neural networks framework based on temporal domain decomposition to solve partial differential equations. Specifically, we divide the domain into multiple subdomains according to time, each of which is solved using an individual neural network. Subsequently, the trained subnetwork and the predicted values at the common boundary are used as the pretrained model and initial values for the next subdomain, respectively. This not only improves the prediction accuracy of the subnetwork, but also reduces the training time. Finally, we present a series of classical numerical experiments including one-dimensional, two-dimensional, and three-dimensional partial differential equations. The experimental results indicate that the proposed method outperforms existing approaches in terms of accuracy and efficiency. Relevant data and code can be accessed on GitHub via https://github.com/karry-Zhang/Trans-Net.
更多查看译文
关键词
Partial differential equations,Deep neural network,Temporal domain decomposition,Pretrained model
AI 理解论文
溯源树
样例
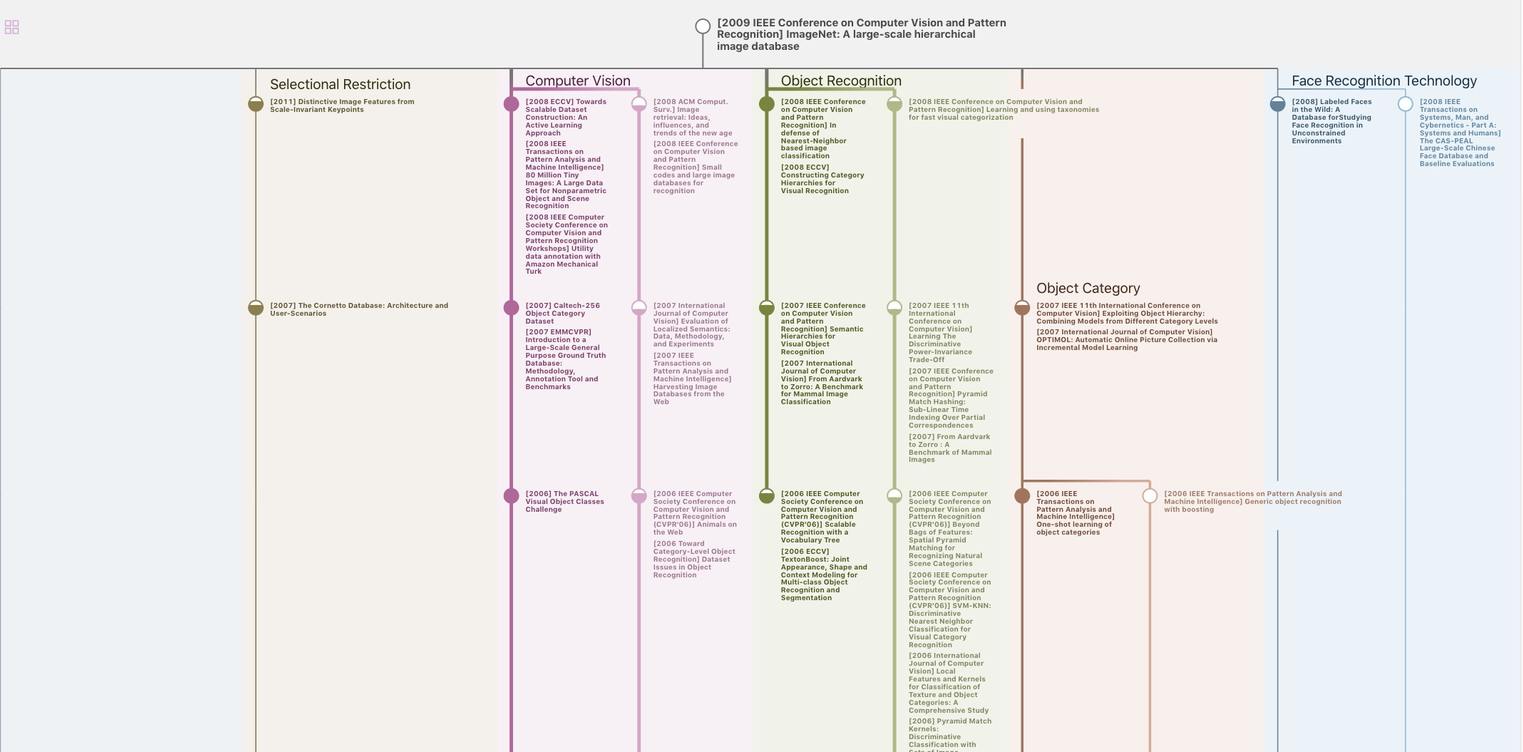
生成溯源树,研究论文发展脉络
Chat Paper
正在生成论文摘要