SCS-SLSP: Hard uncertain pixels mining and utilization for semi-supervised cardiac image segmentation using subjective logic theory and subset prototype generation
BIOMEDICAL SIGNAL PROCESSING AND CONTROL(2024)
摘要
Semi -supervised learning methods have achieved great success in cardiac image analysis. This paper presents an effective semi -supervised cardiac image segmentation framework (SCS-SLSP) that utilizes subjective logic (SL) theory and the subset prototype generation (SPG) module. Specifically, based on the student-teacher model, SL theory generates soft labels with uncertainty for unlabeled data, distinguishing in -distribution pixels and out -of -distribution (uncertain) pixels located near class feature boundaries. The SPG module generates subset prototypes locating the feature space region of uncertain pixels. Soft multi -labels, computed using both subset and single -class prototypes, determine the relative position of uncertain pixels' feature vectors in the feature space. Leveraging soft multi -labels, our developed hard uncertain pixel mining (HM) module distinguishes easy uncertain samples, accepting their refined labels as trusted pseudo -labels. For hard uncertain samples, despite unreliable refined labels, some certainty to non -associated classes is observed. Thus, their soft multi -labels are stored in a memory bank as negative samples for certain classes. Then, contrastive learning is applied to all data to attract the positive sample pairs and repulse the negative sample pairs. We evaluated the proposed SCS-SLSP against several state-of-the-art semi -supervised approaches on three public datasets, and the results demonstrate the superiority of our network. When trained with 10% labeled data on the ACDC dataset, compared to the best results in all compared methods, SCS-SLSP improved the Dice by 0.41%, increased the JSC by 1.02%, reduced the HD95 by 56.82%, and decreased the ASD by 49.63%. The code for this work is available at https://github.com/helathegreat/SCS-SLSP.
更多查看译文
关键词
Semi-supervised learning,Cardiac image segmentation,Subjective logic,Soft pseudo-label,Uncertainty
AI 理解论文
溯源树
样例
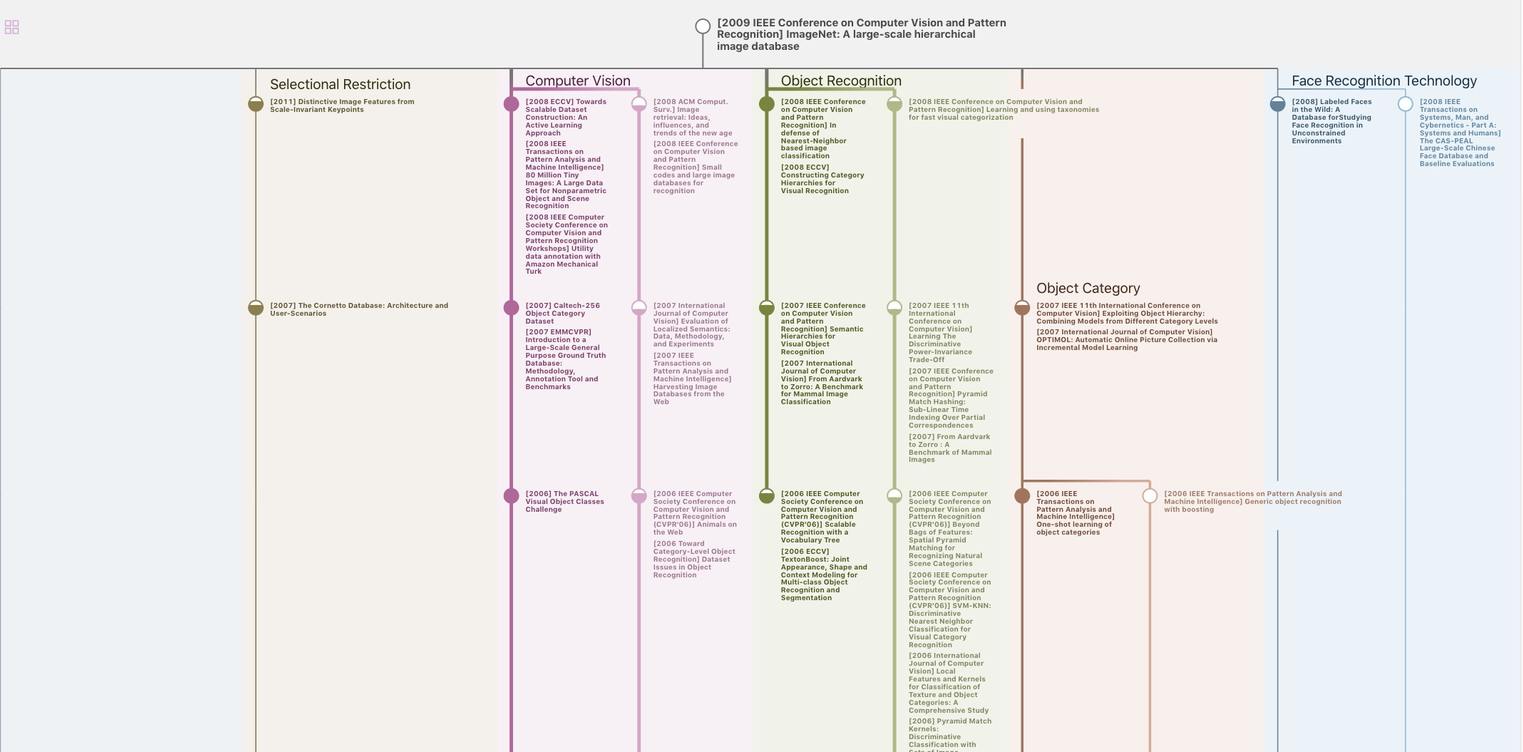
生成溯源树,研究论文发展脉络
Chat Paper
正在生成论文摘要