Graph-based multi-level feature fusion network for diabetic retinopathy grading using ultra-wide-field images
Biomedical Signal Processing and Control(2024)
摘要
Diabetic Retinopathy (DR) grading plays an important role for early intervention in managing the disease. However, conventional grading methods predominantly rely on traditional color fundus photography, which may not fully capture crucial pathological information. In contrast, Ultra-wide-field (UWF) represents a novel non-contact and non-invasive modality, capable of generating images with an unprecedented field of view, ranging from 180∘ to 200∘. This offers distinct advantages over conventional color fundus photography for DR grading. Furthermore, given the subtle distinctions in imaging characteristics among adjacent levels of DR, the grading task becomes exceptionally challenging. Therefore, we propose a new multi-level feature fusion network based on the graph convolution to accomplish the DR grading task using UWF images. Specifically, we first use the progressive multi-level constraint module to iteratively refine features by imposing constraints on model features at different levels. This process serves to minimize the inter-individual distances within the intra-class feature space, ultimately reducing the overlap of feature spaces between adjacent classes. Subsequently, since features at different network levels exhibit distinct semantics and distributions, conventional aggregation techniques, such as splicing and summing, may introduce redundant information and noise interference. To address this issue, we introduce a graph-based feature fusion module, capable of capturing spatial relationships and effectively fusing multi-layered features. Experimental results evaluated on an in-house UWF dataset with 1234 images and a publicly available fundus dataset with 3662 images illustrate that the proposed approach outperforms the state-of-the-art methods and shows promise for DR grading, with a respective accuracy of 0.81 and 0.84.
更多查看译文
关键词
Diabetic retinopathy grading,UWF,Graph-based feature fusion,Multi-level,Retina
AI 理解论文
溯源树
样例
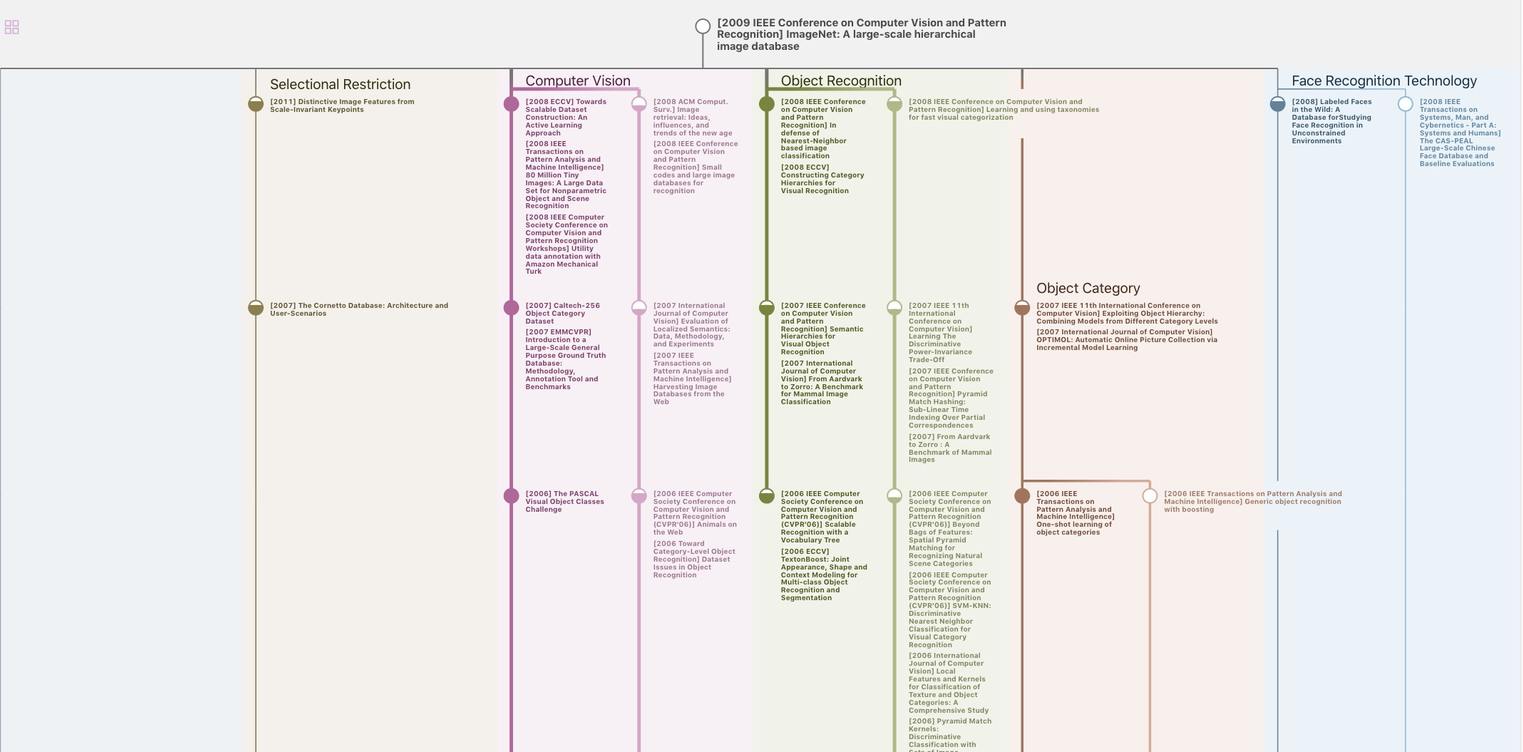
生成溯源树,研究论文发展脉络
Chat Paper
正在生成论文摘要