The G-invariant graph Laplacian Part I: Convergence rate and eigendecomposition
Applied and Computational Harmonic Analysis(2024)
摘要
Graph Laplacian based algorithms for data lying on a manifold have been proven effective for tasks such as dimensionality reduction, clustering, and denoising. In this work, we consider data sets whose data points lie on a manifold that is closed under the action of a known unitary matrix Lie group G. We propose to construct the graph Laplacian by incorporating the distances between all the pairs of points generated by the action of G on the data set. We deem the latter construction the “G-invariant Graph Laplacian” (G-GL). We show that the G-GL converges to the Laplace-Beltrami operator on the data manifold, while enjoying a significantly improved convergence rate compared to the standard graph Laplacian which only utilizes the distances between the points in the given data set. Furthermore, we show that the G-GL admits a set of eigenfunctions that have the form of certain products between the group elements and eigenvectors of certain matrices, which can be estimated from the data efficiently using FFT-type algorithms. We demonstrate our construction and its advantages on the problem of filtering data on a noisy manifold closed under the action of the special unitary group SU(2).
更多查看译文
关键词
Manifold learning,Group invariance,Graph Laplacian,Manifold denoising
AI 理解论文
溯源树
样例
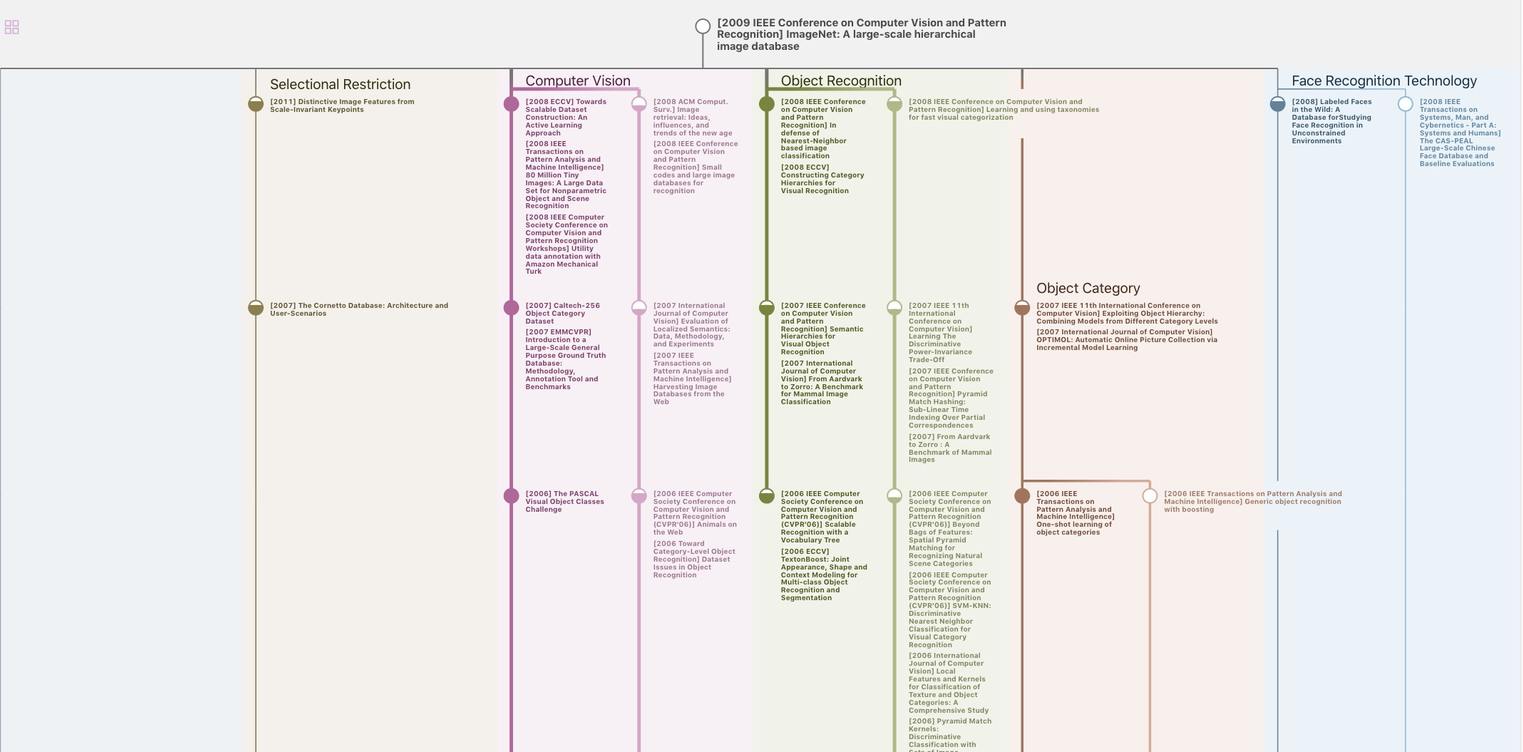
生成溯源树,研究论文发展脉络
Chat Paper
正在生成论文摘要