Multi-strategy active learning for power quality disturbance identification
APPLIED SOFT COMPUTING(2024)
摘要
As clean energy facilities are increasingly integrated into power distribution networks, the incidence of multiple power quality disturbances (MPQDs) is on the rise. Currently, most MPQDs classification models rely on supervised learning, which demands a substantial amount of labeled data. However, obtaining labeled MPQDs data from the real world is often a time-consuming and labor-intensive process, requiring annotation by experienced engineers, particularly for events involving MPQDs. To tackle this challenge, we introduce an innovative active learning (AL) approach known as Adaptive Weighting Multi -Strategy AL (AWMSAL) for MPQDs classification. In our framework, AWMSAL simultaneously considers multiple strategies using rank aggregation to select MPQDs data that is both full -information and highly representative for labeling. In addition, our method employs a unique weight calculation function to dynamically adjust weights based on the differences in data values observed with various strategies during the AL process. To enhance the classifier performance, we incorporate the Laplacian manifold regularizer into the Extreme Learning Machine in both supervised and unsupervised settings. We compared the performance of the AWMSAL method against several state-of-the-art active learning algorithms using synthetic and hardware -generated data sets. The results demonstrated that AWMSAL achieved recognition rates exceeding 99% in a shorter number of iterations. When the same termination condition was met, AWMSAL outperformed other active learning algorithms by 2.45% to 18.33% in terms of accuracy.
更多查看译文
关键词
Multi-strategy active learning,Extreme learning machine,Power quality disturbance,Rank aggregation
AI 理解论文
溯源树
样例
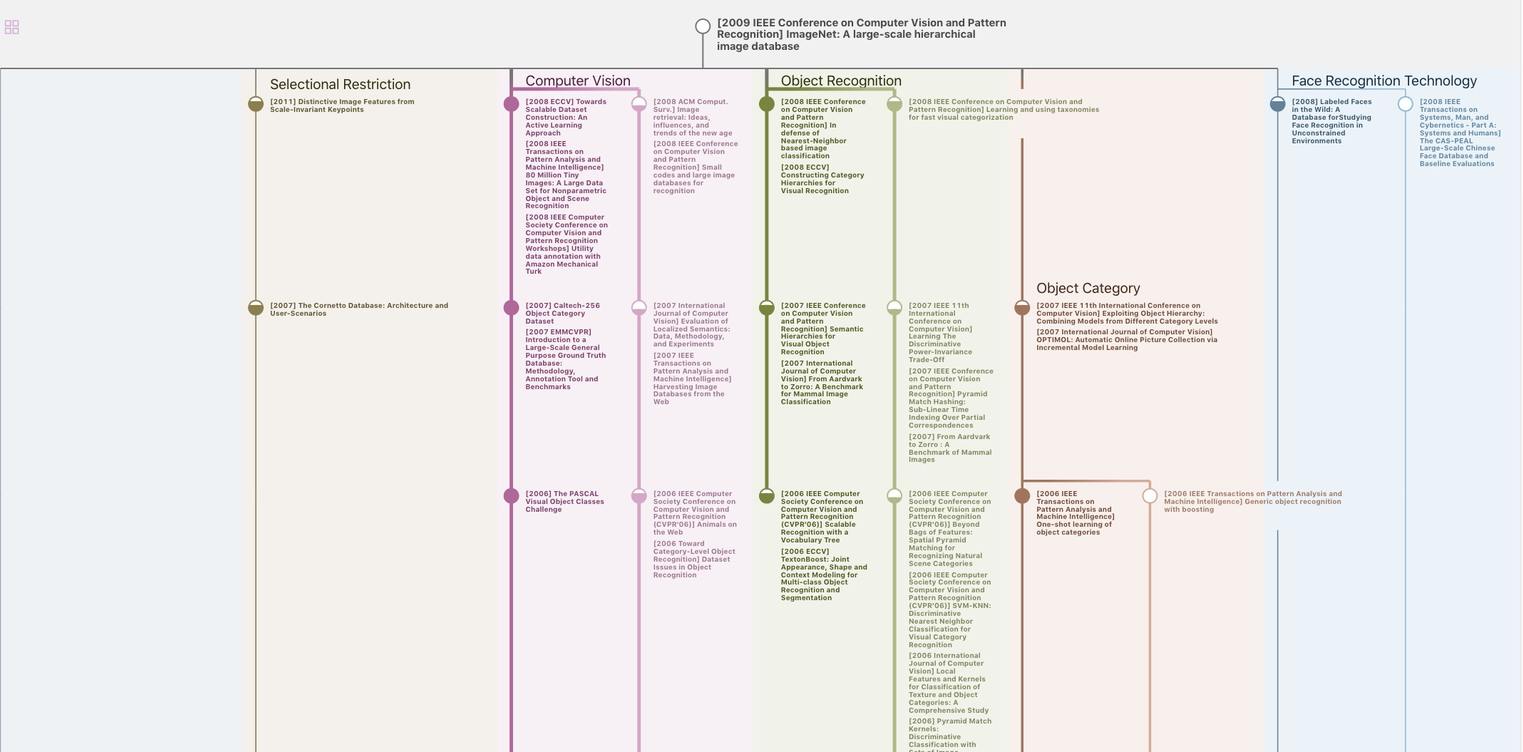
生成溯源树,研究论文发展脉络
Chat Paper
正在生成论文摘要